Determination of mammographic breast density using a deep convolutional neural network
- PMID: 30209957
- PMCID: PMC6435091
- DOI: 10.1259/bjr.20180691
Determination of mammographic breast density using a deep convolutional neural network
Abstract
Objective: High breast density is a risk factor for breast cancer. The aim of this study was to develop a deep convolutional neural network (dCNN) for the automatic classification of breast density based on the mammographic appearance of the tissue according to the American College of Radiology Breast Imaging Reporting and Data System (ACR BI-RADS) Atlas.
Methods: In this study, 20,578 mammography single views from 5221 different patients (58.3 ± 11.5 years) were downloaded from the picture archiving and communications system of our institution and automatically sorted according to the ACR density (a-d) provided by the corresponding radiological reports. A dCNN with 11 convolutional layers and 3 fully connected layers was trained and validated on an augmented dataset. The model was finally tested on two different datasets against: i) the radiological reports and ii) the consensus decision of two human readers. None of the test datasets was part of the dataset used for the training and validation of the algorithm.
Results: The optimal number of epochs was 91 for medio-lateral oblique (MLO) projections and 94 for cranio-caudal projections (CC), respectively. Accuracy for MLO projections obtained on the validation dataset was 90.9% (CC: 90.1%). Tested on the first test dataset of mammographies (850 MLO and 880 CC), the algorithm showed an accordance with the corresponding radiological reports of 71.7% for MLO and of 71.0% for CC. The agreement with the radiological reports improved in the differentiation between dense and fatty breast for both projections (MLO = 88.6% and CC = 89.9%). In the second test dataset of 200 mammographies, a good accordance was found between the consensus decision of the two readers on both, the MLO-model (92.2%) and the right craniocaudal-model (87.4%). In the differentiation between fatty (ACR A/B) and dense breasts (ACR C/D), the agreement reached 99% for the MLO and 96% for the CC projections, respectively.
Conclusions: The dCNN allows for accurate classification of breast density based on the ACR BI-RADS system. The proposed technique may allow accurate, standardized, and observer independent breast density evaluation of mammographies.
Advances in knowledge: Standardized classification of mammographies by a dCNN could lead to a reduction of falsely classified breast densities, thereby allowing for a more accurate breast cancer risk assessment for the individual patient and a more reliable decision, whether additional ultrasound is recommended.
Figures
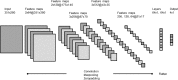
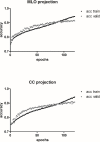
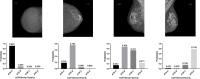
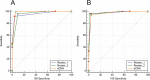
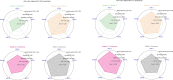
Similar articles
-
Diagnostic accuracy of automated ACR BI-RADS breast density classification using deep convolutional neural networks.Eur Radiol. 2023 Jul;33(7):4589-4596. doi: 10.1007/s00330-023-09474-7. Epub 2023 Mar 1. Eur Radiol. 2023. PMID: 36856841 Free PMC article.
-
Automated mammographic breast density estimation using a fully convolutional network.Med Phys. 2018 Mar;45(3):1178-1190. doi: 10.1002/mp.12763. Epub 2018 Feb 19. Med Phys. 2018. PMID: 29363774 Free PMC article.
-
Understanding Clinical Mammographic Breast Density Assessment: a Deep Learning Perspective.J Digit Imaging. 2018 Aug;31(4):387-392. doi: 10.1007/s10278-017-0022-2. J Digit Imaging. 2018. PMID: 28932980 Free PMC article.
-
Supplemental Screening for Breast Cancer in Women With Dense Breasts: A Systematic Review for the U.S. Preventive Service Task Force [Internet].Rockville (MD): Agency for Healthcare Research and Quality (US); 2016 Jan. Report No.: 14-05201-EF-3. Rockville (MD): Agency for Healthcare Research and Quality (US); 2016 Jan. Report No.: 14-05201-EF-3. PMID: 26866210 Free Books & Documents. Review.
-
Learning multi-frequency features in convolutional network for mammography classification.Med Biol Eng Comput. 2022 Jul;60(7):2051-2062. doi: 10.1007/s11517-022-02582-4. Epub 2022 May 13. Med Biol Eng Comput. 2022. PMID: 35553003 Review.
Cited by
-
Assessment of the Breast Density Prevalence in Swiss Women with a Deep Convolutional Neural Network: A Cross-Sectional Study.Diagnostics (Basel). 2024 Oct 3;14(19):2212. doi: 10.3390/diagnostics14192212. Diagnostics (Basel). 2024. PMID: 39410616 Free PMC article.
-
CAD and AI for breast cancer-recent development and challenges.Br J Radiol. 2020 Apr;93(1108):20190580. doi: 10.1259/bjr.20190580. Epub 2019 Dec 16. Br J Radiol. 2020. PMID: 31742424 Free PMC article. Review.
-
Research on Online Social Network Information Leakage-Tracking Algorithm Based on Deep Learning.Comput Intell Neurosci. 2022 Jun 28;2022:1926794. doi: 10.1155/2022/1926794. eCollection 2022. Comput Intell Neurosci. 2022. PMID: 35800694 Free PMC article.
-
Digital breast tomosynthesis-based peritumoral radiomics approaches in the differentiation of benign and malignant breast lesions.Diagn Interv Radiol. 2022 May;28(3):217-225. doi: 10.5152/dir.2022.20664. Diagn Interv Radiol. 2022. PMID: 35748203 Free PMC article.
-
Comparative Study on Local Binary Patterns for Mammographic Density and Risk Scoring.J Imaging. 2019 Feb 1;5(2):24. doi: 10.3390/jimaging5020024. J Imaging. 2019. PMID: 34460472 Free PMC article.
References
MeSH terms
LinkOut - more resources
Full Text Sources
Other Literature Sources
Medical