A novel method of using Deep Belief Networks and genetic perturbation data to search for yeast signaling pathways
- PMID: 30208101
- PMCID: PMC6135403
- DOI: 10.1371/journal.pone.0203871
A novel method of using Deep Belief Networks and genetic perturbation data to search for yeast signaling pathways
Abstract
Perturbing a signaling system with a serial of single gene deletions and then observing corresponding expression changes in model organisms, such as yeast, is an important and widely used experimental technique for studying signaling pathways. People have developed different computational methods to analyze the perturbation data from gene deletion experiments for exploring the signaling pathways. The most popular methods/techniques include K-means clustering and hierarchical clustering techniques, or combining the expression data with knowledge, such as protein-protein interactions (PPIs) or gene ontology (GO), to search for new pathways. However, these methods neither consider nor fully utilize the intrinsic relation between the perturbation of a pathway and expression changes of genes regulated by the pathway, which served as the main motivation for developing a new computational method in this study. In our new model, we first find gene transcriptomic modules such that genes in each module are highly likely to be regulated by a common signal. We then use the expression status of those modules as readouts of pathway perturbations to search for up-stream pathways. Systematic evaluation, such as through gene ontology enrichment analysis, has provided evidence that genes in each transcriptomic module are highly likely to be regulated by a common signal. The PPI density analysis and literature search revealed that our new perturbation modules are functionally coherent. For example, the literature search revealed that 9 genes in one of our perturbation module are related to cell cycle and all 10 genes in another perturbation module are related by DNA damage, with much evidence from the literature coming from in vitro or/and in vivo verifications. Hence, utilizing the intrinsic relation between the perturbation of a pathway and the expression changes of genes regulated by the pathway is a useful method of searching for signaling pathways using genetic perturbation data. This model would also be suitable for analyzing drug experiment data, such as the CMap data, for finding drugs that perturb the same pathways.
Conflict of interest statement
The authors have declared that no competing interests exist.
Figures
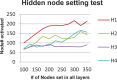
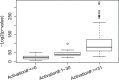
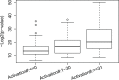
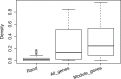
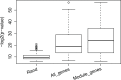
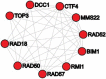
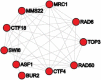
Similar articles
-
Construction of gene networks with hybrid approach from expression profile and gene ontology.IEEE Trans Inf Technol Biomed. 2010 Jan;14(1):107-18. doi: 10.1109/TITB.2009.2033056. Epub 2009 Sep 29. IEEE Trans Inf Technol Biomed. 2010. PMID: 19789116
-
Novel symmetry-based gene-gene dissimilarity measures utilizing Gene Ontology: Application in gene clustering.Gene. 2018 Dec 30;679:341-351. doi: 10.1016/j.gene.2018.08.062. Epub 2018 Sep 2. Gene. 2018. PMID: 30184472
-
Bioinformatics analysis to identify key genes and pathways influencing synovial inflammation in osteoarthritis.Mol Med Rep. 2018 Dec;18(6):5594-5602. doi: 10.3892/mmr.2018.9575. Epub 2018 Oct 23. Mol Med Rep. 2018. PMID: 30365099 Free PMC article.
-
Differential Co-Expression Analyses Allow the Identification of Critical Signalling Pathways Altered during Tumour Transformation and Progression.Int J Mol Sci. 2020 Dec 12;21(24):9461. doi: 10.3390/ijms21249461. Int J Mol Sci. 2020. PMID: 33322692 Free PMC article. Review.
-
A review of connectivity map and computational approaches in pharmacogenomics.Brief Bioinform. 2018 May 1;19(3):506-523. doi: 10.1093/bib/bbw112. Brief Bioinform. 2018. PMID: 28069634 Free PMC article. Review.
Cited by
-
Revealing the Impact of Genomic Alterations on Cancer Cell Signaling with an Interpretable Deep Learning Model.Cancers (Basel). 2023 Jul 29;15(15):3857. doi: 10.3390/cancers15153857. Cancers (Basel). 2023. PMID: 37568673 Free PMC article.
-
Omics Data and Data Representations for Deep Learning-Based Predictive Modeling.Int J Mol Sci. 2022 Oct 14;23(20):12272. doi: 10.3390/ijms232012272. Int J Mol Sci. 2022. PMID: 36293133 Free PMC article. Review.
References
-
- Hughes TR, Marton MJ, Jones AR, Roberts CJ, Stoughton R, Armour CD, et al. Functional discovery via a compendium of expression profiles. Cell. 2000;102(1):109–26. . - PubMed
Publication types
MeSH terms
Grants and funding
LinkOut - more resources
Full Text Sources
Other Literature Sources
Molecular Biology Databases