A massively parallel reporter assay reveals context-dependent activity of homeodomain binding sites in vivo
- PMID: 30158147
- PMCID: PMC6169884
- DOI: 10.1101/gr.231886.117
A massively parallel reporter assay reveals context-dependent activity of homeodomain binding sites in vivo
Abstract
Cone-rod homeobox (CRX) is a paired-like homeodomain transcription factor (TF) and a master regulator of photoreceptor development in vertebrates. The in vitro DNA binding preferences of CRX have been described in detail, but the degree to which in vitro binding affinity is correlated with in vivo enhancer activity is not known. In addition, paired-class homeodomain TFs can bind DNA cooperatively as both homodimers and heterodimers at inverted TAAT half-sites separated by 2 or 3 nucleotides. This dimeric configuration is thought to mediate target specificity, but whether monomeric and dimeric sites encode distinct levels of activity is not known. Here, we used a massively parallel reporter assay to determine how local sequence context shapes the regulatory activity of CRX binding sites in mouse photoreceptors. We assayed inactivating mutations in more than 1700 TF binding sites and found that dimeric CRX binding sites act as stronger enhancers than monomeric CRX binding sites. Furthermore, the activity of dimeric half-sites is cooperative, dependent on a strict 3-bp spacing, and tuned by the identity of the spacer nucleotides. Saturating single-nucleotide mutagenesis of 195 CRX binding sites showed that, on average, changes in TF binding site affinity are correlated with changes in regulatory activity, but this relationship is obscured when considering mutations across multiple cis-regulatory elements (CREs). Taken together, these results demonstrate that the activity of CRX binding sites is highly dependent on sequence context, providing insight into photoreceptor gene regulation and illustrating functional principles of homeodomain binding sites that may be conserved in other cell types.
© 2018 Hughes et al.; Published by Cold Spring Harbor Laboratory Press.
Figures
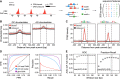
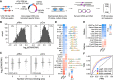
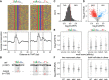
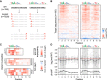
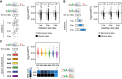
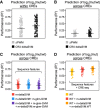
Similar articles
-
Information content differentiates enhancers from silencers in mouse photoreceptors.Elife. 2021 Sep 6;10:e67403. doi: 10.7554/eLife.67403. Elife. 2021. PMID: 34486522 Free PMC article.
-
In silico modeling of epigenetic-induced changes in photoreceptor cis-regulatory elements.Mol Vis. 2018 Mar 14;24:218-230. eCollection 2018. Mol Vis. 2018. PMID: 29563767 Free PMC article.
-
A Simple Grammar Defines Activating and Repressing cis-Regulatory Elements in Photoreceptors.Cell Rep. 2016 Oct 25;17(5):1247-1254. doi: 10.1016/j.celrep.2016.09.066. Cell Rep. 2016. PMID: 27783940 Free PMC article.
-
High-Throughput Analysis of Retinal Cis-Regulatory Networks by Massively Parallel Reporter Assays.Adv Exp Med Biol. 2019;1185:359-364. doi: 10.1007/978-3-030-27378-1_59. Adv Exp Med Biol. 2019. PMID: 31884638 Free PMC article. Review.
-
Homotypic clusters of transcription factor binding sites: A model system for understanding the physical mechanics of gene expression.Comput Struct Biotechnol J. 2014 Aug 1;10(17):63-9. doi: 10.1016/j.csbj.2014.07.005. eCollection 2014 Jul. Comput Struct Biotechnol J. 2014. PMID: 25349675 Free PMC article. Review.
Cited by
-
Quantitative Dissection of the Proximal Ciona brachyury Enhancer.Front Cell Dev Biol. 2022 Jan 21;9:804032. doi: 10.3389/fcell.2021.804032. eCollection 2021. Front Cell Dev Biol. 2022. PMID: 35127721 Free PMC article.
-
Machine Learning Prediction of Non-Coding Variant Impact in Human Retinal cis-Regulatory Elements.Transl Vis Sci Technol. 2022 Apr 1;11(4):16. doi: 10.1167/tvst.11.4.16. Transl Vis Sci Technol. 2022. PMID: 35435921 Free PMC article.
-
Quantitative-enhancer-FACS-seq (QeFS) reveals epistatic interactions among motifs within transcriptional enhancers in developing Drosophila tissue.Genome Biol. 2021 Dec 20;22(1):348. doi: 10.1186/s13059-021-02574-x. Genome Biol. 2021. PMID: 34930411 Free PMC article.
-
Transcription factor interactions explain the context-dependent activity of CRX binding sites.PLoS Comput Biol. 2024 Jan 16;20(1):e1011802. doi: 10.1371/journal.pcbi.1011802. eCollection 2024 Jan. PLoS Comput Biol. 2024. PMID: 38227575 Free PMC article.
-
Binding and Regulation of Transcription by Yeast Ste12 Variants To Drive Mating and Invasion Phenotypes.Genetics. 2020 Feb;214(2):397-407. doi: 10.1534/genetics.119.302929. Epub 2019 Dec 6. Genetics. 2020. PMID: 31810988 Free PMC article.
References
-
- Abrahams A, Parker MI, Prince S. 2010. The T-box transcription factor Tbx2: its role in development and possible implication in cancer. IUBMB Life 62: 92–102. - PubMed
-
- Arnold CD, Gerlach D, Stelzer C, Boryn LM, Rath M, Stark A. 2013. Genome-wide quantitative enhancer activity maps identified by STARR-seq. Science 339: 1074–1077. - PubMed
Publication types
MeSH terms
Substances
Grants and funding
LinkOut - more resources
Full Text Sources
Other Literature Sources
Molecular Biology Databases
Research Materials
Miscellaneous