Predicting HLA CD4 Immunogenicity in Human Populations
- PMID: 29963059
- PMCID: PMC6010533
- DOI: 10.3389/fimmu.2018.01369
Predicting HLA CD4 Immunogenicity in Human Populations
Abstract
Background: Prediction of T cell immunogenicity is a topic of considerable interest, both in terms of basic understanding of the mechanisms of T cells responses and in terms of practical applications. HLA binding affinity is often used to predict T cell epitopes, since HLA binding affinity is a key requisite for human T cell immunogenicity. However, immunogenicity at the population it is complicated by the high level of variability of HLA molecules, potential other factors beyond HLA as well as the frequent lack of HLA typing data. To overcome those issues, we explored an alternative approach to identify the common characteristics able to distinguish immunogenic peptides from non-recognized peptides.
Methods: Sets of dominant epitopes derived from peer-reviewed published papers were used in conjunction with negative peptides from the same experiments/donors to train neural networks and generate an "immunogenicity score." We also compared the performance of the immunogenicity score with previously described method for immunogenicity prediction based on HLA class II binding at the population level.
Results: The immunogenicity score was validated on a series of independent datasets derived from the published literature, representing 57 independent studies where immunogenicity in human populations was assessed by testing overlapping peptides spanning different antigens. Overall, these testing datasets corresponded to over 2,000 peptides and tested in over 1,600 different human donors. The 7-allele method prediction and the immunogenicity score were associated with similar performance [average area under the ROC curve (AUC) values of 0.703 and 0.702, respectively] while the combined methods reached an average AUC of 0.725. This increase in average AUC value is significant compared with the immunogenicity score (p = 0.0135) and a strong trend toward significance is observed when compared to the 7-allele method (p = 0.0938). The new immunogenicity score method is now freely available using CD4 T cell immunogenicity prediction tool on the Immune Epitope Database website (http://tools.iedb.org/CD4episcore).
Conclusion: The new immunogenicity score predicts CD4 T cell immunogenicity at the population level starting from protein sequences and with no need for HLA typing. Its efficacy has been validated in the context of different antigen sources, ethnicities, and disparate techniques for epitope identification.
Keywords: HLA; TCR repertoire; bioinformatics; epitopes; immunodominance; immunogenicity; predictions.
Figures
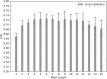
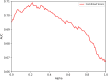
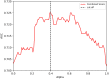
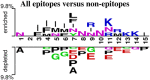
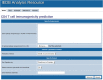
Similar articles
-
Development and validation of a broad scheme for prediction of HLA class II restricted T cell epitopes.J Immunol Methods. 2015 Jul;422:28-34. doi: 10.1016/j.jim.2015.03.022. Epub 2015 Apr 7. J Immunol Methods. 2015. PMID: 25862607 Free PMC article.
-
DeepNetBim: deep learning model for predicting HLA-epitope interactions based on network analysis by harnessing binding and immunogenicity information.BMC Bioinformatics. 2021 May 5;22(1):231. doi: 10.1186/s12859-021-04155-y. BMC Bioinformatics. 2021. PMID: 33952199 Free PMC article.
-
PREDIVAC: CD4+ T-cell epitope prediction for vaccine design that covers 95% of HLA class II DR protein diversity.BMC Bioinformatics. 2013 Feb 14;14:52. doi: 10.1186/1471-2105-14-52. BMC Bioinformatics. 2013. PMID: 23409948 Free PMC article.
-
A comprehensive review and performance evaluation of bioinformatics tools for HLA class I peptide-binding prediction.Brief Bioinform. 2020 Jul 15;21(4):1119-1135. doi: 10.1093/bib/bbz051. Brief Bioinform. 2020. PMID: 31204427 Free PMC article. Review.
-
Identification of autoantigen epitopes in MHC class II transgenic mice.Immunol Rev. 1998 Aug;164:129-38. doi: 10.1111/j.1600-065x.1998.tb01215.x. Immunol Rev. 1998. PMID: 9795771 Review.
Cited by
-
Cross-variant proof predictive vaccine design based on SARS-CoV-2 spike protein using immunoinformatics approach.Beni Suef Univ J Basic Appl Sci. 2023;12(1):5. doi: 10.1186/s43088-023-00341-4. Epub 2023 Jan 10. Beni Suef Univ J Basic Appl Sci. 2023. PMID: 36644779 Free PMC article.
-
Block-Removed Immunoglobulin Technology to enhance rituximab effector function by counteracting CA125-mediated immunosuppression.Oncol Lett. 2022 Jan;23(1):2. doi: 10.3892/ol.2021.13120. Epub 2021 Nov 2. Oncol Lett. 2022. PMID: 34820001 Free PMC article.
-
Epitope prediction and identification- adaptive T cell responses in humans.Semin Immunol. 2020 Aug;50:101418. doi: 10.1016/j.smim.2020.101418. Epub 2020 Oct 31. Semin Immunol. 2020. PMID: 33131981 Free PMC article. Review.
-
CoronaVR: A Computational Resource and Analysis of Epitopes and Therapeutics for Severe Acute Respiratory Syndrome Coronavirus-2.Front Microbiol. 2020 Jul 31;11:1858. doi: 10.3389/fmicb.2020.01858. eCollection 2020. Front Microbiol. 2020. PMID: 32849449 Free PMC article.
-
Artificial intelligence-driven reverse vaccinology for Neisseria gonorrhoeae vaccine: Prioritizing epitope-based candidates.Front Mol Biosci. 2024 Aug 13;11:1442158. doi: 10.3389/fmolb.2024.1442158. eCollection 2024. Front Mol Biosci. 2024. PMID: 39193221 Free PMC article.
References
Grants and funding
LinkOut - more resources
Full Text Sources
Other Literature Sources
Research Materials