More than fishing for a cure: The promises and pitfalls of high throughput cancer cell line screens
- PMID: 29953899
- PMCID: PMC7001883
- DOI: 10.1016/j.pharmthera.2018.06.014
More than fishing for a cure: The promises and pitfalls of high throughput cancer cell line screens
Abstract
High-throughput screens in cancer cell lines (CCLs) have been used for decades to help researchers identify compounds with the potential to improve the treatment of cancer and, more recently, to identify genomic susceptibilities in cancer via genome-wide shRNA and CRISPR/Cas9 screens. Additionally, rich genomic and transcriptomic data of these CCLs has allowed researchers to pair this screening data with biological features, enabling efforts to identify biomarkers of treatment response and gene dependencies. In this paper, we review the major CCL screening efforts and the large datasets these screens have made available. We also assess the CCL screens collectively and include a resource with harmonized CCL and compound identifiers to facilitate comparisons across screens. The CCLs in these screens were found to represent a wide range of cancer types, with a strong correlation between the representation of a cancer type and its associated mortality. Patient ages and gender distributions of CCLs were generally as expected, with some notable exceptions of female underrepresentation in certain disease types. Also, ethnicity information, while largely incomplete, suggests that African American and Hispanic patients may be severely underrepresented in these screens. Nearly all genes were targeted in the genetic perturbations screens, but the compounds used for the drug screens target less than half of known cancer drivers, likely reflecting known limitations in our drug design capabilities. Finally, we discuss recent developments in the field and the promise they hold for enabling future screens to overcome previous limitations and lead to new breakthroughs in cancer treatment.
Keywords: Biomarkers; Cancer; Cell lines; Drug screens; Genetic perturbation screens; Pharmacogenomics.
Copyright © 2018 Elsevier Inc. All rights reserved.
Conflict of interest statement
Conflict of Interest Statement:
The authors declare that there are no conflicts of interest.
Figures
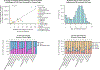
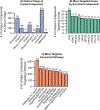
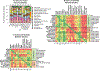
Similar articles
-
New tools for old drugs: Functional genetic screens to optimize current chemotherapy.Drug Resist Updat. 2018 Jan;36:30-46. doi: 10.1016/j.drup.2018.01.001. Epub 2018 Jan 12. Drug Resist Updat. 2018. PMID: 29499836 Free PMC article. Review.
-
Agreement between two large pan-cancer CRISPR-Cas9 gene dependency data sets.Nat Commun. 2019 Dec 20;10(1):5817. doi: 10.1038/s41467-019-13805-y. Nat Commun. 2019. PMID: 31862961 Free PMC article.
-
CRISPR Screens Provide a Comprehensive Assessment of Cancer Vulnerabilities but Generate False-Positive Hits for Highly Amplified Genomic Regions.Cancer Discov. 2016 Aug;6(8):900-13. doi: 10.1158/2159-8290.CD-16-0178. Epub 2016 Jun 3. Cancer Discov. 2016. PMID: 27260157
-
Modernizing the NCI60 Cell Line Screen for Phenotypic Drug Discovery in the 21st Century.Cancer Res. 2024 Aug 1;84(15):2397-2399. doi: 10.1158/0008-5472.CAN-24-1506. Cancer Res. 2024. PMID: 39086314
-
Hacking the Cancer Genome: Profiling Therapeutically Actionable Long Non-coding RNAs Using CRISPR-Cas9 Screening.Cancer Cell. 2019 Apr 15;35(4):545-557. doi: 10.1016/j.ccell.2019.01.019. Epub 2019 Feb 28. Cancer Cell. 2019. PMID: 30827888 Review.
Cited by
-
Computational drug discovery pipelines identify NAMPT as a therapeutic target in neuroendocrine prostate cancer.Clin Transl Sci. 2024 Sep;17(9):e70030. doi: 10.1111/cts.70030. Clin Transl Sci. 2024. PMID: 39295559 Free PMC article.
-
Machine learning approaches to drug response prediction: challenges and recent progress.NPJ Precis Oncol. 2020 Jun 15;4:19. doi: 10.1038/s41698-020-0122-1. eCollection 2020. NPJ Precis Oncol. 2020. PMID: 32566759 Free PMC article. Review.
-
Identification of 5 Hub Genes Related to the Early Diagnosis, Tumour Stage, and Poor Outcomes of Hepatitis B Virus-Related Hepatocellular Carcinoma by Bioinformatics Analysis.Comput Math Methods Med. 2021 Sep 23;2021:9991255. doi: 10.1155/2021/9991255. eCollection 2021. Comput Math Methods Med. 2021. PMID: 34603487 Free PMC article.
-
A review of computational methods for predicting cancer drug response at the single-cell level through integration with bulk RNAseq data.Curr Opin Struct Biol. 2024 Feb;84:102745. doi: 10.1016/j.sbi.2023.102745. Epub 2023 Dec 17. Curr Opin Struct Biol. 2024. PMID: 38109840 Free PMC article. Review.
-
Simplicity: web-based visualization and analysis of high-throughput cancer cell line screens.bioRxiv [Preprint]. 2023 Sep 12:2023.09.08.556619. doi: 10.1101/2023.09.08.556619. bioRxiv. 2023. Update in: J Cancer Sci Clin Ther. 2023;7(4):249-252. doi: 10.26502/jcsct.5079217 PMID: 37745579 Free PMC article. Updated. Preprint.
References
Publication types
MeSH terms
Substances
Grants and funding
LinkOut - more resources
Full Text Sources
Other Literature Sources