Development and application of an integrated allele-specific pipeline for methylomic and epigenomic analysis (MEA)
- PMID: 29907088
- PMCID: PMC6003194
- DOI: 10.1186/s12864-018-4835-2
Development and application of an integrated allele-specific pipeline for methylomic and epigenomic analysis (MEA)
Abstract
Background: Allele-specific transcriptional regulation, including of imprinted genes, is essential for normal mammalian development. While the regulatory regions controlling imprinted genes are associated with DNA methylation (DNAme) and specific histone modifications, the interplay between transcription and these epigenetic marks at allelic resolution is typically not investigated genome-wide due to a lack of bioinformatic packages that can process and integrate multiple epigenomic datasets with allelic resolution. In addition, existing ad-hoc software only consider SNVs for allele-specific read discovery. This limitation omits potentially informative INDELs, which constitute about one fifth of the number of SNVs in mice, and introduces a systematic reference bias in allele-specific analyses.
Results: Here, we describe MEA, an INDEL-aware Methylomic and Epigenomic Allele-specific analysis pipeline which enables user-friendly data exploration, visualization and interpretation of allelic imbalance. Applying MEA to mouse embryonic datasets yields robust allele-specific DNAme maps and low reference bias. We validate allele-specific DNAme at known differentially methylated regions and show that automated integration of such methylation data with RNA- and ChIP-seq datasets yields an intuitive, multidimensional view of allelic gene regulation. MEA uncovers numerous novel dynamically methylated loci, highlighting the sensitivity of our pipeline. Furthermore, processing and visualization of epigenomic datasets from human brain reveals the expected allele-specific enrichment of H3K27ac and DNAme at imprinted as well as novel monoallelically expressed genes, highlighting MEA's utility for integrating human datasets of distinct provenance for genome-wide analysis of allelic phenomena.
Conclusions: Our novel pipeline for standardized allele-specific processing and visualization of disparate epigenomic and methylomic datasets enables rapid analysis and navigation with allelic resolution. MEA is freely available as a Docker container at https://github.com/julienrichardalbert/MEA .
Keywords: Allele-specific; Allelic; ChIP; ChIP-seq; Chromatin immunoprecipitation; Epigenomics; Imprinting; MEA; RNA-seq; WGBS; Whole genome bisulphite-sequencing.
Conflict of interest statement
Ethics approval and consent to participate
Not applicable.
Competing interests
The authors declare that they have no competing interests.
Publisher’s Note
Springer Nature remains neutral with regard to jurisdictional claims in published maps and institutional affiliations.
Figures
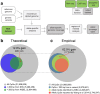
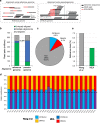
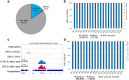
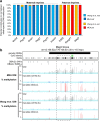
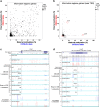
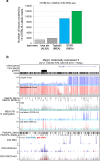
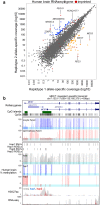
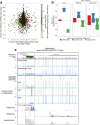
Similar articles
-
ALEA: a toolbox for allele-specific epigenomics analysis.Bioinformatics. 2014 Apr 15;30(8):1172-1174. doi: 10.1093/bioinformatics/btt744. Epub 2013 Dec 26. Bioinformatics. 2014. PMID: 24371156
-
Non-referenced genome assembly from epigenomic short-read data.Epigenetics. 2014 Oct;9(10):1329-38. doi: 10.4161/15592294.2014.969610. Epigenetics. 2014. PMID: 25437048 Free PMC article.
-
Functional variation in allelic methylomes underscores a strong genetic contribution and reveals novel epigenetic alterations in the human epigenome.Genome Biol. 2017 Mar 10;18(1):50. doi: 10.1186/s13059-017-1173-7. Genome Biol. 2017. PMID: 28283040 Free PMC article.
-
Genome-wide epigenomic profiling for biomarker discovery.Clin Epigenetics. 2016 Nov 21;8:122. doi: 10.1186/s13148-016-0284-4. eCollection 2016. Clin Epigenetics. 2016. PMID: 27895806 Free PMC article. Review.
-
The dynamic chromatin landscape and mechanisms of DNA methylation during mouse germ cell development.Genes Genet Syst. 2022 Jun 4;97(1):3-14. doi: 10.1266/ggs.21-00069. Epub 2022 Apr 16. Genes Genet Syst. 2022. PMID: 35431282 Review.
Cited by
-
Allele-specific differential regulation of monoallelically expressed autosomal genes in the cardiac lineage.Nat Commun. 2022 Oct 10;13(1):5984. doi: 10.1038/s41467-022-33722-x. Nat Commun. 2022. PMID: 36216821 Free PMC article.
-
Pervasive Inter-Individual Variation in Allele-Specific Expression in Monozygotic Twins.Front Genet. 2019 Nov 26;10:1178. doi: 10.3389/fgene.2019.01178. eCollection 2019. Front Genet. 2019. PMID: 31850058 Free PMC article.
-
LTR retrotransposons transcribed in oocytes drive species-specific and heritable changes in DNA methylation.Nat Commun. 2018 Aug 20;9(1):3331. doi: 10.1038/s41467-018-05841-x. Nat Commun. 2018. PMID: 30127397 Free PMC article.
-
Elimination of Reference Mapping Bias Reveals Robust Immune Related Allele-Specific Expression in Crossbred Sheep.Front Genet. 2019 Sep 19;10:863. doi: 10.3389/fgene.2019.00863. eCollection 2019. Front Genet. 2019. PMID: 31608110 Free PMC article.
-
Biophysics is reshaping our perception of the epigenome: from DNA-level to high-throughput studies.Biophys Rep (N Y). 2021 Sep 29;1(2):100028. doi: 10.1016/j.bpr.2021.100028. eCollection 2021 Dec 8. Biophys Rep (N Y). 2021. PMID: 36425454 Free PMC article. Review.
References
-
- Holliday R. Genomic imprinting and allelic exclusion. Development. 1990;108(Supplement):125–129. - PubMed
Publication types
MeSH terms
Grants and funding
LinkOut - more resources
Full Text Sources
Other Literature Sources
Molecular Biology Databases