VariFunNet, an integrated multiscale modeling framework to study the effects of rare non-coding variants in Genome-Wide Association Studies: applied to Alzheimer's Disease
- PMID: 29692948
- PMCID: PMC5911367
- DOI: 10.1109/BIBM.2017.8217995
VariFunNet, an integrated multiscale modeling framework to study the effects of rare non-coding variants in Genome-Wide Association Studies: applied to Alzheimer's Disease
Abstract
It is a grand challenge to reveal the causal effects of DNA variants in complex phenotypes. Although statistical techniques can establish correlations between genotypes and phenotypes in Genome-Wide Association Studies (GWAS), they often fail when the variant is rare. The emerging Network-based Association Studies aim to address this shortcoming in statistical analysis, but are mainly applied to coding variations. Increasing evidences suggest that non-coding variants play critical roles in the etiology of complex diseases. However, few computational tools are available to study the effect of rare non-coding variants on phenotypes. Here we have developed a multiscale modeling variant-to-function-to-network framework VariFunNet to address these challenges. VariFunNet first predict the functional variations of molecular interactions, which result from the non-coding variants. Then we incorporate the genes associated with the functional variation into a tissue-specific gene network, and identify subnetworks that transmit the functional variation to molecular phenotypes. Finally, we quantify the functional implication of the subnetwork, and prioritize the association of the non-coding variants with the phenotype. We have applied VariFunNet to investigating the causal effect of rare non-coding variants on Alzheimer's disease (AD). Among top 21 ranked causal non-coding variants, 16 of them are directly supported by existing evidences. The remaining 5 novel variants dysregulate multiple downstream biological processes, all of which are associated with the pathology of AD. Furthermore, we propose potential new drug targets that may modulate diverse pathways responsible for AD. These findings may shed new light on discovering new biomarkers and therapies for the prevention, diagnosis, and treatment of AD. Our results suggest that multiscale modeling is a potentially powerful approach to studying causal genotype-phenotype associations.
Keywords: RNA binding; complex disease; network robustness; single nucleotide polymorphism; systems biology; transcription factor.
Figures
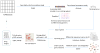
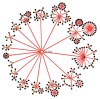
Similar articles
-
Multiscale modeling of the causal functional roles of nsSNPs in a genome-wide association study: application to hypoxia.BMC Genomics. 2013;14 Suppl 3(Suppl 3):S9. doi: 10.1186/1471-2164-14-S3-S9. Epub 2013 May 28. BMC Genomics. 2013. PMID: 23819581 Free PMC article.
-
Beyond association: successes and challenges in linking non-coding genetic variation to functional consequences that modulate Alzheimer's disease risk.Mol Neurodegener. 2021 Apr 21;16(1):27. doi: 10.1186/s13024-021-00449-0. Mol Neurodegener. 2021. PMID: 33882988 Free PMC article. Review.
-
Challenges and progress in interpretation of non-coding genetic variants associated with human disease.Exp Biol Med (Maywood). 2017 Jul;242(13):1325-1334. doi: 10.1177/1535370217713750. Epub 2017 Jun 5. Exp Biol Med (Maywood). 2017. PMID: 28581336 Free PMC article.
-
Quantifying functional impact of non-coding variants with multi-task Bayesian neural network.Bioinformatics. 2020 Mar 1;36(5):1397-1404. doi: 10.1093/bioinformatics/btz767. Bioinformatics. 2020. PMID: 31693090
-
Interpreting functional effects of coding variants: challenges in proteome-scale prediction, annotation and assessment.Brief Bioinform. 2016 Sep;17(5):841-62. doi: 10.1093/bib/bbv084. Epub 2015 Oct 22. Brief Bioinform. 2016. PMID: 26494363 Free PMC article. Review.
Cited by
-
Long noncoding RNA LINC00341 promotes the vascular smooth muscle cells proliferation and migration via miR-214/FOXO4 feedback loop.Am J Transl Res. 2019 Mar 15;11(3):1835-1842. eCollection 2019. Am J Transl Res. 2019. PMID: 30972207 Free PMC article.
-
Family-Based Quantitative Trait Meta-Analysis Implicates Rare Noncoding Variants in DENND1A in Polycystic Ovary Syndrome.J Clin Endocrinol Metab. 2019 Sep 1;104(9):3835-3850. doi: 10.1210/jc.2018-02496. J Clin Endocrinol Metab. 2019. PMID: 31038695 Free PMC article.
References
-
- Bickel PJ, Brown JB, Huang H, Li Q. An overview of recent developments in genomics and associated statistical methods. Philos Trans A Math Phys Eng Sci. 2009 Nov 13;367:4313–37. - PubMed
-
- Taylor IW, Linding R, Warde-Farley D, Liu Y, Pesquita C, Faria D, et al. Dynamic modularity in protein interaction networks predicts breast cancer outcome. Nat Biotechnol. 2009 Feb;27:199–204. - PubMed
-
- Lage K, Karlberg EO, Storling ZM, Olason PI, Pedersen AG, Rigina O, et al. A human phenome-interactome network of protein complexes implicated in genetic disorders. Nat Biotechnol. 2007 Mar;25:309–16. - PubMed
Grants and funding
LinkOut - more resources
Full Text Sources
Other Literature Sources