A novel k-mer set memory (KSM) motif representation improves regulatory variant prediction
- PMID: 29654070
- PMCID: PMC5991515
- DOI: 10.1101/gr.226852.117
A novel k-mer set memory (KSM) motif representation improves regulatory variant prediction
Abstract
The representation and discovery of transcription factor (TF) sequence binding specificities is critical for understanding gene regulatory networks and interpreting the impact of disease-associated noncoding genetic variants. We present a novel TF binding motif representation, the k-mer set memory (KSM), which consists of a set of aligned k-mers that are overrepresented at TF binding sites, and a new method called KMAC for de novo discovery of KSMs. We find that KSMs more accurately predict in vivo binding sites than position weight matrix (PWM) models and other more complex motif models across a large set of ChIP-seq experiments. Furthermore, KSMs outperform PWMs and more complex motif models in predicting in vitro binding sites. KMAC also identifies correct motifs in more experiments than five state-of-the-art motif discovery methods. In addition, KSM-derived features outperform both PWM and deep learning model derived sequence features in predicting differential regulatory activities of expression quantitative trait loci (eQTL) alleles. Finally, we have applied KMAC to 1600 ENCODE TF ChIP-seq data sets and created a public resource of KSM and PWM motifs. We expect that the KSM representation and KMAC method will be valuable in characterizing TF binding specificities and in interpreting the effects of noncoding genetic variations.
© 2018 Guo et al.; Published by Cold Spring Harbor Laboratory Press.
Figures
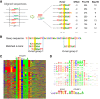
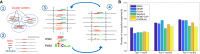
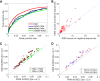
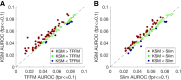
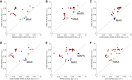
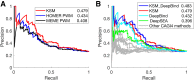
Similar articles
-
Transcription factor-binding k-mer analysis clarifies the cell type dependency of binding specificities and cis-regulatory SNPs in humans.BMC Genomics. 2023 Oct 7;24(1):597. doi: 10.1186/s12864-023-09692-9. BMC Genomics. 2023. PMID: 37805453 Free PMC article.
-
Learning position weight matrices from sequence and expression data.Comput Syst Bioinformatics Conf. 2007;6:249-60. Comput Syst Bioinformatics Conf. 2007. PMID: 17951829
-
abc4pwm: affinity based clustering for position weight matrices in applications of DNA sequence analysis.BMC Bioinformatics. 2022 Mar 3;23(1):83. doi: 10.1186/s12859-022-04615-z. BMC Bioinformatics. 2022. PMID: 35240993 Free PMC article.
-
Inferring intra-motif dependencies of DNA binding sites from ChIP-seq data.BMC Bioinformatics. 2015 Nov 9;16:375. doi: 10.1186/s12859-015-0797-4. BMC Bioinformatics. 2015. PMID: 26552868 Free PMC article.
-
An algorithmic perspective of de novo cis-regulatory motif finding based on ChIP-seq data.Brief Bioinform. 2018 Sep 28;19(5):1069-1081. doi: 10.1093/bib/bbx026. Brief Bioinform. 2018. PMID: 28334268 Review.
Cited by
-
Motto: Representing Motifs in Consensus Sequences with Minimum Information Loss.Genetics. 2020 Oct;216(2):353-358. doi: 10.1534/genetics.120.303597. Epub 2020 Aug 19. Genetics. 2020. PMID: 32816922 Free PMC article.
-
Uncovering tissue-specific binding features from differential deep learning.Nucleic Acids Res. 2020 Mar 18;48(5):e27. doi: 10.1093/nar/gkaa009. Nucleic Acids Res. 2020. PMID: 31974574 Free PMC article.
-
Ranking reprogramming factors for cell differentiation.Nat Methods. 2022 Jul;19(7):812-822. doi: 10.1038/s41592-022-01522-2. Epub 2022 Jun 16. Nat Methods. 2022. PMID: 35710610 Free PMC article.
-
VannoPortal: multiscale functional annotation of human genetic variants for interrogating molecular mechanism of traits and diseases.Nucleic Acids Res. 2022 Jan 7;50(D1):D1408-D1416. doi: 10.1093/nar/gkab853. Nucleic Acids Res. 2022. PMID: 34570217 Free PMC article.
-
HOX paralogs selectively convert binding of ubiquitous transcription factors into tissue-specific patterns of enhancer activation.PLoS Genet. 2020 Dec 14;16(12):e1009162. doi: 10.1371/journal.pgen.1009162. eCollection 2020 Dec. PLoS Genet. 2020. PMID: 33315856 Free PMC article.
References
-
- Aho AV, Corasick MJ. 1975. Efficient string matching: an aid to bibliographic search. Commun ACM 18: 333–340.
-
- Alipanahi B, Delong A, Weirauch MT, Frey BJ. 2015. Predicting the sequence specificities of DNA- and RNA-binding proteins by deep learning. Nat Biotechnol 33: 831–838. - PubMed
-
- Bailey TL, Elkan C. 1994. Fitting a mixture model by expectation maximization to discover motifs in biopolymers. Proc Int Conf Intell Syst Mol Biol 2: 28–36. - PubMed
-
- Barash Y, Bejerano G, Friedman N. 2001. A simple hyper-geometric approach for discovering putative transcription factor binding sites. In Proceedings of the First International Workshop on Algorithms in Bioinformatics, WABI ’01, pp. 278–293, Springer-Verlag, London, UK.
Publication types
MeSH terms
Substances
Grants and funding
LinkOut - more resources
Full Text Sources
Other Literature Sources
Miscellaneous