The Immune Landscape of Cancer
- PMID: 29628290
- PMCID: PMC5982584
- DOI: 10.1016/j.immuni.2018.03.023
The Immune Landscape of Cancer
Erratum in
-
The Immune Landscape of Cancer.Immunity. 2019 Aug 20;51(2):411-412. doi: 10.1016/j.immuni.2019.08.004. Immunity. 2019. PMID: 31433971 No abstract available.
Abstract
We performed an extensive immunogenomic analysis of more than 10,000 tumors comprising 33 diverse cancer types by utilizing data compiled by TCGA. Across cancer types, we identified six immune subtypes-wound healing, IFN-γ dominant, inflammatory, lymphocyte depleted, immunologically quiet, and TGF-β dominant-characterized by differences in macrophage or lymphocyte signatures, Th1:Th2 cell ratio, extent of intratumoral heterogeneity, aneuploidy, extent of neoantigen load, overall cell proliferation, expression of immunomodulatory genes, and prognosis. Specific driver mutations correlated with lower (CTNNB1, NRAS, or IDH1) or higher (BRAF, TP53, or CASP8) leukocyte levels across all cancers. Multiple control modalities of the intracellular and extracellular networks (transcription, microRNAs, copy number, and epigenetic processes) were involved in tumor-immune cell interactions, both across and within immune subtypes. Our immunogenomics pipeline to characterize these heterogeneous tumors and the resulting data are intended to serve as a resource for future targeted studies to further advance the field.
Keywords: cancer genomics; immune subtypes; immuno-oncology; immunomodulatory; immunotherapy; integrative network analysis; tumor immunology; tumor microenvironment.
Copyright © 2018 The Authors. Published by Elsevier Inc. All rights reserved.
Conflict of interest statement
DECLARATION OF INTERESTS
The authors declare no competing interests
Figures
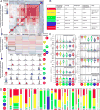
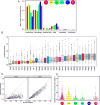
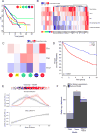
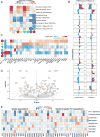
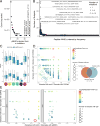
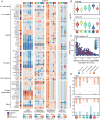
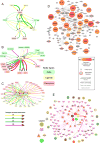
Similar articles
-
The correlation between immune subtypes and consensus molecular subtypes in colorectal cancer identifies novel tumour microenvironment profiles, with prognostic and therapeutic implications.Eur J Cancer. 2019 Dec;123:118-129. doi: 10.1016/j.ejca.2019.09.008. Epub 2019 Nov 1. Eur J Cancer. 2019. PMID: 31678770
-
Lactic acid bacteria enhance autophagic ability of mononuclear phagocytes by increasing Th1 autophagy-promoting cytokine (IFN-gamma) and nitric oxide (NO) levels and reducing Th2 autophagy-restraining cytokines (IL-4 and IL-13) in response to Mycobacterium tuberculosis antigen.Int Immunopharmacol. 2010 Jun;10(6):694-706. doi: 10.1016/j.intimp.2010.03.014. Epub 2010 Apr 7. Int Immunopharmacol. 2010. PMID: 20381647
-
Immunogenomic Landscape in Breast Cancer Reveals Immunotherapeutically Relevant Gene Signatures.Front Immunol. 2022 Jan 27;13:805184. doi: 10.3389/fimmu.2022.805184. eCollection 2022. Front Immunol. 2022. PMID: 35154121 Free PMC article.
-
The conditional role of inflammation in pregnancy and cancer.Clin Nutr. 2013 Jun;32(3):460-5. doi: 10.1016/j.clnu.2012.07.010. Epub 2012 Aug 21. Clin Nutr. 2013. PMID: 22981258 Review.
-
The soluble transforming growth factor-beta receptor: advantages and applications.Int J Biochem Cell Biol. 2009 Mar;41(3):472-6. doi: 10.1016/j.biocel.2008.01.026. Epub 2008 Feb 1. Int J Biochem Cell Biol. 2009. PMID: 18339576 Review.
Cited by
-
Machine learning and single-cell RNA sequencing reveal relationship between intratumor CD8+ T cells and uveal melanoma metastasis.Cancer Cell Int. 2024 Oct 30;24(1):359. doi: 10.1186/s12935-024-03539-3. Cancer Cell Int. 2024. PMID: 39478575 Free PMC article.
-
Human-interpretable image features derived from densely mapped cancer pathology slides predict diverse molecular phenotypes.Nat Commun. 2021 Mar 12;12(1):1613. doi: 10.1038/s41467-021-21896-9. Nat Commun. 2021. PMID: 33712588 Free PMC article.
-
A predictive signature based on enhancer RNA associates with immune infiltration and aids treatment decision in clear cell renal cell carcinoma.Front Oncol. 2022 Oct 12;12:964838. doi: 10.3389/fonc.2022.964838. eCollection 2022. Front Oncol. 2022. PMID: 36313627 Free PMC article.
-
Machine learning revealed stemness features and a novel stemness-based classification with appealing implications in discriminating the prognosis, immunotherapy and temozolomide responses of 906 glioblastoma patients.Brief Bioinform. 2021 Sep 2;22(5):bbab032. doi: 10.1093/bib/bbab032. Brief Bioinform. 2021. PMID: 33839757 Free PMC article.
-
Identifying an immunosenescence-associated gene signature in gastric cancer by integrating bulk and single-cell sequencing data.Sci Rep. 2024 Jul 24;14(1):17055. doi: 10.1038/s41598-024-68054-x. Sci Rep. 2024. PMID: 39048596 Free PMC article.
References
-
- Bailey P, Chang DK, Nones K, Johns AL, Patch AM, Gingras MC, Miller DK, Christ AN, Bruxner TJ, Quinn MC, et al. Genomic analyses identify molecular subtypes of pancreatic cancer. Nature. 2016;531:47–52. - PubMed
Publication types
MeSH terms
Substances
Grants and funding
- U24 CA143866/CA/NCI NIH HHS/United States
- P30 CA016086/CA/NCI NIH HHS/United States
- U54 HG003273/HG/NHGRI NIH HHS/United States
- P50 CA058223/CA/NCI NIH HHS/United States
- U24 CA144025/CA/NCI NIH HHS/United States
- U24 CA143843/CA/NCI NIH HHS/United States
- U24 CA143848/CA/NCI NIH HHS/United States
- U01 CA217858/CA/NCI NIH HHS/United States
- U24 CA210949/CA/NCI NIH HHS/United States
- U24 CA143867/CA/NCI NIH HHS/United States
- R50 CA221675/CA/NCI NIH HHS/United States
- U24 CA210990/CA/NCI NIH HHS/United States
- P30 ES010126/ES/NIEHS NIH HHS/United States
- P30 CA016672/CA/NCI NIH HHS/United States
- U24 CA143882/CA/NCI NIH HHS/United States
- U54 CA209997/CA/NCI NIH HHS/United States
- U54 HG003067/HG/NHGRI NIH HHS/United States
- U24 CA143835/CA/NCI NIH HHS/United States
- R01 LM009239/LM/NLM NIH HHS/United States
- U24 CA180924/CA/NCI NIH HHS/United States
- U24 CA210950/CA/NCI NIH HHS/United States
- R01 CA184585/CA/NCI NIH HHS/United States
- U24 CA143845/CA/NCI NIH HHS/United States
- U24 CA143799/CA/NCI NIH HHS/United States
- S10 OD012351/OD/NIH HHS/United States
- U24 CA143840/CA/NCI NIH HHS/United States
- U24 CA143858/CA/NCI NIH HHS/United States
- P20 GM130423/GM/NIGMS NIH HHS/United States
- U24 CA210957/CA/NCI NIH HHS/United States
- P30 CA045508/CA/NCI NIH HHS/United States
- U54 HG003079/HG/NHGRI NIH HHS/United States
- U24 CA210969/CA/NCI NIH HHS/United States
- U24 CA143883/CA/NCI NIH HHS/United States
- S10 OD021764/OD/NIH HHS/United States
- R01 CA163722/CA/NCI NIH HHS/United States
- R35 CA197745/CA/NCI NIH HHS/United States
- K24 CA169004/CA/NCI NIH HHS/United States
LinkOut - more resources
Full Text Sources
Other Literature Sources
Medical
Research Materials
Miscellaneous