Integrated analysis sheds light on evolutionary trajectories of young transcription start sites in the human genome
- PMID: 29618487
- PMCID: PMC5932608
- DOI: 10.1101/gr.231449.117
Integrated analysis sheds light on evolutionary trajectories of young transcription start sites in the human genome
Abstract
Understanding the molecular mechanisms and evolution of the gene regulatory system remains a major challenge in biology. Transcription start sites (TSSs) are especially interesting because they are central to initiating gene expression. Previous studies revealed widespread transcription initiation and fast turnover of TSSs in mammalian genomes. Yet, how new TSSs originate and how they evolve over time remain poorly understood. To address these questions, we analyzed ∼200,000 human TSSs by integrating evolutionary (inter- and intra-species) and functional genomic data, particularly focusing on evolutionarily young TSSs that emerged in the primate lineage. TSSs were grouped according to their evolutionary age using sequence alignment information as a proxy. Comparisons of young and old TSSs revealed that (1) new TSSs emerge through a combination of intrinsic factors, like the sequence properties of transposable elements and tandem repeats, and extrinsic factors such as their proximity to existing regulatory modules; (2) new TSSs undergo rapid evolution that reduces the inherent instability of repeat sequences associated with a high propensity of TSS emergence; and (3) once established, the transcriptional competence of surviving TSSs is gradually enhanced, with evolutionary changes subject to temporal (fewer regulatory changes in younger TSSs) and spatial constraints (fewer regulatory changes in more isolated TSSs). These findings advance our understanding of how regulatory innovations arise in the genome throughout evolution and highlight the genomic robustness and evolvability in these processes.
© 2018 Li et al.; Published by Cold Spring Harbor Laboratory Press.
Figures
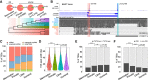
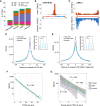
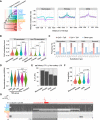
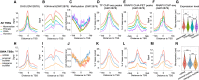
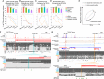
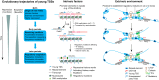
Similar articles
-
Evolutionary rates and patterns for human transcription factor binding sites derived from repetitive DNA.BMC Genomics. 2008 May 17;9:226. doi: 10.1186/1471-2164-9-226. BMC Genomics. 2008. PMID: 18485226 Free PMC article.
-
Simple and accurate transcriptional start site identification using Smar2C2 and examination of conserved promoter features.Plant J. 2022 Oct;112(2):583-596. doi: 10.1111/tpj.15957. Epub 2022 Oct 2. Plant J. 2022. PMID: 36030508 Free PMC article.
-
GPMiner: an integrated system for mining combinatorial cis-regulatory elements in mammalian gene group.BMC Genomics. 2012;13 Suppl 1(Suppl 1):S3. doi: 10.1186/1471-2164-13-S1-S3. Epub 2012 Jan 17. BMC Genomics. 2012. PMID: 22369687 Free PMC article.
-
Functions of DNA methylation: islands, start sites, gene bodies and beyond.Nat Rev Genet. 2012 May 29;13(7):484-92. doi: 10.1038/nrg3230. Nat Rev Genet. 2012. PMID: 22641018 Review.
-
Exploring the genesis and functions of Human Accelerated Regions sheds light on their role in human evolution.Curr Opin Genet Dev. 2014 Dec;29:15-21. doi: 10.1016/j.gde.2014.07.005. Epub 2014 Aug 23. Curr Opin Genet Dev. 2014. PMID: 25156517 Review.
Cited by
-
De novo activated transcription of inserted foreign coding sequences is inheritable in the plant genome.PLoS One. 2021 Jun 10;16(6):e0252674. doi: 10.1371/journal.pone.0252674. eCollection 2021. PLoS One. 2021. PMID: 34111139 Free PMC article.
-
Evolutionary dynamics of circular RNAs in primates.Elife. 2021 Sep 20;10:e69148. doi: 10.7554/eLife.69148. Elife. 2021. PMID: 34542404 Free PMC article.
-
Repurposing of promoters and enhancers during mammalian evolution.Nat Commun. 2018 Oct 4;9(1):4066. doi: 10.1038/s41467-018-06544-z. Nat Commun. 2018. PMID: 30287902 Free PMC article.
-
The Role of XPB/Ssl2 dsDNA Translocase Processivity in Transcription Start-site Scanning.J Mol Biol. 2021 Jul 9;433(14):166813. doi: 10.1016/j.jmb.2021.166813. Epub 2021 Jan 13. J Mol Biol. 2021. PMID: 33453189 Free PMC article.
-
Skewing of the genetic architecture at the ZMYM3 human-specific 5' UTR short tandem repeat in schizophrenia.Mol Genet Genomics. 2018 Jun;293(3):747-752. doi: 10.1007/s00438-018-1415-8. Epub 2018 Jan 13. Mol Genet Genomics. 2018. PMID: 29332164
References
-
- Albert FW, Kruglyak L. 2015. The role of regulatory variation in complex traits and disease. Nat Rev Genet 16: 197–212. - PubMed
Publication types
MeSH terms
Substances
Grants and funding
LinkOut - more resources
Full Text Sources
Other Literature Sources