SvABA: genome-wide detection of structural variants and indels by local assembly
- PMID: 29535149
- PMCID: PMC5880247
- DOI: 10.1101/gr.221028.117
SvABA: genome-wide detection of structural variants and indels by local assembly
Abstract
Structural variants (SVs), including small insertion and deletion variants (indels), are challenging to detect through standard alignment-based variant calling methods. Sequence assembly offers a powerful approach to identifying SVs, but is difficult to apply at scale genome-wide for SV detection due to its computational complexity and the difficulty of extracting SVs from assembly contigs. We describe SvABA, an efficient and accurate method for detecting SVs from short-read sequencing data using genome-wide local assembly with low memory and computing requirements. We evaluated SvABA's performance on the NA12878 human genome and in simulated and real cancer genomes. SvABA demonstrates superior sensitivity and specificity across a large spectrum of SVs and substantially improves detection performance for variants in the 20-300 bp range, compared with existing methods. SvABA also identifies complex somatic rearrangements with chains of short (<1000 bp) templated-sequence insertions copied from distant genomic regions. We applied SvABA to 344 cancer genomes from 11 cancer types and found that short templated-sequence insertions occur in ∼4% of all somatic rearrangements. Finally, we demonstrate that SvABA can identify sites of viral integration and cancer driver alterations containing medium-sized (50-300 bp) SVs.
© 2018 Wala et al.; Published by Cold Spring Harbor Laboratory Press.
Figures
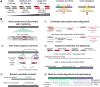
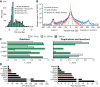
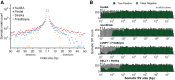
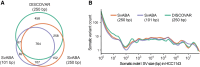
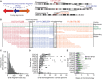
Similar articles
-
Identification of genomic indels and structural variations using split reads.BMC Genomics. 2011 Jul 25;12:375. doi: 10.1186/1471-2164-12-375. BMC Genomics. 2011. PMID: 21787423 Free PMC article.
-
VolcanoSV enables accurate and robust structural variant calling in diploid genomes from single-molecule long read sequencing.Nat Commun. 2024 Aug 13;15(1):6956. doi: 10.1038/s41467-024-51282-0. Nat Commun. 2024. PMID: 39138168 Free PMC article.
-
Comparison of structural variant callers for massive whole-genome sequence data.BMC Genomics. 2024 Mar 28;25(1):318. doi: 10.1186/s12864-024-10239-9. BMC Genomics. 2024. PMID: 38549092 Free PMC article.
-
Geographic distribution and adaptive significance of genomic structural variants: an anthropological genetics perspective.Hum Biol. 2014 Fall;86(4):260-75. doi: 10.13110/humanbiology.86.4.0260. Hum Biol. 2014. PMID: 25959693 Review.
-
A Practical Guide for Structural Variation Detection in the Human Genome.Curr Protoc Hum Genet. 2020 Sep;107(1):e103. doi: 10.1002/cphg.103. Curr Protoc Hum Genet. 2020. PMID: 32813322 Free PMC article. Review.
Cited by
-
A robust benchmark for detection of germline large deletions and insertions.Nat Biotechnol. 2020 Nov;38(11):1347-1355. doi: 10.1038/s41587-020-0538-8. Epub 2020 Jun 15. Nat Biotechnol. 2020. PMID: 32541955 Free PMC article.
-
Tangent normalization for somatic copy-number inference in cancer genome analysis.Bioinformatics. 2022 Oct 14;38(20):4677-4686. doi: 10.1093/bioinformatics/btac586. Bioinformatics. 2022. PMID: 36040167 Free PMC article.
-
Chromosome evolution screens recapitulate tissue-specific tumor aneuploidy patterns.Nat Genet. 2024 May;56(5):900-912. doi: 10.1038/s41588-024-01665-2. Epub 2024 Feb 22. Nat Genet. 2024. PMID: 38388848 Free PMC article.
-
Genomic landscape of early-stage prostate adenocarcinoma in Mexican patients: an exploratory study.Discov Oncol. 2024 Aug 28;15(1):378. doi: 10.1007/s12672-024-01199-3. Discov Oncol. 2024. PMID: 39196408 Free PMC article.
-
Long-insert sequence capture detects high copy numbers in a defence-related beta-glucosidase gene βglu-1 with large variations in white spruce but not Norway spruce.BMC Genomics. 2024 Jan 27;25(1):118. doi: 10.1186/s12864-024-09978-6. BMC Genomics. 2024. PMID: 38281030 Free PMC article.
References
-
- Campbell PJ, Getz G, Stuart JM, Korbel JO, Stein LD, ICGC/TCGA Pan-Cancer of Whole Genomes Net. 2017. Pan-cancer analysis of whole genomes. bioRxiv 10.1101/162784. - DOI
-
- Chen X, Schulz-Trieglaff O, Shaw R, Barnes B, Schlesinger F, Källberg M, Cox AJ, Kruglyak S, Saunders CT. 2016. Manta: rapid detection of structural variants and indels for germline and cancer sequencing applications. Bioinformatics 32: 1220–1222. - PubMed
Publication types
MeSH terms
Grants and funding
LinkOut - more resources
Full Text Sources
Other Literature Sources