A bayesian method for accelerated magnetic resonance elastography of the liver
- PMID: 29334131
- PMCID: PMC5980673
- DOI: 10.1002/mrm.27083
A bayesian method for accelerated magnetic resonance elastography of the liver
Abstract
Purpose: Magnetic resonance elastography (MRE) is a noninvasive tool for quantifying soft tissue stiffness. MRE has been adopted as a clinical method for staging liver fibrosis. The application of liver MRE, however, requires multiple lengthy breath holds. We propose a new data acquisition and processing method to reduce MRE scan time.
Theory and Methods: A Bayesian image reconstruction method that utilizes transform sparsity and magnitude consistency across different phase offsets to recover images from highly undersampled data is proposed. The method is validated using retrospectively downsampled phantom data and prospectively downsampled in vivo data (n=86).
Results: The proposed technique allows accurate quantification of mean liver stiffness up to an acceleration factor of R=6, enabling acquisition of a slice in 4.3 seconds. Bland Altman analysis indicates that the proposed technique (R=6) has a bias of −0.04 kPa and limits of agreement of –0.36 to +0.28 kPa when compared to traditional GRAPPA reconstruction (R=1.4).
Conclusion: By exploiting transform sparsity and magnitude consistency, accurate quantification of mean stiffness in the liver can be obtained at acceleration rate of up to R=6. This potentially enables collection of three to four liver slices, as per clinical protocol, within a single breath hold.
Keywords: Bayesian model; MRI; compressive sensing; elastography; factor graph; liver MRE.
Figures
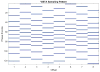
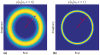
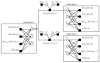
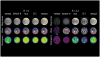
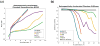
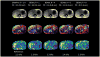
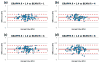
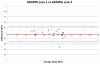
Similar articles
-
Comparison of diagnostic accuracies of two- and three-dimensional MR elastography of the liver.J Magn Reson Imaging. 2017 Apr;45(4):1163-1170. doi: 10.1002/jmri.25425. Epub 2016 Sep 23. J Magn Reson Imaging. 2017. PMID: 27662640 Free PMC article.
-
Noninvasive hepatic fibrosis staging using mr elastography: The usefulness of the bayesian prediction method.J Magn Reson Imaging. 2017 Aug;46(2):375-382. doi: 10.1002/jmri.25551. Epub 2016 Nov 9. J Magn Reson Imaging. 2017. PMID: 27859956
-
Prospective comparison of transient, point shear wave, and magnetic resonance elastography for staging liver fibrosis.Eur Radiol. 2019 Dec;29(12):6477-6488. doi: 10.1007/s00330-019-06331-4. Epub 2019 Jul 5. Eur Radiol. 2019. PMID: 31278577
-
Magnetic resonance elastography: basic principles, technique, and clinical applications in the liver.Diagn Interv Radiol. 2018 Nov;24(6):328-335. doi: 10.5152/dir.2018.18186. Diagn Interv Radiol. 2018. PMID: 30272563 Free PMC article. Review.
-
Magnetic Resonance Elastography of Liver: Current Update.Top Magn Reson Imaging. 2018 Oct;27(5):319-333. doi: 10.1097/RMR.0000000000000177. Top Magn Reson Imaging. 2018. PMID: 30289828 Free PMC article. Review.
Cited by
-
Advances and Future Direction of Magnetic Resonance Elastography.Top Magn Reson Imaging. 2018 Oct;27(5):363-384. doi: 10.1097/RMR.0000000000000179. Top Magn Reson Imaging. 2018. PMID: 30289831 Free PMC article. Review.
-
Simultaneous multislice rapid magnetic resonance elastography of the liver.NMR Biomed. 2020 Apr;33(4):e4252. doi: 10.1002/nbm.4252. Epub 2020 Jan 23. NMR Biomed. 2020. PMID: 31971301 Free PMC article.
-
OSCILLATE: A low-rank approach for accelerated magnetic resonance elastography.Magn Reson Med. 2022 Oct;88(4):1659-1672. doi: 10.1002/mrm.29308. Epub 2022 Jun 1. Magn Reson Med. 2022. PMID: 35649188 Free PMC article.
References
Publication types
MeSH terms
Grants and funding
LinkOut - more resources
Full Text Sources
Other Literature Sources
Medical