GDSCTools for mining pharmacogenomic interactions in cancer
- PMID: 29186349
- PMCID: PMC6031019
- DOI: 10.1093/bioinformatics/btx744
GDSCTools for mining pharmacogenomic interactions in cancer
Abstract
Motivation: Large pharmacogenomic screenings integrate heterogeneous cancer genomic datasets as well as anti-cancer drug responses on thousand human cancer cell lines. Mining this data to identify new therapies for cancer sub-populations would benefit from common data structures, modular computational biology tools and user-friendly interfaces.
Results: We have developed GDSCTools: a software aimed at the identification of clinically relevant genomic markers of drug response. The Genomics of Drug Sensitivity in Cancer (GDSC) database (www.cancerRxgene.org) integrates heterogeneous cancer genomic datasets as well as anti-cancer drug responses on a thousand cancer cell lines. Including statistical tools (analysis of variance) and predictive methods (Elastic Net), as well as common data structures, GDSCTools allows users to reproduce published results from GDSC and to implement new analytical methods. In addition, non-GDSC data resources can also be analysed since drug responses and genomic features can be encoded as CSV files.
Contact: thomas.cokelaer@pasteur.fr or saezrodriguez.rwth-aachen.de or mg12@sanger.ac.uk.
Supplementary information: Supplementary data are available at Bioinformatics online.
Figures
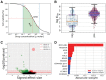
Similar articles
-
Genomics of Drug Sensitivity in Cancer (GDSC): a resource for therapeutic biomarker discovery in cancer cells.Nucleic Acids Res. 2013 Jan;41(Database issue):D955-61. doi: 10.1093/nar/gks1111. Epub 2012 Nov 23. Nucleic Acids Res. 2013. PMID: 23180760 Free PMC article.
-
Improved anticancer drug response prediction in cell lines using matrix factorization with similarity regularization.BMC Cancer. 2017 Aug 2;17(1):513. doi: 10.1186/s12885-017-3500-5. BMC Cancer. 2017. PMID: 28768489 Free PMC article.
-
Integrating heterogeneous drug sensitivity data from cancer pharmacogenomic studies.Oncotarget. 2016 Aug 9;7(32):51619-51625. doi: 10.18632/oncotarget.10010. Oncotarget. 2016. PMID: 27322211 Free PMC article.
-
Evaluating the consistency of large-scale pharmacogenomic studies.Brief Bioinform. 2019 Sep 27;20(5):1734-1753. doi: 10.1093/bib/bby046. Brief Bioinform. 2019. PMID: 31846027 Free PMC article. Review.
-
A gentle introduction to understanding preclinical data for cancer pharmaco-omic modeling.Brief Bioinform. 2021 Nov 5;22(6):bbab312. doi: 10.1093/bib/bbab312. Brief Bioinform. 2021. PMID: 34368843 Review.
Cited by
-
Transcriptional Response to Standard AML Drugs Identifies Synergistic Combinations.Int J Mol Sci. 2023 Aug 18;24(16):12926. doi: 10.3390/ijms241612926. Int J Mol Sci. 2023. PMID: 37629110 Free PMC article.
-
A Methodological Framework to Discover Pharmacogenomic Interactions Based on Random Forests.Genes (Basel). 2021 Jun 18;12(6):933. doi: 10.3390/genes12060933. Genes (Basel). 2021. PMID: 34207374 Free PMC article.
-
CellMiner Cross-Database (CellMinerCDB) version 1.2: Exploration of patient-derived cancer cell line pharmacogenomics.Nucleic Acids Res. 2021 Jan 8;49(D1):D1083-D1093. doi: 10.1093/nar/gkaa968. Nucleic Acids Res. 2021. PMID: 33196823 Free PMC article.
-
Machine learning approaches to drug response prediction: challenges and recent progress.NPJ Precis Oncol. 2020 Jun 15;4:19. doi: 10.1038/s41698-020-0122-1. eCollection 2020. NPJ Precis Oncol. 2020. PMID: 32566759 Free PMC article. Review.
-
Large-scale Pan-cancer Cell Line Screening Identifies Actionable and Effective Drug Combinations.Cancer Discov. 2024 May 1;14(5):846-865. doi: 10.1158/2159-8290.CD-23-0388. Cancer Discov. 2024. PMID: 38456804 Free PMC article.
References
-
- Köster J., Rahmann S. (2012) Snakemake–a scalable bioinformatics workflow engine. Bioinformatics, 28, 2520–2522. - PubMed
MeSH terms
Substances
LinkOut - more resources
Full Text Sources
Other Literature Sources