Metabolomics for the masses: The future of metabolomics in a personalized world
- PMID: 29094062
- PMCID: PMC5653644
- DOI: 10.1016/j.nhtm.2017.06.001
Metabolomics for the masses: The future of metabolomics in a personalized world
Abstract
Current clinical practices focus on a small number of biochemical directly related to the pathophysiology with patients and thus only describe a very limited metabolome of a patient and fail to consider the interations of these small molecules. This lack of extended information may prevent clinicians from making the best possible therapeutic interventions in sufficient time to improve patient care. Various post-genomics '('omic)' approaches have been used for therapeutic interventions previously. Metabolomics now a well-established'omics approach, has been widely adopted as a novel approach for biomarker discovery and in tandem with genomics (especially SNPs and GWAS) has the potential for providing systemic understanding of the underlying causes of pathology. In this review, we discuss the relevance of metabolomics approaches in clinical sciences and its potential for biomarker discovery which may help guide clinical interventions. Although a powerful and potentially high throughput approach for biomarker discovery at the molecular level, true translation of metabolomics into clinics is an extremely slow process. Quicker adaptation of biomarkers discovered using metabolomics can be possible with novel portable and wearable technologies aided by clever data mining, as well as deep learning and artificial intelligence; we shall also discuss this with an eye to the future of precision medicine where metabolomics can be delivered to the masses.
Figures
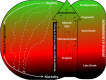
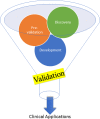
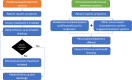
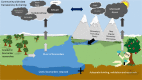
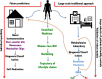
Similar articles
-
Translational Metabolomics of Head Injury: Exploring Dysfunctional Cerebral Metabolism with Ex Vivo NMR Spectroscopy-Based Metabolite Quantification.In: Kobeissy FH, editor. Brain Neurotrauma: Molecular, Neuropsychological, and Rehabilitation Aspects. Boca Raton (FL): CRC Press/Taylor & Francis; 2015. Chapter 25. In: Kobeissy FH, editor. Brain Neurotrauma: Molecular, Neuropsychological, and Rehabilitation Aspects. Boca Raton (FL): CRC Press/Taylor & Francis; 2015. Chapter 25. PMID: 26269925 Free Books & Documents. Review.
-
Metabolomics enables precision medicine: "A White Paper, Community Perspective".Metabolomics. 2016;12(10):149. doi: 10.1007/s11306-016-1094-6. Epub 2016 Sep 2. Metabolomics. 2016. PMID: 27642271 Free PMC article.
-
Metabolomics for personalized medicine: the input of analytical chemistry from biomarker discovery to point-of-care tests.Anal Bioanal Chem. 2022 Jan;414(2):759-789. doi: 10.1007/s00216-021-03586-z. Epub 2021 Aug 25. Anal Bioanal Chem. 2022. PMID: 34432105 Free PMC article. Review.
-
The future of Cochrane Neonatal.Early Hum Dev. 2020 Nov;150:105191. doi: 10.1016/j.earlhumdev.2020.105191. Epub 2020 Sep 12. Early Hum Dev. 2020. PMID: 33036834
-
Status and perspectives of biomarker validation for diagnosis, stratification, and treatment.Public Health. 2021 Jan;190:173-175. doi: 10.1016/j.puhe.2020.11.002. Epub 2020 Dec 9. Public Health. 2021. PMID: 33309006
Cited by
-
Metabolomics Research Conducted by Nurse Scientists: A Systematic Scoping Review.Biol Res Nurs. 2020 Oct;22(4):436-448. doi: 10.1177/1099800420940041. Epub 2020 Jul 10. Biol Res Nurs. 2020. PMID: 32648468 Free PMC article.
-
Tissue-Specific Metabolic Profiles After Prolonged Cardiac Arrest Reveal Brain Metabolome Dysfunction Predominantly After Resuscitation.J Am Heart Assoc. 2019 Sep 3;8(17):e012809. doi: 10.1161/JAHA.119.012809. Epub 2019 Aug 31. J Am Heart Assoc. 2019. PMID: 31475603 Free PMC article.
-
Metabolomic profiling in small vessel disease identifies multiple associations with disease severity.Brain. 2022 Jul 29;145(7):2461-2471. doi: 10.1093/brain/awac041. Brain. 2022. PMID: 35254405 Free PMC article.
-
Current Status of Metabolomic Biomarker Discovery: Impact of Study Design and Demographic Characteristics.Metabolites. 2020 May 29;10(6):224. doi: 10.3390/metabo10060224. Metabolites. 2020. PMID: 32485899 Free PMC article. Review.
-
Metabolomic Laboratory-Developed Tests: Current Status and Perspectives.Metabolites. 2021 Jun 26;11(7):423. doi: 10.3390/metabo11070423. Metabolites. 2021. PMID: 34206934 Free PMC article. Review.
References
-
- Oliver S.G. Systematic functional analysis of the yeast genome. Trends Biotechnol. 1998;16(9):373–378. - PubMed
-
- Broadhurst D.I., Kell D.B. Statistical strategies for avoiding false discoveries in metabolomics and related experiments. Metabolomics. 2006;2(4):171–196.
-
- Poste G. Bring on the biomarkers. Nature. 2011;469(7329):156–157. - PubMed
Publication types
Grants and funding
LinkOut - more resources
Full Text Sources
Other Literature Sources