An Effective Model of the Retinoic Acid Induced HL-60 Differentiation Program
- PMID: 29085021
- PMCID: PMC5662654
- DOI: 10.1038/s41598-017-14523-5
An Effective Model of the Retinoic Acid Induced HL-60 Differentiation Program
Abstract
In this study, we present an effective model All-Trans Retinoic Acid (ATRA)-induced differentiation of HL-60 cells. The model describes reinforcing feedback between an ATRA-inducible signalsome complex involving many proteins including Vav1, a guanine nucleotide exchange factor, and the activation of the mitogen activated protein kinase (MAPK) cascade. We decomposed the effective model into three modules; a signal initiation module that sensed and transformed an ATRA signal into program activation signals; a signal integration module that controlled the expression of upstream transcription factors; and a phenotype module which encoded the expression of functional differentiation markers from the ATRA-inducible transcription factors. We identified an ensemble of effective model parameters using measurements taken from ATRA-induced HL-60 cells. Using these parameters, model analysis predicted that MAPK activation was bistable as a function of ATRA exposure. Conformational experiments supported ATRA-induced bistability. Additionally, the model captured intermediate and phenotypic gene expression data. Knockout analysis suggested Gfi-1 and PPARg were critical to the ATRAinduced differentiation program. These findings, combined with other literature evidence, suggested that reinforcing feedback is central to hyperactive signaling in a diversity of cell fate programs.
Conflict of interest statement
The authors declare that they have no competing interests.
Figures
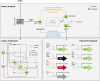
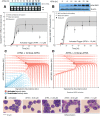
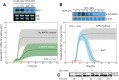
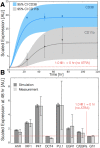
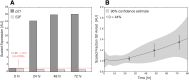
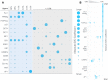
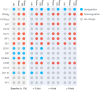
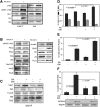
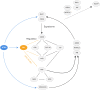
Similar articles
-
Roscovitine enhances All-trans retinoic acid (ATRA)-induced leukemia cell differentiation: Novel effects on signaling molecules for a putative Cdk2 inhibitor.Cell Signal. 2020 Jul;71:109555. doi: 10.1016/j.cellsig.2020.109555. Epub 2020 Feb 4. Cell Signal. 2020. PMID: 32032659
-
RARγ-C-Fos-PPARγ2 signaling rather than ROS generation is critical for all-trans retinoic acid-inhibited adipocyte differentiation.Biochimie. 2014 Nov;106:121-30. doi: 10.1016/j.biochi.2014.08.009. Epub 2014 Aug 28. Biochimie. 2014. PMID: 25173565
-
Vav1 and PU.1 are recruited to the CD11b promoter in APL-derived promyelocytes: role of Vav1 in modulating PU.1-containing complexes during ATRA-induced differentiation.Exp Cell Res. 2010 Jan 1;316(1):38-47. doi: 10.1016/j.yexcr.2009.09.004. Epub 2009 Sep 10. Exp Cell Res. 2010. PMID: 19747912
-
Stress-induced NF-κB activation differentiates promyelocytic leukemia cells to macrophages in response to all-trans-retinoic acid.Cell Signal. 2015 Mar;27(3):694-706. doi: 10.1016/j.cellsig.2014.11.019. Epub 2014 Nov 27. Cell Signal. 2015. PMID: 25435432
-
Vav1 in differentiation of tumoral promyelocytes.Cell Signal. 2012 Mar;24(3):612-20. doi: 10.1016/j.cellsig.2011.11.017. Epub 2011 Nov 28. Cell Signal. 2012. PMID: 22133616 Review.
Cited by
-
Characterization of iPS87, a prostate cancer stem cell-like cell line.Oncotarget. 2020 Mar 24;11(12):1075-1084. doi: 10.18632/oncotarget.27524. eCollection 2020 Mar 24. Oncotarget. 2020. PMID: 32256979 Free PMC article.
-
Molecular mechanisms of aberrant neutrophil differentiation in glycogen storage disease type Ib.Cell Mol Life Sci. 2022 Apr 18;79(5):246. doi: 10.1007/s00018-022-04267-5. Cell Mol Life Sci. 2022. PMID: 35437689 Free PMC article.
-
Systems Biology for Drug Target Discovery in Acute Myeloid Leukemia.Int J Mol Sci. 2024 Apr 23;25(9):4618. doi: 10.3390/ijms25094618. Int J Mol Sci. 2024. PMID: 38731835 Free PMC article.
-
Proteomic Profiling of HL-60 Cells during ATRA-Induced Differentiation.Bull Exp Biol Med. 2018 Aug;165(4):530-543. doi: 10.1007/s10517-018-4210-y. Epub 2018 Aug 18. Bull Exp Biol Med. 2018. PMID: 30121918 Free PMC article.
-
Retinoic acid and 6-formylindolo(3,2-b)carbazole (FICZ) combination therapy reveals putative targets for enhancing response in non-APL AML.Leuk Lymphoma. 2019 Jul;60(7):1697-1708. doi: 10.1080/10428194.2018.1543880. Epub 2018 Dec 20. Leuk Lymphoma. 2019. PMID: 30570341 Free PMC article.
References
Publication types
MeSH terms
Substances
Grants and funding
LinkOut - more resources
Full Text Sources
Other Literature Sources
Miscellaneous