Aging and sarcopenia associate with specific interactions between gut microbes, serum biomarkers and host physiology in rats
- PMID: 28783713
- PMCID: PMC5559170
- DOI: 10.18632/aging.101262
Aging and sarcopenia associate with specific interactions between gut microbes, serum biomarkers and host physiology in rats
Abstract
The microbiome has been demonstrated to play an integral role in the maintenance of many aspects of health that are also associated with aging. In order to identify areas of potential exploration and intervention, we simultaneously characterized age-related alterations in gut microbiome, muscle physiology and serum proteomic and lipidomic profiles in aged rats to define an integrated signature of the aging phenotype. We demonstrate that aging skews the composition of the gut microbiome, in particular by altering the Sutterella to Barneseilla ratio, and alters the metabolic potential of intestinal bacteria. Age-related changes of the gut microbiome were associated with the physiological decline of musculoskeletal function, and with molecular markers of nutrient processing/availability, and inflammatory/immune status in aged versus adult rats. Altogether, our study highlights that aging leads to a complex interplay between the microbiome and host physiology, and provides candidate microbial species to target physical and metabolic decline during aging by modulating gut microbial ecology.
Keywords: aging; lipidomics; microbiome; muscle physiology; proteomics; sarcopenia.
Conflict of interest statement
All authors are employees of the Nestle Institute of Health Sciences SA.
Figures
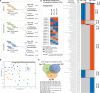
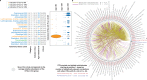
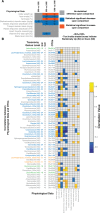
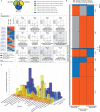
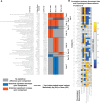
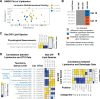
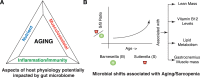
Similar articles
-
Gut Dysbiosis and Muscle Aging: Searching for Novel Targets against Sarcopenia.Mediators Inflamm. 2018 Jan 30;2018:7026198. doi: 10.1155/2018/7026198. eCollection 2018. Mediators Inflamm. 2018. PMID: 29686533 Free PMC article. Review.
-
Aging Gut Microbiota at the Cross-Road between Nutrition, Physical Frailty, and Sarcopenia: Is There a Gut-Muscle Axis?Nutrients. 2017 Nov 30;9(12):1303. doi: 10.3390/nu9121303. Nutrients. 2017. PMID: 29189738 Free PMC article. Review.
-
Gut microbiota and physical frailty through the mediation of sarcopenia.Exp Gerontol. 2019 Sep;124:110639. doi: 10.1016/j.exger.2019.110639. Epub 2019 Jun 18. Exp Gerontol. 2019. PMID: 31226349 Review.
-
Gut Microbiota, Muscle Mass and Function in Aging: A Focus on Physical Frailty and Sarcopenia.Nutrients. 2019 Jul 17;11(7):1633. doi: 10.3390/nu11071633. Nutrients. 2019. PMID: 31319564 Free PMC article. Review.
-
Gut Microbiota Contribute to Age-Related Changes in Skeletal Muscle Size, Composition, and Function: Biological Basis for a Gut-Muscle Axis.Calcif Tissue Int. 2018 Apr;102(4):433-442. doi: 10.1007/s00223-017-0345-5. Epub 2017 Oct 20. Calcif Tissue Int. 2018. PMID: 29058056 Free PMC article. Review.
Cited by
-
Effect of Isoflavone on Muscle Atrophy in Ovariectomized Mice.Nutrients. 2024 Sep 28;16(19):3295. doi: 10.3390/nu16193295. Nutrients. 2024. PMID: 39408262 Free PMC article.
-
Exploring Causal Links Between Gut Microbiota and Geriatric Syndromes: A Two-Sample Mendelian Randomization Analysis.Int J Med Sci. 2024 Jul 22;21(10):1945-1963. doi: 10.7150/ijms.94335. eCollection 2024. Int J Med Sci. 2024. PMID: 39113894 Free PMC article.
-
Harnessing immunomodulation to combat sarcopenia: current insights and possible approaches.Immun Ageing. 2024 Aug 5;21(1):55. doi: 10.1186/s12979-024-00458-9. Immun Ageing. 2024. PMID: 39103919 Free PMC article. Review.
-
Causal Relationship Between Gut Microbiota, Metabolites, and Sarcopenia: A Mendelian Randomization Study.J Gerontol A Biol Sci Med Sci. 2024 Sep 1;79(9):glae173. doi: 10.1093/gerona/glae173. J Gerontol A Biol Sci Med Sci. 2024. PMID: 38995073 Free PMC article.
-
Therapeutic strategies to modulate gut microbial health: Approaches for sarcopenia management.Histol Histopathol. 2024 Nov;39(11):1395-1425. doi: 10.14670/HH-18-730. Epub 2024 Mar 6. Histol Histopathol. 2024. PMID: 38497338 Review.
References
-
- Cho I, Blaser MJ. The human microbiome: at the interface of health and disease. Nat Rev Genet. 2012;13:260–70. https://doi.org/10.1038/nrg3182 - DOI - PMC - PubMed
-
- López-Otín C, Blasco MA, Partridge L, Serrano M, Kroemer G. The hallmarks of aging. Cell. 2013;153:1194–217. https://doi.org/10.1016/j.cell.2013.05.039 - DOI - PMC - PubMed
-
- Rampelli S, Candela M, Turroni S, Biagi E, Collino S, Franceschi C, O'Toole PW, Brigidi P. Functional metagenomic profiling of intestinal microbiome in extreme ageing. Aging (Albany NY) 2013;5:902–12. https://doi.org/10.18632/aging.100623 - DOI - PMC - PubMed
-
- Houtkooper RH, Argmann C, Houten SM, Cantó C, Jeninga EH, Andreux PA, Thomas C, Doenlen R, Schoonjans K, Auwerx J. The metabolic footprint of aging in mice. Sci Rep. 2011;1:134. https://doi.org/10.1038/srep00134 - DOI - PMC - PubMed
-
- Edwards MH, Dennison EM, Aihie Sayer A, Fielding R, Cooper C. Osteoporosis and sarcopenia in older age. Bone. 2015;80:126–30. https://doi.org/10.1016/j.bone.2015.04.016 - DOI - PMC - PubMed
Publication types
MeSH terms
Substances
LinkOut - more resources
Full Text Sources
Other Literature Sources
Medical