MDD-Palm: Identification of protein S-palmitoylation sites with substrate motifs based on maximal dependence decomposition
- PMID: 28662047
- PMCID: PMC5491019
- DOI: 10.1371/journal.pone.0179529
MDD-Palm: Identification of protein S-palmitoylation sites with substrate motifs based on maximal dependence decomposition
Abstract
S-palmitoylation, the covalent attachment of 16-carbon palmitic acids to a cysteine residue via a thioester linkage, is an important reversible lipid modification that plays a regulatory role in a variety of physiological and biological processes. As the number of experimentally identified S-palmitoylated peptides increases, it is imperative to investigate substrate motifs to facilitate the study of protein S-palmitoylation. Based on 710 non-homologous S-palmitoylation sites obtained from published databases and the literature, we carried out a bioinformatics investigation of S-palmitoylation sites based on amino acid composition. Two Sample Logo indicates that positively charged and polar amino acids surrounding S-palmitoylated sites may be associated with the substrate site specificity of protein S-palmitoylation. Additionally, maximal dependence decomposition (MDD) was applied to explore the motif signatures of S-palmitoylation sites by categorizing a large-scale dataset into subgroups with statistically significant conservation of amino acids. Single features such as amino acid composition (AAC), amino acid pair composition (AAPC), position specific scoring matrix (PSSM), position weight matrix (PWM), amino acid substitution matrix (BLOSUM62), and accessible surface area (ASA) were considered, along with the effectiveness of incorporating MDD-identified substrate motifs into a two-layered prediction model. Evaluation by five-fold cross-validation showed that a hybrid of AAC and PSSM performs best at discriminating between S-palmitoylation and non-S-palmitoylation sites, according to the support vector machine (SVM). The two-layered SVM model integrating MDD-identified substrate motifs performed well, with a sensitivity of 0.79, specificity of 0.80, accuracy of 0.80, and Matthews Correlation Coefficient (MCC) value of 0.45. Using an independent testing dataset (613 S-palmitoylated and 5412 non-S-palmitoylated sites) obtained from the literature, we demonstrated that the two-layered SVM model could outperform other prediction tools, yielding a balanced sensitivity and specificity of 0.690 and 0.694, respectively. This two-layered SVM model has been implemented as a web-based system (MDD-Palm), which is now freely available at http://csb.cse.yzu.edu.tw/MDDPalm/.
Conflict of interest statement
Figures
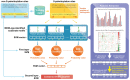
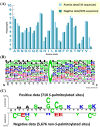
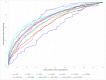
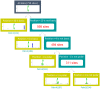
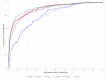
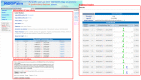
Similar articles
-
Characterization and identification of lysine glutarylation based on intrinsic interdependence between positions in the substrate sites.BMC Bioinformatics. 2019 Feb 4;19(Suppl 13):384. doi: 10.1186/s12859-018-2394-9. BMC Bioinformatics. 2019. PMID: 30717647 Free PMC article.
-
UbiSite: incorporating two-layered machine learning method with substrate motifs to predict ubiquitin-conjugation site on lysines.BMC Syst Biol. 2016 Jan 11;10 Suppl 1(Suppl 1):6. doi: 10.1186/s12918-015-0246-z. BMC Syst Biol. 2016. PMID: 26818456 Free PMC article.
-
MDD-SOH: exploiting maximal dependence decomposition to identify S-sulfenylation sites with substrate motifs.Bioinformatics. 2016 Jan 15;32(2):165-72. doi: 10.1093/bioinformatics/btv558. Epub 2015 Sep 26. Bioinformatics. 2016. PMID: 26411868
-
Protein Palmitoylation by DHHC Protein Family.In: Kittler JT, Moss SJ, editors. The Dynamic Synapse: Molecular Methods in Ionotropic Receptor Biology. Boca Raton (FL): CRC Press/Taylor & Francis; 2006. Chapter 5. In: Kittler JT, Moss SJ, editors. The Dynamic Synapse: Molecular Methods in Ionotropic Receptor Biology. Boca Raton (FL): CRC Press/Taylor & Francis; 2006. Chapter 5. PMID: 21204476 Free Books & Documents. Review.
-
Use of analogs and inhibitors to study the functional significance of protein palmitoylation.Methods. 2006 Oct;40(2):191-7. doi: 10.1016/j.ymeth.2006.04.013. Methods. 2006. PMID: 17012032 Free PMC article. Review.
Cited by
-
GNA13 regulates BCL2 expression and the sensitivity of GCB-DLBCL cells to BCL2 inhibitors in a palmitoylation-dependent manner.Cell Death Dis. 2021 Jan 9;12(1):54. doi: 10.1038/s41419-020-03311-1. Cell Death Dis. 2021. PMID: 33423045 Free PMC article.
-
A peptidic inhibitor for PD-1 palmitoylation targets its expression and functions.RSC Chem Biol. 2020 Nov 3;2(1):192-205. doi: 10.1039/d0cb00157k. eCollection 2021 Feb 1. RSC Chem Biol. 2020. PMID: 34458782 Free PMC article.
-
pCysMod: Prediction of Multiple Cysteine Modifications Based on Deep Learning Framework.Front Cell Dev Biol. 2021 Feb 23;9:617366. doi: 10.3389/fcell.2021.617366. eCollection 2021. Front Cell Dev Biol. 2021. PMID: 33732693 Free PMC article.
-
Palmitoyltransferase ZDHHC3 Aggravates Nonalcoholic Steatohepatitis by Targeting S-Palmitoylated IRHOM2.Adv Sci (Weinh). 2023 Oct;10(28):e2302130. doi: 10.1002/advs.202302130. Epub 2023 Aug 6. Adv Sci (Weinh). 2023. PMID: 37544908 Free PMC article.
-
Topology-Driven Discovery of Transmembrane Protein S-Palmitoylation.bioRxiv [Preprint]. 2024 Sep 8:2024.09.08.611865. doi: 10.1101/2024.09.08.611865. bioRxiv. 2024. PMID: 39282397 Free PMC article. Preprint.
References
-
- Dietrich LE, Ungermann C (2004) On the mechanism of protein palmitoylation. EMBO Rep 5: 1053–1057. doi: 10.1038/sj.embor.7400277 - DOI - PMC - PubMed
-
- el-Husseini Ael D, Bredt DS (2002) Protein palmitoylation: a regulator of neuronal development and function. Nat Rev Neurosci 3: 791–802. doi: 10.1038/nrn940 - DOI - PubMed
-
- Linder ME, Deschenes RJ (2003) New insights into the mechanisms of protein palmitoylation. Biochemistry 42: 4311–4320. doi: 10.1021/bi034159a - DOI - PubMed
-
- Smotrys JE, Linder ME (2004) Palmitoylation of intracellular signaling proteins: regulation and function. Annu Rev Biochem 73: 559–587. doi: 10.1146/annurev.biochem.73.011303.073954 - DOI - PubMed
-
- Huang K, El-Husseini A (2005) Modulation of neuronal protein trafficking and function by palmitoylation. Curr Opin Neurobiol 15: 527–535. doi: 10.1016/j.conb.2005.08.001 - DOI - PubMed
MeSH terms
Substances
Grants and funding
LinkOut - more resources
Full Text Sources
Other Literature Sources
Research Materials