Meta-analysis of miRNA expression profiles for prostate cancer recurrence following radical prostatectomy
- PMID: 28651018
- PMCID: PMC5484492
- DOI: 10.1371/journal.pone.0179543
Meta-analysis of miRNA expression profiles for prostate cancer recurrence following radical prostatectomy
Abstract
Background: Prostate cancer (PCa) is a leading reason of death in men and the most diagnosed malignancies in the western countries at the present time. After radical prostatectomy (RP), nearly 30% of men develop clinical recurrence with high serum prostate-specific antigen levels. An important challenge in PCa research is to identify effective predictors of tumor recurrence. The molecular alterations in microRNAs are associated with PCa initiation and progression. Several miRNA microarray studies have been conducted in recurrence PCa, but the results vary among different studies.
Methods: We conducted a meta-analysis of 6 available miRNA expression datasets to identify a panel of co-deregulated miRNA genes and overlapping biological processes. The meta-analysis was performed using the 'MetaDE' package, based on combined P-value approaches (adaptive weight and Fisher's methods), in R version 3.3.1.
Results: Meta-analysis of six miRNA datasets revealed miR-125A, miR-199A-3P, miR-28-5P, miR-301B, miR-324-5P, miR-361-5P, miR-363*, miR-449A, miR-484, miR-498, miR-579, miR-637, miR-720, miR-874 and miR-98 are commonly upregulated miRNA genes, while miR-1, miR-133A, miR-133B, miR-137, miR-221, miR-340, miR-370, miR-449B, miR-489, miR-492, miR-496, miR-541, miR-572, miR-583, miR-606, miR-624, miR-636, miR-639, miR-661, miR-760, miR-890, and miR-939 are commonly downregulated miRNA genes in recurrent PCa samples in comparison to non-recurrent PCa samples. The network-based analysis showed that some of these miRNAs have an established prognostic significance in other cancers and can be actively involved in tumor growth. Gene ontology enrichment revealed many target genes of co-deregulated miRNAs are involved in "regulation of epithelial cell proliferation" and "tissue morphogenesis". Kyoto Encyclopedia of Genes and Genomes (KEGG) analysis indicated that these miRNAs regulate cancer pathways. The PPI hub proteins analysis identified CTNNB1 as the most highly ranked hub protein. Besides, common pathway analysis showed that TCF3, MAX, MYC, CYP26A1, and SREBF1 significantly interact with those DE miRNA genes. The identified genes have been known as tumor suppressors and biomarkers which are closely related to several cancer types, such as colorectal cancer, breast cancer, PCa, gastric, and hepatocellular carcinomas. Additionally, it was shown that the combination of DE miRNAs can assist in the more specific detection of the PCa and prediction of biochemical recurrence (BCR).
Conclusion: We found that the identified miRNAs through meta-analysis are candidate predictive markers for recurrent PCa after radical prostatectomy.
Conflict of interest statement
Figures
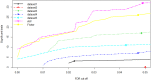
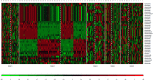
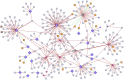
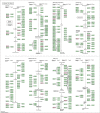
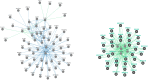
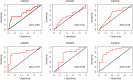
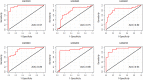
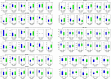
Similar articles
-
The association of let-7c, miR-21, miR-145, miR-182, and miR-221 with clinicopathologic parameters of prostate cancer in patients diagnosed with low-risk disease.Prostate. 2019 Jul;79(10):1125-1132. doi: 10.1002/pros.23825. Epub 2019 May 2. Prostate. 2019. PMID: 31045265
-
Clinical significance of miRNA host gene promoter methylation in prostate cancer.Hum Mol Genet. 2017 Jul 1;26(13):2451-2461. doi: 10.1093/hmg/ddx138. Hum Mol Genet. 2017. PMID: 28398479
-
Identification of miRNA signature in cancer-associated fibroblast to predict recurrent prostate cancer.Comput Biol Med. 2024 Sep;180:108989. doi: 10.1016/j.compbiomed.2024.108989. Epub 2024 Aug 13. Comput Biol Med. 2024. PMID: 39142223
-
Tissue-Based MicroRNAs as Predictors of Biochemical Recurrence after Radical Prostatectomy: What Can We Learn from Past Studies?Int J Mol Sci. 2017 Sep 21;18(10):2023. doi: 10.3390/ijms18102023. Int J Mol Sci. 2017. PMID: 28934131 Free PMC article. Review.
-
MiRNAs and radical prostatectomy: Current data, bioinformatic analysis and utility as predictors of tumour relapse.Andrology. 2021 Jul;9(4):1092-1107. doi: 10.1111/andr.12994. Epub 2021 Mar 24. Andrology. 2021. PMID: 33638886 Review.
Cited by
-
Regulation of Macrophage Polarization by miR-449a/Cripto-1-PI3K/AKT/NF-κB Signaling Pathway in Allogeneic Transfusion Mice.Biomed Res Int. 2023 Jan 6;2023:1277258. doi: 10.1155/2023/1277258. eCollection 2023. Biomed Res Int. 2023. PMID: 36644162 Free PMC article.
-
miR-484: A Potential Biomarker in Health and Disease.Front Oncol. 2022 Mar 9;12:830420. doi: 10.3389/fonc.2022.830420. eCollection 2022. Front Oncol. 2022. PMID: 35356223 Free PMC article. Review.
-
miRNA-29b Inhibits Prostate Tumor Growth and Induces Apoptosis by Increasing Bim Expression.Cells. 2019 Nov 18;8(11):1455. doi: 10.3390/cells8111455. Cells. 2019. PMID: 31752117 Free PMC article.
-
Positive surgical margin is associated with biochemical recurrence risk following radical prostatectomy: a meta-analysis from high-quality retrospective cohort studies.World J Surg Oncol. 2018 Jul 3;16(1):124. doi: 10.1186/s12957-018-1433-3. World J Surg Oncol. 2018. PMID: 29970100 Free PMC article. Review.
-
miR-939, as an important regulator in various cancers pathogenesis, has diagnostic, prognostic, and therapeutic values: a review.J Egypt Natl Canc Inst. 2024 Apr 29;36(1):16. doi: 10.1186/s43046-024-00220-8. J Egypt Natl Canc Inst. 2024. PMID: 38679648 Review.
References
-
- Fendler A, Jung M, Stephan C, Honey RJ, Stewart RJ, Pace KT, et al. miRNAs can predict prostate cancer biochemical relapse and are involved in tumor progression. Int J Oncol. 2011;39(5):1183–92. doi: 10.3892/ijo.2011.1128 - DOI - PubMed
-
- Barron N, Keenan J, Gammell P, Martinez VG, Freeman A, Masters JR, et al. Biochemical relapse following radical prostatectomy and miR-200a levels in prostate cancer. Prostate. 2012; 72: 1193–1199. doi: 10.1002/pros.22469 - DOI - PubMed
-
- Bhatnagar N, Li X, Padi SK, Zhang Q, Tang MS, Guo B. Downregulation of miR-205 and miR-31 confers resistance to chemotherapy-induced apoptosis in prostate cancer cells. Cell Death Dis. 2010;1: e105 doi: 10.1038/cddis.2010.85 - DOI - PMC - PubMed
-
- Ross-Adams H, Lamb AD, Dunning MJ, Halim S, Lindberg J, Massie CM, et al. Integration of copy number and transcriptomics provides risk stratification in prostate cancer: A discovery and validation cohort study. EBioMedicine. 2015. July 29; 2 (9): 1133–44. doi: 10.1016/j.ebiom.2015.07.017 - DOI - PMC - PubMed
-
- Sun Y, Goodison S. Optimizing molecular signatures for predicting prostate cancer recurrence. Prostate. 2009. July 1; 69 (10): 1119–27. doi: 10.1002/pros.20961 - DOI - PMC - PubMed
Publication types
MeSH terms
Substances
Grants and funding
LinkOut - more resources
Full Text Sources
Other Literature Sources
Medical
Miscellaneous