A comparison of per sample global scaling and per gene normalization methods for differential expression analysis of RNA-seq data
- PMID: 28459823
- PMCID: PMC5411036
- DOI: 10.1371/journal.pone.0176185
A comparison of per sample global scaling and per gene normalization methods for differential expression analysis of RNA-seq data
Abstract
Normalization is an essential step with considerable impact on high-throughput RNA sequencing (RNA-seq) data analysis. Although there are numerous methods for read count normalization, it remains a challenge to choose an optimal method due to multiple factors contributing to read count variability that affects the overall sensitivity and specificity. In order to properly determine the most appropriate normalization methods, it is critical to compare the performance and shortcomings of a representative set of normalization routines based on different dataset characteristics. Therefore, we set out to evaluate the performance of the commonly used methods (DESeq, TMM-edgeR, FPKM-CuffDiff, TC, Med UQ and FQ) and two new methods we propose: Med-pgQ2 and UQ-pgQ2 (per-gene normalization after per-sample median or upper-quartile global scaling). Our per-gene normalization approach allows for comparisons between conditions based on similar count levels. Using the benchmark Microarray Quality Control Project (MAQC) and simulated datasets, we performed differential gene expression analysis to evaluate these methods. When evaluating MAQC2 with two replicates, we observed that Med-pgQ2 and UQ-pgQ2 achieved a slightly higher area under the Receiver Operating Characteristic Curve (AUC), a specificity rate > 85%, the detection power > 92% and an actual false discovery rate (FDR) under 0.06 given the nominal FDR (≤0.05). Although the top commonly used methods (DESeq and TMM-edgeR) yield a higher power (>93%) for MAQC2 data, they trade off with a reduced specificity (<70%) and a slightly higher actual FDR than our proposed methods. In addition, the results from an analysis based on the qualitative characteristics of sample distribution for MAQC2 and human breast cancer datasets show that only our gene-wise normalization methods corrected data skewed towards lower read counts. However, when we evaluated MAQC3 with less variation in five replicates, all methods performed similarly. Thus, our proposed Med-pgQ2 and UQ-pgQ2 methods perform slightly better for differential gene analysis of RNA-seq data skewed towards lowly expressed read counts with high variation by improving specificity while maintaining a good detection power with a control of the nominal FDR level.
Conflict of interest statement
Figures
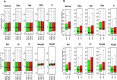
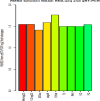
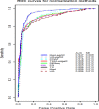
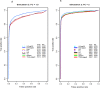
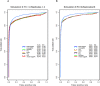
Similar articles
-
Choice of library size normalization and statistical methods for differential gene expression analysis in balanced two-group comparisons for RNA-seq studies.BMC Genomics. 2020 Jan 28;21(1):75. doi: 10.1186/s12864-020-6502-7. BMC Genomics. 2020. PMID: 31992223 Free PMC article.
-
Comparison of normalization and differential expression analyses using RNA-Seq data from 726 individual Drosophila melanogaster.BMC Genomics. 2016 Jan 5;17:28. doi: 10.1186/s12864-015-2353-z. BMC Genomics. 2016. PMID: 26732976 Free PMC article.
-
Comparing the normalization methods for the differential analysis of Illumina high-throughput RNA-Seq data.BMC Bioinformatics. 2015 Oct 28;16:347. doi: 10.1186/s12859-015-0778-7. BMC Bioinformatics. 2015. PMID: 26511205 Free PMC article.
-
Normalization for Single-Cell RNA-Seq Data Analysis.Methods Mol Biol. 2019;1935:11-23. doi: 10.1007/978-1-4939-9057-3_2. Methods Mol Biol. 2019. PMID: 30758817 Review.
-
Statistical detection of differentially expressed genes based on RNA-seq: from biological to phylogenetic replicates.Brief Bioinform. 2016 Mar;17(2):243-8. doi: 10.1093/bib/bbv035. Epub 2015 Jun 24. Brief Bioinform. 2016. PMID: 26108230 Review.
Cited by
-
Response to "Neglecting normalization impact in semi-synthetic RNA-seq data simulation generates artificial false positives" and "Winsorization greatly reduces false positives by popular differential expression methods when analyzing human population samples".Genome Biol. 2024 Oct 30;25(1):283. doi: 10.1186/s13059-024-03232-8. Genome Biol. 2024. PMID: 39478544 Free PMC article.
-
ToxDAR: A Workflow Software for Analyzing Toxicologically Relevant Proteomic and Transcriptomic Data, from Data Preparation to Toxicological Mechanism Elucidation.Int J Mol Sci. 2024 Sep 2;25(17):9544. doi: 10.3390/ijms25179544. Int J Mol Sci. 2024. PMID: 39273492 Free PMC article.
-
Normalization of RNA-Seq data using adaptive trimmed mean with multi-reference.Brief Bioinform. 2024 Mar 27;25(3):bbae241. doi: 10.1093/bib/bbae241. Brief Bioinform. 2024. PMID: 38770720 Free PMC article.
-
BEERS2: RNA-Seq simulation through high fidelity in silico modeling.Brief Bioinform. 2024 Mar 27;25(3):bbae164. doi: 10.1093/bib/bbae164. Brief Bioinform. 2024. PMID: 38605641 Free PMC article.
-
Standardizing digital biobanks: integrating imaging, genomic, and clinical data for precision medicine.J Transl Med. 2024 Feb 5;22(1):136. doi: 10.1186/s12967-024-04891-8. J Transl Med. 2024. PMID: 38317237 Free PMC article. Review.
References
-
- Wang ET, Sandberg R, Luo S, Khrebtukova I, Zhang L, Mayr C, et al. (2008) Alternative isoform regulation in human tissue transcriptomes. Nature 456: 470–476. doi: 10.1038/nature07509 - DOI - PMC - PubMed
-
- Pan Q, Shai O, Lee LJ, Frey BJ, Blencowe BJ (2008) Deep surveying of alternative splicing complexity in the human transcriptome by high-throughput sequencing. Nat Genet 40: 1413–1415. doi: 10.1038/ng.259 - DOI - PubMed
-
- Schliebner I, Becher R, Hempel M, Deising HB, Horbach R (2014) New gene models and alternative splicing in the maize pathogen Colletotrichum graminicola revealed by RNA-Seq analysis. BMC Genomics 15: 842 doi: 10.1186/1471-2164-15-842 - DOI - PMC - PubMed
-
- Robertson G, Schein J, Chiu R, Corbett R, Field M, Jackman SD, et al. (2010) De novo assembly and analysis of RNA-seq data. Nat Methods 7: 909–912. doi: 10.1038/nmeth.1517 - DOI - PubMed
-
- Schulz MH, Zerbino DR, Vingron M, Birney E (2012) Oases: robust de novo RNA-seq assembly across the dynamic range of expression levels. Bioinformatics 28: 1086–1092. doi: 10.1093/bioinformatics/bts094 - DOI - PMC - PubMed
Publication types
MeSH terms
Grants and funding
LinkOut - more resources
Full Text Sources
Other Literature Sources