Meta-analysis approach as a gene selection method in class prediction: does it improve model performance? A case study in acute myeloid leukemia
- PMID: 28399794
- PMCID: PMC5387259
- DOI: 10.1186/s12859-017-1619-7
Meta-analysis approach as a gene selection method in class prediction: does it improve model performance? A case study in acute myeloid leukemia
Abstract
Background: Aggregating gene expression data across experiments via meta-analysis is expected to increase the precision of the effect estimates and to increase the statistical power to detect a certain fold change. This study evaluates the potential benefit of using a meta-analysis approach as a gene selection method prior to predictive modeling in gene expression data.
Results: Six raw datasets from different gene expression experiments in acute myeloid leukemia (AML) and 11 different classification methods were used to build classification models to classify samples as either AML or healthy control. First, the classification models were trained on gene expression data from single experiments using conventional supervised variable selection and externally validated with the other five gene expression datasets (referred to as the individual-classification approach). Next, gene selection was performed through meta-analysis on four datasets, and predictive models were trained with the selected genes on the fifth dataset and validated on the sixth dataset. For some datasets, gene selection through meta-analysis helped classification models to achieve higher performance as compared to predictive modeling based on a single dataset; but for others, there was no major improvement. Synthetic datasets were generated from nine simulation scenarios. The effect of sample size, fold change and pairwise correlation between differentially expressed (DE) genes on the difference between MA- and individual-classification model was evaluated. The fold change and pairwise correlation significantly contributed to the difference in performance between the two methods. The gene selection via meta-analysis approach was more effective when it was conducted using a set of data with low fold change and high pairwise correlation on the DE genes.
Conclusion: Gene selection through meta-analysis on previously published studies potentially improves the performance of a predictive model on a given gene expression data.
Keywords: Acute myeloid leukemia; Gene expression; Meta-analysis; Predictive modeling.
Figures
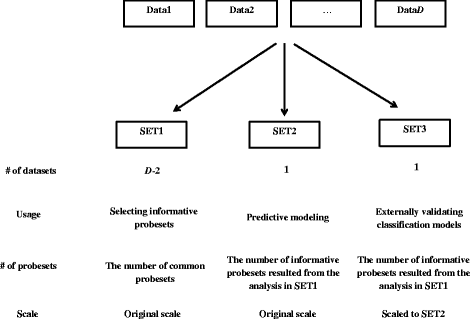
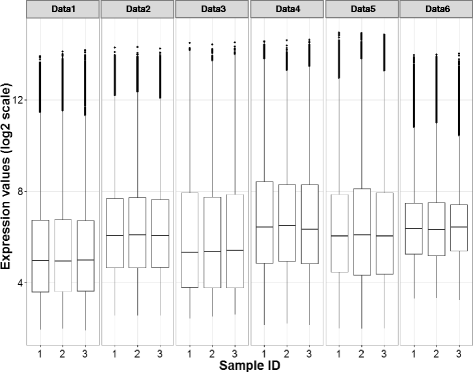
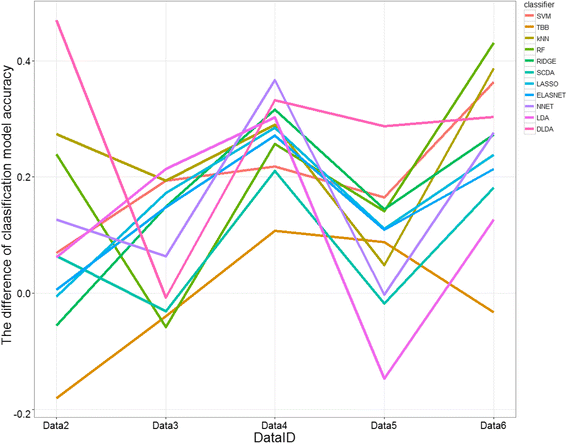
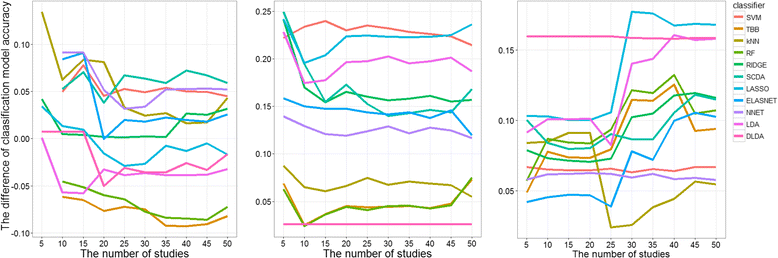
Similar articles
-
Statistical Test of Expression Pattern (STEPath): a new strategy to integrate gene expression data with genomic information in individual and meta-analysis studies.BMC Bioinformatics. 2011 Apr 11;12:92. doi: 10.1186/1471-2105-12-92. BMC Bioinformatics. 2011. PMID: 21481242 Free PMC article.
-
Folic acid supplementation and malaria susceptibility and severity among people taking antifolate antimalarial drugs in endemic areas.Cochrane Database Syst Rev. 2022 Feb 1;2(2022):CD014217. doi: 10.1002/14651858.CD014217. Cochrane Database Syst Rev. 2022. PMID: 36321557 Free PMC article.
-
Factors affecting the accuracy of a class prediction model in gene expression data.BMC Bioinformatics. 2015 Jun 21;16:199. doi: 10.1186/s12859-015-0610-4. BMC Bioinformatics. 2015. PMID: 26093633 Free PMC article.
-
Stratification of acute myeloid leukemia based on gene expression profiles.Int J Hematol. 2004 Dec;80(5):389-94. doi: 10.1532/ijh97.04111. Int J Hematol. 2004. PMID: 15646648 Review.
-
Gene expression profiling in acute myeloid leukaemia (AML).Best Pract Res Clin Haematol. 2009 Jun;22(2):169-80. doi: 10.1016/j.beha.2009.04.003. Best Pract Res Clin Haematol. 2009. PMID: 19698926 Review.
Cited by
-
Robustification of Naïve Bayes Classifier and Its Application for Microarray Gene Expression Data Analysis.Biomed Res Int. 2017;2017:3020627. doi: 10.1155/2017/3020627. Epub 2017 Aug 7. Biomed Res Int. 2017. PMID: 28848763 Free PMC article.
-
The importance of genomic predictors for clinical outcome of hematological malignancies.Blood Sci. 2021 Jul 7;3(3):93-95. doi: 10.1097/BS9.0000000000000075. eCollection 2021 Jul. Blood Sci. 2021. PMID: 35402837 Free PMC article. No abstract available.
-
High-Throughput Omics and Statistical Learning Integration for the Discovery and Validation of Novel Diagnostic Signatures in Colorectal Cancer.Int J Mol Sci. 2019 Jan 12;20(2):296. doi: 10.3390/ijms20020296. Int J Mol Sci. 2019. PMID: 30642095 Free PMC article.
References
MeSH terms
LinkOut - more resources
Full Text Sources
Other Literature Sources
Medical