Synergistic drug combinations for cancer identified in a CRISPR screen for pairwise genetic interactions
- PMID: 28319085
- PMCID: PMC5557292
- DOI: 10.1038/nbt.3834
Synergistic drug combinations for cancer identified in a CRISPR screen for pairwise genetic interactions
Abstract
Identification of effective combination therapies is critical to address the emergence of drug-resistant cancers, but direct screening of all possible drug combinations is infeasible. Here we introduce a CRISPR-based double knockout (CDKO) system that improves the efficiency of combinatorial genetic screening using an effective strategy for cloning and sequencing paired single guide RNA (sgRNA) libraries and a robust statistical scoring method for calculating genetic interactions (GIs) from CRISPR-deleted gene pairs. We applied CDKO to generate a large-scale human GI map, comprising 490,000 double-sgRNAs directed against 21,321 pairs of drug targets in K562 leukemia cells and identified synthetic lethal drug target pairs for which corresponding drugs exhibit synergistic killing. These included the BCL2L1 and MCL1 combination, which was also effective in imatinib-resistant cells. We further validated this system by identifying known and previously unidentified GIs between modifiers of ricin toxicity. This work provides an effective strategy to screen synergistic drug combinations in high-throughput and a CRISPR-based tool to dissect functional GI networks.
Conflict of interest statement
The authors declare no competing financial interest.
Figures
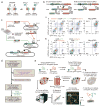
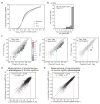
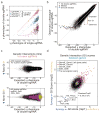
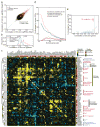
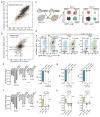
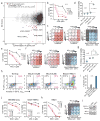
Comment in
-
Genetic screens: CRISPR-based mapping of genetic interactions.Nat Rev Genet. 2017 May;18(5):272-273. doi: 10.1038/nrg.2017.25. Epub 2017 Apr 3. Nat Rev Genet. 2017. PMID: 28366937 No abstract available.
-
Cancer: Identifying synergistic drug combinations.Nat Rev Drug Discov. 2017 May;16(5):314. doi: 10.1038/nrd.2017.76. Epub 2017 Apr 18. Nat Rev Drug Discov. 2017. PMID: 28417987 No abstract available.
Similar articles
-
Combinatorial CRISPR-Cas9 screens for de novo mapping of genetic interactions.Nat Methods. 2017 Jun;14(6):573-576. doi: 10.1038/nmeth.4225. Epub 2017 Mar 20. Nat Methods. 2017. PMID: 28319113 Free PMC article.
-
Minimized combinatorial CRISPR screens identify genetic interactions in autophagy.Nucleic Acids Res. 2021 Jun 4;49(10):5684-5704. doi: 10.1093/nar/gkab309. Nucleic Acids Res. 2021. PMID: 33956155 Free PMC article.
-
Multiplexed barcoded CRISPR-Cas9 screening enabled by CombiGEM.Proc Natl Acad Sci U S A. 2016 Mar 1;113(9):2544-9. doi: 10.1073/pnas.1517883113. Epub 2016 Feb 10. Proc Natl Acad Sci U S A. 2016. PMID: 26864203 Free PMC article.
-
A Perspective on the Future of High-Throughput RNAi Screening: Will CRISPR Cut Out the Competition or Can RNAi Help Guide the Way?J Biomol Screen. 2015 Sep;20(8):1040-51. doi: 10.1177/1087057115590069. Epub 2015 Jun 5. J Biomol Screen. 2015. PMID: 26048892 Review.
-
CRISPR-Cas System Is an Effective Tool for Identifying Drug Combinations That Provide Synergistic Therapeutic Potential in Cancers.Cells. 2023 Nov 9;12(22):2593. doi: 10.3390/cells12222593. Cells. 2023. PMID: 37998328 Free PMC article. Review.
Cited by
-
A genome-wide atlas of co-essential modules assigns function to uncharacterized genes.Nat Genet. 2021 May;53(5):638-649. doi: 10.1038/s41588-021-00840-z. Epub 2021 Apr 15. Nat Genet. 2021. PMID: 33859415 Free PMC article.
-
Characterization of cancer omics and drug perturbations in panels of lung cancer cells.Sci Rep. 2019 Dec 20;9(1):19529. doi: 10.1038/s41598-019-55692-9. Sci Rep. 2019. PMID: 31863083 Free PMC article.
-
A case of mistaken identity.Nat Biotechnol. 2018 Sep 6;36(9):802-804. doi: 10.1038/nbt.4208. Nat Biotechnol. 2018. PMID: 30188534 No abstract available.
-
Gene editing and its applications in biomedicine.Sci China Life Sci. 2022 Apr;65(4):660-700. doi: 10.1007/s11427-021-2057-0. Epub 2022 Feb 18. Sci China Life Sci. 2022. PMID: 35235150 Free PMC article. Review.
-
CRISPR in cancer biology and therapy.Nat Rev Cancer. 2022 May;22(5):259-279. doi: 10.1038/s41568-022-00441-w. Epub 2022 Feb 22. Nat Rev Cancer. 2022. PMID: 35194172 Review.
References
-
- Jia J, et al. Mechanisms of drug combinations: interaction and network perspectives. Nat Rev Drug Discov. 2009;8:111. - PubMed
-
- Ashburn TT, Thor KB. Drug repositioning: identifying and developing new uses for existing drugs. Nat Rev Drug Discov. 2004;3:673–683. - PubMed
-
- Al-Lazikani B, Banerji U, Workman P. Combinatorial drug therapy for cancer in the post-genomic era. Nat Biotechnol. 2012;30:679–692. - PubMed
-
- Sun X, Vilar S, Tatonetti NP. High-Throughput Methods for Combinatorial Drug Discovery. Sci Transl Med. 2013;5:205rv1–205rv1. - PubMed
Publication types
MeSH terms
Substances
Grants and funding
LinkOut - more resources
Full Text Sources
Other Literature Sources
Research Materials