A Bayesian spatio-temporal model for forecasting the prevalence of antibodies to Ehrlichia species in domestic dogs within the contiguous United States
- PMID: 28274248
- PMCID: PMC5343545
- DOI: 10.1186/s13071-017-2068-x
A Bayesian spatio-temporal model for forecasting the prevalence of antibodies to Ehrlichia species in domestic dogs within the contiguous United States
Abstract
Background: Dogs in the United States are hosts to a diverse range of vector-borne pathogens, several of which are important zoonoses. This paper describes factors deemed to be significantly related to the prevalence of antibodies to Ehrlichia spp. in domestic dogs, including climatic conditions, geographical factors, and societal factors. These factors are used in concert with a spatio-temporal model to construct an annual seroprevalence forecast. The proposed method of forecasting and an assessment of its fidelity are described.
Methods: Approximately twelve million serological test results for canine exposure to Ehrlichia spp. were used in the development of a Bayesian approach to forecast canine infection. Data used were collected on the county level across the contiguous United States from routine veterinary diagnostic tests between 2011-2015. Maps depicting the spatial baseline Ehrlichia spp. prevalence were constructed using Kriging and head-banging smoothing methods. Data were statistically analyzed to identify factors related to antibody prevalence via a Bayesian spatio-temporal conditional autoregressive (CAR) model. Finally, a forecast of future Ehrlichia seroprevalence was constructed based on the proposed model using county-level data on five predictive factors identified at a workshop hosted by the Companion Animal Parasite Council and published in 2014: annual temperature, percentage forest coverage, percentage surface water coverage, population density and median household income. Data were statistically analyzed to identify factors related to disease prevalence via a Bayesian spatio-temporal model. The fitted model and factor extrapolations were then used to forecast the regional seroprevalence for 2016.
Results: The correlation between the observed and model-estimated county-by-county Ehrlichia seroprevalence for the five-year period 2011-2015 is 0.842, demonstrating reasonable model accuracy. The weighted correlation (acknowledging unequal sample sizes) between 2015 observed and forecasted county-by-county Ehrlichia seroprevalence is 0.970, demonstrating that Ehrlichia seroprevalence can be forecasted accurately.
Conclusions: The forecast presented herein can be an a priori alert to veterinarians regarding areas expected to see expansion of Ehrlichia beyond the accepted endemic range, or in some regions a dynamic change from historical average prevalence. Moreover, this forecast could potentially serve as a surveillance tool for human health and prove useful for forecasting other vector-borne diseases.
Keywords: Autoregression; CAR Model; Ehrlichiosis; Head-banging; Kriging; Prevalence; Spatio-temporal modeling.
Figures
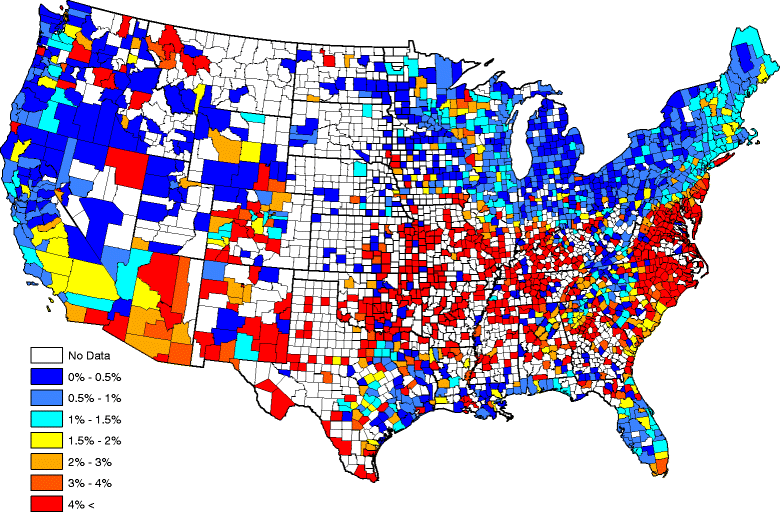
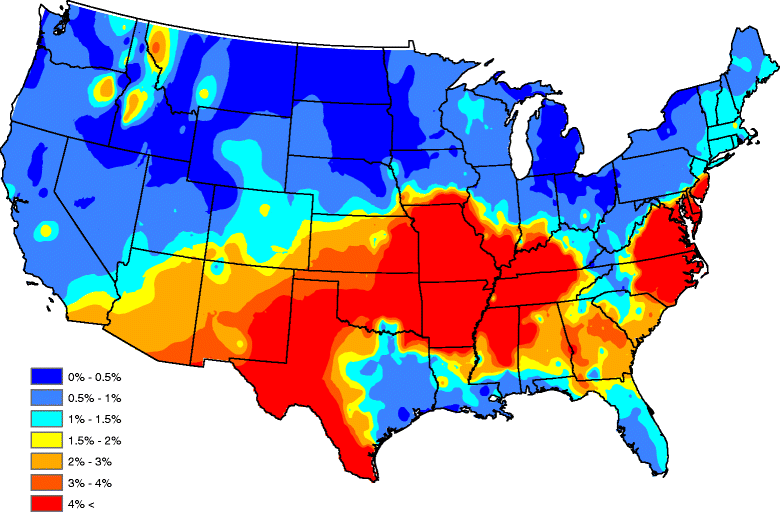
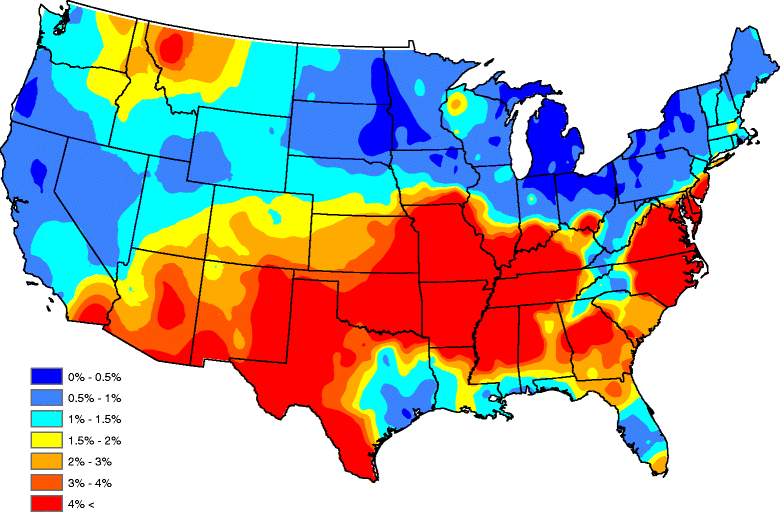
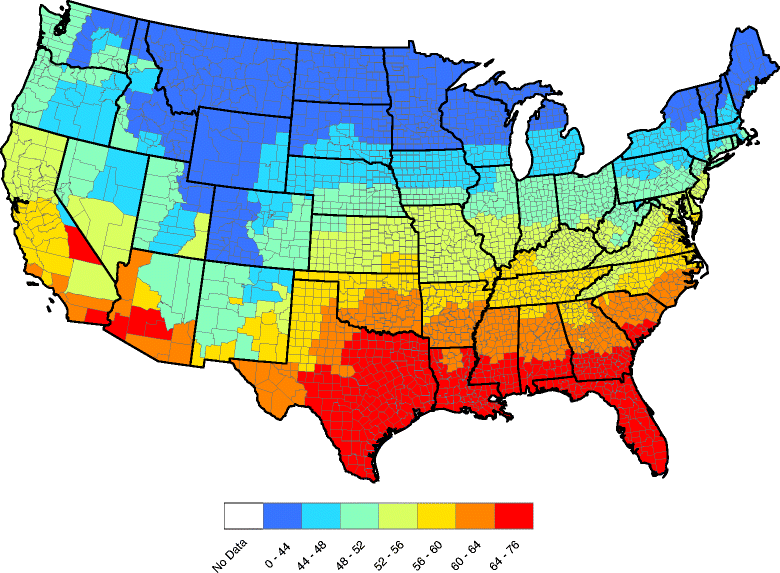
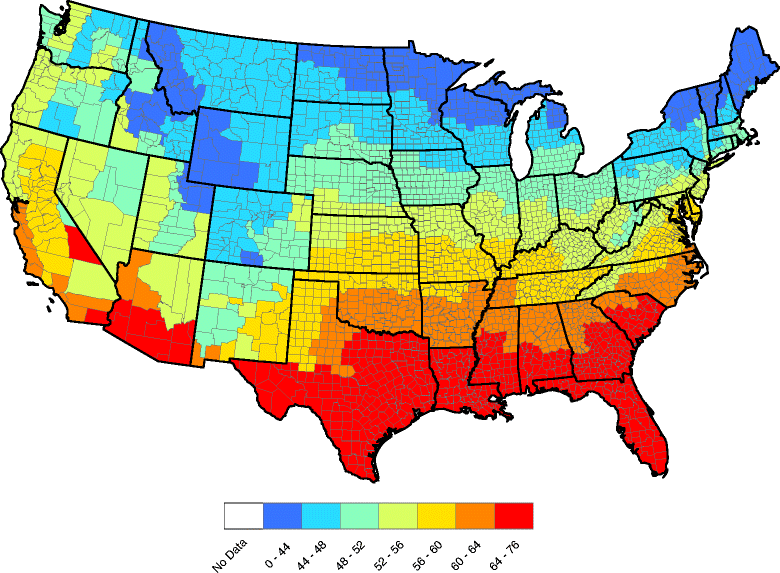
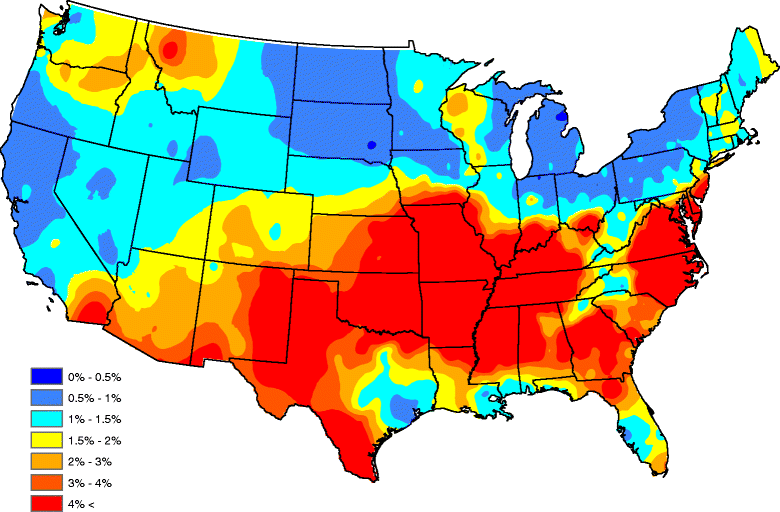
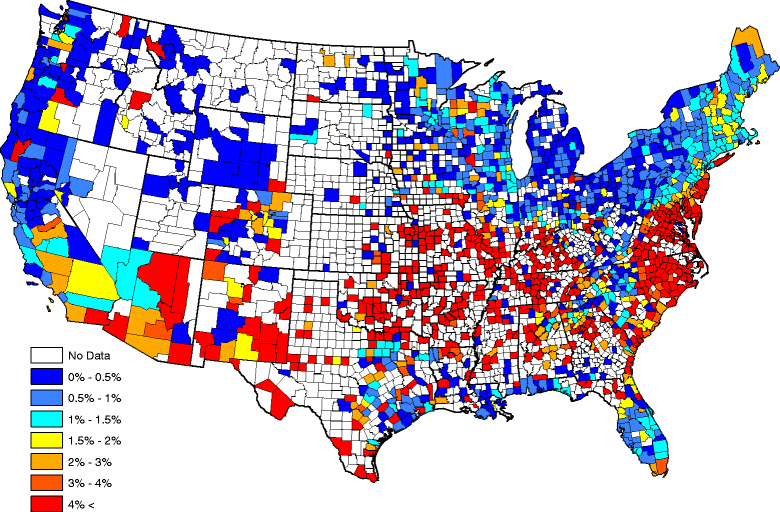
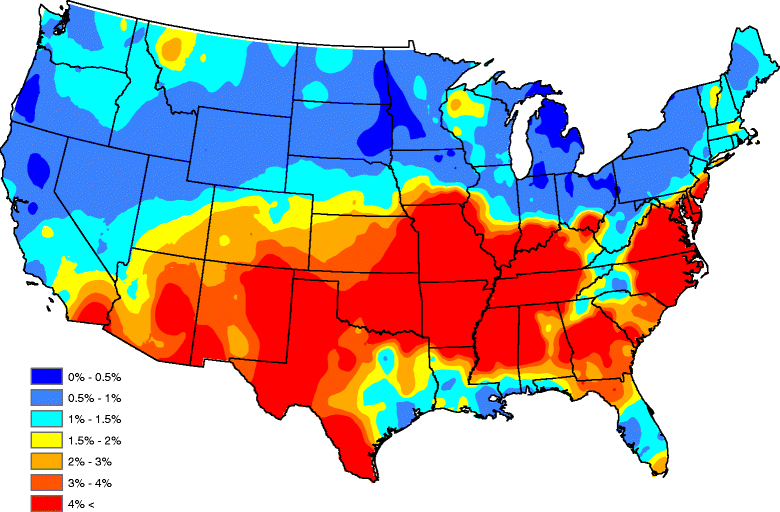
Similar articles
-
A Bayesian spatio-temporal model for forecasting the prevalence of antibodies to Borrelia burgdorferi, causative agent of Lyme disease, in domestic dogs within the contiguous United States.PLoS One. 2017 May 4;12(5):e0174428. doi: 10.1371/journal.pone.0174428. eCollection 2017. PLoS One. 2017. PMID: 28472096 Free PMC article.
-
A Bayesian spatio-temporal model for forecasting Anaplasma species seroprevalence in domestic dogs within the contiguous United States.PLoS One. 2017 Jul 24;12(7):e0182028. doi: 10.1371/journal.pone.0182028. eCollection 2017. PLoS One. 2017. PMID: 28738085 Free PMC article.
-
Forecasting United States heartworm Dirofilaria immitis prevalence in dogs.Parasit Vectors. 2016 Oct 10;9(1):540. doi: 10.1186/s13071-016-1804-y. Parasit Vectors. 2016. PMID: 27724981 Free PMC article.
-
Canine vector-borne disease: mapping and the accuracy of forecasting using big data from the veterinary community.Anim Health Res Rev. 2019 Jun;20(1):47-60. doi: 10.1017/S1466252319000045. Epub 2019 Sep 26. Anim Health Res Rev. 2019. PMID: 31895020 Review.
-
Guideline for veterinary practitioners on canine ehrlichiosis and anaplasmosis in Europe.Parasit Vectors. 2015 Feb 4;8:75. doi: 10.1186/s13071-015-0649-0. Parasit Vectors. 2015. PMID: 25649069 Free PMC article. Review.
Cited by
-
A Bayesian spatio-temporal model for forecasting the prevalence of antibodies to Borrelia burgdorferi, causative agent of Lyme disease, in domestic dogs within the contiguous United States.PLoS One. 2017 May 4;12(5):e0174428. doi: 10.1371/journal.pone.0174428. eCollection 2017. PLoS One. 2017. PMID: 28472096 Free PMC article.
-
Canine leptospirosis: A One Health approach for improved surveillance, prevention, and interdisciplinary collaboration.Can Vet J. 2024 Jun;65(6):609-612. Can Vet J. 2024. PMID: 38827602 Free PMC article. No abstract available.
-
Ecological and Socioeconomic Factors Associated with Bartonella henselae Exposure in Dogs Tested for Vector-Borne Diseases in North Carolina.Vector Borne Zoonotic Dis. 2019 Aug;19(8):582-595. doi: 10.1089/vbz.2018.2397. Epub 2019 May 21. Vector Borne Zoonotic Dis. 2019. PMID: 31112095 Free PMC article.
-
Accessibility to rabies centers and human rabies post-exposure prophylaxis rates in Cambodia: A Bayesian spatio-temporal analysis to identify optimal locations for future centers.PLoS Negl Trop Dis. 2022 Jun 30;16(6):e0010494. doi: 10.1371/journal.pntd.0010494. eCollection 2022 Jun. PLoS Negl Trop Dis. 2022. PMID: 35771752 Free PMC article.
-
Large-scale health disparities associated with Lyme disease and human monocytic ehrlichiosis in the United States, 2007-2013.PLoS One. 2018 Sep 27;13(9):e0204609. doi: 10.1371/journal.pone.0204609. eCollection 2018. PLoS One. 2018. PMID: 30261027 Free PMC article.
References
-
- Yabsley MJ, Dugan VG, Stallknecht DE, Little SE, Lockhart JM, Dawson JE, Davidson WR. Evaluation of a prototype Ehrlichia chaffeensis surveillance system using white-tailed deer (Odocoileus virginianus) as natural sentinels. Vector Borne Zoonotic Dis. 2003;3(4):195–207. doi: 10.1089/153036603322662183. - DOI - PubMed
MeSH terms
LinkOut - more resources
Full Text Sources
Other Literature Sources
Miscellaneous