Positive-Unlabeled Learning for inferring drug interactions based on heterogeneous attributes
- PMID: 28249566
- PMCID: PMC5333429
- DOI: 10.1186/s12859-017-1546-7
Positive-Unlabeled Learning for inferring drug interactions based on heterogeneous attributes
Abstract
Background: Investigating and understanding drug-drug interactions (DDIs) is important in improving the effectiveness of clinical care. DDIs can occur when two or more drugs are administered together. Experimentally based DDI detection methods require a large cost and time. Hence, there is a great interest in developing efficient and useful computational methods for inferring potential DDIs. Standard binary classifiers require both positives and negatives for training. In a DDI context, drug pairs that are known to interact can serve as positives for predictive methods. But, the negatives or drug pairs that have been confirmed to have no interaction are scarce. To address this lack of negatives, we introduce a Positive-Unlabeled Learning method for inferring potential DDIs.
Results: The proposed method consists of three steps: i) application of Growing Self Organizing Maps to infer negatives from the unlabeled dataset; ii) using a pairwise similarity function to quantify the overlap between individual features of drugs and iii) using support vector machine classifier for inferring DDIs. We obtained 6036 DDIs from DrugBank database. Using the proposed approach, we inferred 589 drug pairs that are likely to not interact with each other; these drug pairs are used as representative data for the negative class in binary classification for DDI prediction. Moreover, we classify the predicted DDIs as Cytochrome P450 (CYP) enzyme-Dependent and CYP-Independent interactions invoking their locations on the Growing Self Organizing Map, due to the particular importance of these enzymes in clinically significant interaction effects. Further, we provide a case study on three predicted CYP-Dependent DDIs to evaluate the clinical relevance of this study.
Conclusion: Our proposed approach showed an absolute improvement in F1-score of 14 and 38% in comparison to the method that randomly selects unlabeled data points as likely negatives, depending on the choice of similarity function. We inferred 5300 possible CYP-Dependent DDIs and 592 CYP-Independent DDIs with the highest posterior probabilities. Our discoveries can be used to improve clinical care as well as the research outcomes of drug development.
Keywords: CYP isoforms; Drug-drug interaction; Growing self organizing map (GSOM); PU learning; Pairwise drug similarity.
Figures
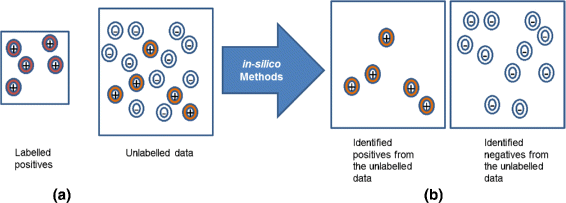
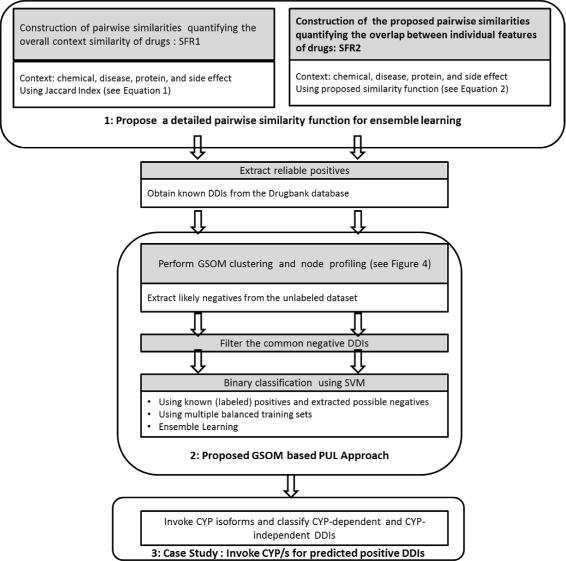
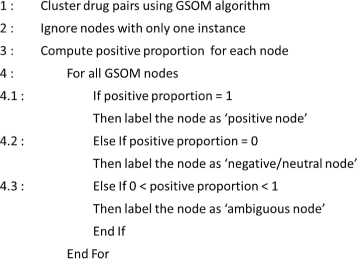
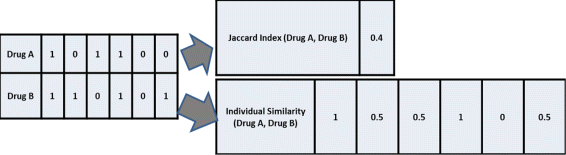
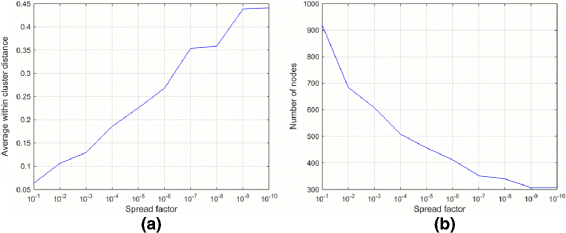
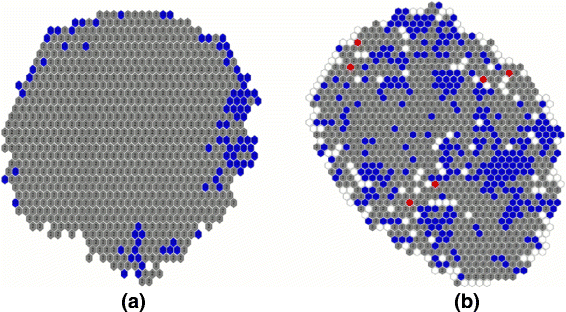
Similar articles
-
DDI-PULearn: a positive-unlabeled learning method for large-scale prediction of drug-drug interactions.BMC Bioinformatics. 2019 Dec 24;20(Suppl 19):661. doi: 10.1186/s12859-019-3214-6. BMC Bioinformatics. 2019. PMID: 31870276 Free PMC article.
-
Dynamic and Static Simulations of Fluvoxamine-Perpetrated Drug-Drug Interactions Using Multiple Cytochrome P450 Inhibition Modeling, and Determination of Perpetrator-Specific CYP Isoform Inhibition Constants and Fractional CYP Isoform Contributions to Victim Clearance.J Pharm Sci. 2016 Mar;105(3):1307-17. doi: 10.1016/j.xphs.2015.11.044. Epub 2016 Jan 30. J Pharm Sci. 2016. PMID: 26886336
-
Machine learning-based prediction of drug-drug interactions by integrating drug phenotypic, therapeutic, chemical, and genomic properties.J Am Med Inform Assoc. 2014 Oct;21(e2):e278-86. doi: 10.1136/amiajnl-2013-002512. Epub 2014 Mar 18. J Am Med Inform Assoc. 2014. PMID: 24644270 Free PMC article.
-
Prediction of drug-drug interaction potential using physiologically based pharmacokinetic modeling.Arch Pharm Res. 2017 Dec;40(12):1356-1379. doi: 10.1007/s12272-017-0976-0. Epub 2017 Oct 27. Arch Pharm Res. 2017. PMID: 29079968 Review.
-
Mini-series: I. Basic science. Uncertainty and inaccuracy of predicting CYP-mediated in vivo drug interactions in the ICU from in vitro models: focus on CYP3A4.Intensive Care Med. 2009 Mar;35(3):417-29. doi: 10.1007/s00134-008-1384-1. Epub 2009 Jan 9. Intensive Care Med. 2009. PMID: 19132343 Review.
Cited by
-
Evaluation of knowledge graph embedding approaches for drug-drug interaction prediction in realistic settings.BMC Bioinformatics. 2019 Dec 18;20(1):726. doi: 10.1186/s12859-019-3284-5. BMC Bioinformatics. 2019. PMID: 31852427 Free PMC article.
-
Positive-unlabelled learning of glycosylation sites in the human proteome.BMC Bioinformatics. 2019 Mar 6;20(1):112. doi: 10.1186/s12859-019-2700-1. BMC Bioinformatics. 2019. PMID: 30841845 Free PMC article.
-
Drug-drug interaction prediction: databases, web servers and computational models.Brief Bioinform. 2023 Nov 22;25(1):bbad445. doi: 10.1093/bib/bbad445. Brief Bioinform. 2023. PMID: 38113076 Free PMC article. Review.
-
Twenty years of bioinformatics research for protease-specific substrate and cleavage site prediction: a comprehensive revisit and benchmarking of existing methods.Brief Bioinform. 2019 Nov 27;20(6):2150-2166. doi: 10.1093/bib/bby077. Brief Bioinform. 2019. PMID: 30184176 Free PMC article. Review.
-
Learning peptide properties with positive examples only.Digit Discov. 2024 Apr 19;3(5):977-986. doi: 10.1039/d3dd00218g. eCollection 2024 May 15. Digit Discov. 2024. PMID: 38756224 Free PMC article.
References
-
- Snyder BD, Polasek TM, Doogue MP. Drug interactions: principles and practice. Aust Prescr. 2012;35(3):85–8. doi: 10.18773/austprescr.2012.037. - DOI
-
- DrugBank. DrugBank Stat. http://www.drugbank.ca/stats. Accessed 31 Mar 2016.
MeSH terms
Substances
LinkOut - more resources
Full Text Sources
Other Literature Sources
Medical