Bioinformatics and Drug Discovery
- PMID: 27848897
- PMCID: PMC5421137
- DOI: 10.2174/1568026617666161116143440
Bioinformatics and Drug Discovery
Abstract
Bioinformatic analysis can not only accelerate drug target identification and drug candidate screening and refinement, but also facilitate characterization of side effects and predict drug resistance. High-throughput data such as genomic, epigenetic, genome architecture, cistromic, transcriptomic, proteomic, and ribosome profiling data have all made significant contribution to mechanismbased drug discovery and drug repurposing. Accumulation of protein and RNA structures, as well as development of homology modeling and protein structure simulation, coupled with large structure databases of small molecules and metabolites, paved the way for more realistic protein-ligand docking experiments and more informative virtual screening. I present the conceptual framework that drives the collection of these high-throughput data, summarize the utility and potential of mining these data in drug discovery, outline a few inherent limitations in data and software mining these data, point out news ways to refine analysis of these diverse types of data, and highlight commonly used software and databases relevant to drug discovery.
Keywords: Drug candidate; Drug screening; Drug target; Epigenetics; Genomics; Proteomics; Structure; Transcriptomics.
Copyright© Bentham Science Publishers; For any queries, please email at epub@benthamscience.org.
Figures
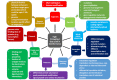
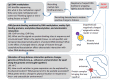
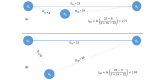
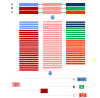
Similar articles
-
Advances in Drug Discovery based on Genomics, Proteomics and Bioinformatics in Malaria.Curr Top Med Chem. 2023;23(7):551-578. doi: 10.2174/1568026623666230418114455. Curr Top Med Chem. 2023. PMID: 37073654 Review.
-
Transcriptomic Data Mining and Repurposing for Computational Drug Discovery.Methods Mol Biol. 2019;1903:73-95. doi: 10.1007/978-1-4939-8955-3_5. Methods Mol Biol. 2019. PMID: 30547437
-
Bioinformatics Approaches for Anti-cancer Drug Discovery.Curr Drug Targets. 2020;21(1):3-17. doi: 10.2174/1389450120666190923162203. Curr Drug Targets. 2020. PMID: 31549592 Review.
-
Informatics resources for tuberculosis--towards drug discovery.Tuberculosis (Edinb). 2012 Mar;92(2):133-8. doi: 10.1016/j.tube.2011.08.006. Epub 2011 Sep 22. Tuberculosis (Edinb). 2012. PMID: 21943870 Review.
-
Established and emerging trends in computational drug discovery in the structural genomics era.Chem Biol. 2012 Jan 27;19(1):29-41. doi: 10.1016/j.chembiol.2011.12.007. Chem Biol. 2012. PMID: 22284352 Review.
Cited by
-
Application of Computational Biology and Artificial Intelligence in Drug Design.Int J Mol Sci. 2022 Nov 5;23(21):13568. doi: 10.3390/ijms232113568. Int J Mol Sci. 2022. PMID: 36362355 Free PMC article. Review.
-
Expression profile analysis identifies IER3 to predict overall survival and promote lymph node metastasis in tongue cancer.Cancer Cell Int. 2019 Nov 21;19:307. doi: 10.1186/s12935-019-1028-2. eCollection 2019. Cancer Cell Int. 2019. PMID: 31832020 Free PMC article.
-
Network pharmacology and molecular docking-based investigations of Kochiae Fructus's active phytomolecules, molecular targets, and pathways in treating COVID-19.Front Microbiol. 2022 Aug 5;13:972576. doi: 10.3389/fmicb.2022.972576. eCollection 2022. Front Microbiol. 2022. PMID: 35992697 Free PMC article.
-
In silico approaches supporting drug repurposing for Leishmaniasis: a scoping review.EXCLI J. 2024 Sep 3;23:1117-1169. doi: 10.17179/excli2024-7552. eCollection 2024. EXCLI J. 2024. PMID: 39421030 Free PMC article. Review.
-
Combined Multiomics and In Silico Approach Uncovers PRKAR1A as a Putative Therapeutic Target in Multi-Organ Dysfunction Syndrome.ACS Omega. 2023 Mar 1;8(10):9555-9568. doi: 10.1021/acsomega.3c00020. eCollection 2023 Mar 14. ACS Omega. 2023. PMID: 36936296 Free PMC article.
References
-
- David E., Tramontin T., Zemmel R., Pharmaceutical R. D: the road to positive returns. Nat. Rev. Drug Discov. 2009;8:609–610. - PubMed
-
- Drews J., Ryser S. The role of innovation in drug development. Nat. Biotechnol. 1997;15:1318–1319. - PubMed
-
- Boxall A.B., Rudd M.A., Brooks B.W., Caldwell D.J., Choi K., Hickmann S., Innes E., Ostapyk K., Staveley J.P., Verslycke T., Ankley G.T., Beazley K.F., Belanger S.E., Berninger J.P., Carriquiriborde P., Coors A., Deleo P.C., Dyer S.D., Ericson J.F., Gagne F., Giesy J.P., Gouin T., Hallstrom L., Karlsson M.V., Larsson D.G., Lazorchak J.M., Mastrocco F., McLaughlin A., McMaster M.E., Meyerhoff R.D., Moore R., Parrott J.L., Snape J.R., Murray-Smith R., Servos M.R., Sibley P.K., Straub J.O., Szabo N.D., Topp E., Tetreault G.R., Trudeau V.L., Van Der Kraak G. Pharmaceuticals and personal care products in the environment: what are the big questions? Environ. Health Perspect. 2012;120:1221–1219. - PMC - PubMed
-
- Doolittle R.F., Hunkapiller M.W., Hood L.E., Devare S.G., Robbins K.C., Aaronson S.A., Antoniades H.N. Simian sarcoma virus onc gene, v-sis, is derived from the gene (or genes) encoding a platelet-derived growth factor. Science. 1983;221:275–277. - PubMed
Publication types
MeSH terms
LinkOut - more resources
Full Text Sources
Other Literature Sources