Tumor reference resolution and characteristic extraction in radiology reports for liver cancer stage prediction
- PMID: 27729234
- PMCID: PMC5136527
- DOI: 10.1016/j.jbi.2016.10.005
Tumor reference resolution and characteristic extraction in radiology reports for liver cancer stage prediction
Abstract
Background: Anaphoric references occur ubiquitously in clinical narrative text. However, the problem, still very much an open challenge, is typically less aggressively focused on in clinical text domain applications. Furthermore, existing research on reference resolution is often conducted disjointly from real-world motivating tasks.
Objective: In this paper, we present our machine-learning system that automatically performs reference resolution and a rule-based system to extract tumor characteristics, with component-based and end-to-end evaluations. Specifically, our goal was to build an algorithm that takes in tumor templates and outputs tumor characteristic, e.g. tumor number and largest tumor sizes, necessary for identifying patient liver cancer stage phenotypes.
Results: Our reference resolution system reached a modest performance of 0.66 F1 for the averaged MUC, B-cubed, and CEAF scores for coreference resolution and 0.43 F1 for particularization relations. However, even this modest performance was helpful to increase the automatic tumor characteristics annotation substantially over no reference resolution.
Conclusion: Experiments revealed the benefit of reference resolution even for relatively simple tumor characteristics variables such as largest tumor size. However we found that different overall variables had different tolerances to reference resolution upstream errors, highlighting the need to characterize systems by end-to-end evaluations.
Keywords: Cancer stages; Information extraction; Liver cancer; Natural language processing; Radiology report; Reference resolution.
Copyright © 2016 Elsevier Inc. All rights reserved.
Figures
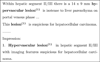
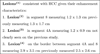
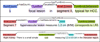
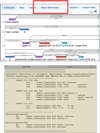
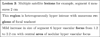

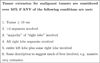

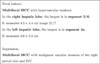
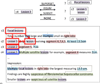
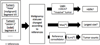
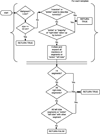
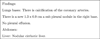
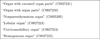
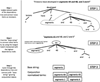
Similar articles
-
A categorical analysis of coreference resolution errors in biomedical texts.J Biomed Inform. 2016 Apr;60:309-18. doi: 10.1016/j.jbi.2016.02.015. Epub 2016 Feb 27. J Biomed Inform. 2016. PMID: 26925515
-
Using domain knowledge and domain-inspired discourse model for coreference resolution for clinical narratives.J Am Med Inform Assoc. 2013 Mar-Apr;20(2):356-62. doi: 10.1136/amiajnl-2011-000767. Epub 2012 Jul 10. J Am Med Inform Assoc. 2013. PMID: 22781192 Free PMC article.
-
Minimalistic Approach to Coreference Resolution in Lithuanian Medical Records.Comput Math Methods Med. 2019 Mar 20;2019:9079840. doi: 10.1155/2019/9079840. eCollection 2019. Comput Math Methods Med. 2019. PMID: 31015858 Free PMC article.
-
Natural Language Processing in Radiology: A Systematic Review.Radiology. 2016 May;279(2):329-43. doi: 10.1148/radiol.16142770. Radiology. 2016. PMID: 27089187 Review.
-
Natural Language Processing Technologies in Radiology Research and Clinical Applications.Radiographics. 2016 Jan-Feb;36(1):176-91. doi: 10.1148/rg.2016150080. Radiographics. 2016. PMID: 26761536 Free PMC article. Review.
Cited by
-
Ontologies for Liver Diseases Representation: A Systematic Literature Review.J Digit Imaging. 2020 Jun;33(3):563-573. doi: 10.1007/s10278-019-00303-2. J Digit Imaging. 2020. PMID: 31848894 Free PMC article. Review.
-
Ontology-Based Approach for Liver Cancer Diagnosis and Treatment.J Digit Imaging. 2019 Feb;32(1):116-130. doi: 10.1007/s10278-018-0115-6. J Digit Imaging. 2019. PMID: 30066122 Free PMC article.
-
Making Sense of Big Textual Data for Health Care: Findings from the Section on Clinical Natural Language Processing.Yearb Med Inform. 2017 Aug;26(1):228-234. doi: 10.15265/IY-2017-027. Epub 2017 Sep 11. Yearb Med Inform. 2017. PMID: 29063569 Free PMC article. Review.
-
Research and Application of Artificial Intelligence Based on Electronic Health Records of Patients With Cancer: Systematic Review.JMIR Med Inform. 2022 Apr 20;10(4):e33799. doi: 10.2196/33799. JMIR Med Inform. 2022. PMID: 35442195 Free PMC article. Review.
-
Low APOA-1 Expression in Hepatocellular Carcinoma Patients Is Associated With DNA Methylation and Poor Overall Survival.Front Genet. 2021 Nov 1;12:760744. doi: 10.3389/fgene.2021.760744. eCollection 2021. Front Genet. 2021. PMID: 34790226 Free PMC article.
References
-
- Grishman R, Sundheim B. Message understanding conference-6: A brief history. COLING. 1996;96:466–471.
-
- Doddington GR, Mitchell A, Przybocki MA, Ramshaw LA, Strassel S, Weischedel RM. The automatic content extraction (ace) program-tasks, data, and evaluation. LREC. 2004;2:1.
-
- OntoNotes Release 5.0 - Linguistic Data Consortium. URL https://catalog.ldc.upenn.edu/LDC2013T19.
-
- Araki J, Liu Z, Hovy E, Mitamura T. Detecting Subevent Structure for Event Coreference Resolution. URL http://citeseerx.ist.psu.edu/viewdoc/citations;jsessionid=AC6C5BDE654DDC....
-
- Kim J-D, Nguyen N, Wang Y, Tsujii J, Takagi T, Yonezawa A. The Genia Event and Protein Coreference tasks of the BioNLP Shared Task 2011. BMC Bioinformatics. 2012;13(11):1–12. URL http://dx.doi.org/10.1186/1471-2105-13-S11-S1. - DOI - PMC - PubMed
MeSH terms
Grants and funding
LinkOut - more resources
Full Text Sources
Other Literature Sources
Medical