Feature Subset Selection for Cancer Classification Using Weight Local Modularity
- PMID: 27703256
- PMCID: PMC5050509
- DOI: 10.1038/srep34759
Feature Subset Selection for Cancer Classification Using Weight Local Modularity
Abstract
Microarray is recently becoming an important tool for profiling the global gene expression patterns of tissues. Gene selection is a popular technology for cancer classification that aims to identify a small number of informative genes from thousands of genes that may contribute to the occurrence of cancers to obtain a high predictive accuracy. This technique has been extensively studied in recent years. This study develops a novel feature selection (FS) method for gene subset selection by utilizing the Weight Local Modularity (WLM) in a complex network, called the WLMGS. In the proposed method, the discriminative power of gene subset is evaluated by using the weight local modularity of a weighted sample graph in the gene subset where the intra-class distance is small and the inter-class distance is large. A higher local modularity of the gene subset corresponds to a greater discriminative of the gene subset. With the use of forward search strategy, a more informative gene subset as a group can be selected for the classification process. Computational experiments show that the proposed algorithm can select a small subset of the predictive gene as a group while preserving classification accuracy.
Figures
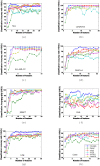
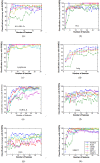
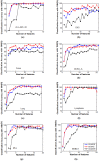
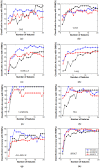
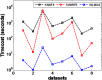
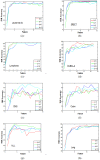
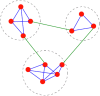
Similar articles
-
Simultaneous gene clustering and subset selection for sample classification via MDL.Bioinformatics. 2003 Jun 12;19(9):1100-9. doi: 10.1093/bioinformatics/btg039. Bioinformatics. 2003. PMID: 12801870
-
Ensemble gene selection by grouping for microarray data classification.J Biomed Inform. 2010 Feb;43(1):81-7. doi: 10.1016/j.jbi.2009.08.010. Epub 2009 Aug 20. J Biomed Inform. 2010. PMID: 19699316
-
Tumor classification ranking from microarray data.BMC Genomics. 2008 Sep 16;9 Suppl 2(Suppl 2):S21. doi: 10.1186/1471-2164-9-S2-S21. BMC Genomics. 2008. PMID: 18831787 Free PMC article.
-
Gene expression data analysis.FEBS Lett. 2000 Aug 25;480(1):17-24. doi: 10.1016/s0014-5793(00)01772-5. FEBS Lett. 2000. PMID: 10967323 Review.
-
Optimal gene expression analysis by microarrays.Cancer Cell. 2002 Nov;2(5):353-61. doi: 10.1016/s1535-6108(02)00181-2. Cancer Cell. 2002. PMID: 12450790 Review.
Cited by
-
Gene Correlation Guided Gene Selection for Microarray Data Classification.Biomed Res Int. 2021 Aug 14;2021:6490118. doi: 10.1155/2021/6490118. eCollection 2021. Biomed Res Int. 2021. PMID: 34435048 Free PMC article.
-
Cluster Analysis of Cell Nuclei in H&E-Stained Histological Sections of Prostate Cancer and Classification Based on Traditional and Modern Artificial Intelligence Techniques.Diagnostics (Basel). 2021 Dec 22;12(1):15. doi: 10.3390/diagnostics12010015. Diagnostics (Basel). 2021. PMID: 35054182 Free PMC article.
-
OmniGA: Optimized Omnivariate Decision Trees for Generalizable Classification Models.Sci Rep. 2017 Jun 20;7(1):3898. doi: 10.1038/s41598-017-04281-9. Sci Rep. 2017. PMID: 28634344 Free PMC article.
-
Gene selection for microarray data classification via subspace learning and manifold regularization.Med Biol Eng Comput. 2018 Jul;56(7):1271-1284. doi: 10.1007/s11517-017-1751-6. Epub 2017 Dec 19. Med Biol Eng Comput. 2018. PMID: 29256006
-
Neuropsychological predictors of conversion from mild cognitive impairment to Alzheimer's disease: a feature selection ensemble combining stability and predictability.BMC Med Inform Decis Mak. 2018 Dec 19;18(1):137. doi: 10.1186/s12911-018-0710-y. BMC Med Inform Decis Mak. 2018. PMID: 30567554 Free PMC article.
References
-
- José E. A., Garć ıa. N., Jourdan L. & Talbi E. G. Gene selection in cancer classification using PSO/SVM and GA/SVM hybrid algorithms. IEEE C. Evol. Computat. 9, 284–290 (2007).
-
- Derrac J., Cornelis C., García S. & Herrera F. Enhancing evolutionary instance selection algorithms by means of fuzzy rough set based feature selection. Information Sciences 186, 73–92 (2012).
-
- Sun X., Liu Y. H., Wei D. & Xu M. T. Selection of interdependent genes via dynamic relevance analysis for cancer diagnosis. J. Biomed. Inform. 46, 252–258 (2013). - PubMed
-
- Guyon I., Weston J., Barnhill S. & Vapnik V. Gene selection for cancer classification using suppor tvector machines. Mach. Learn. 46, 389–422 (2002).
-
- Saeys1 Y., Inza Iñ & Larrañaga P. A review of feature selection techniques in bioinformatics. Bioinformatics 23, 2507–2517 (2007). - PubMed
MeSH terms
LinkOut - more resources
Full Text Sources
Other Literature Sources