Omics-Based Strategies in Precision Medicine: Toward a Paradigm Shift in Inborn Errors of Metabolism Investigations
- PMID: 27649151
- PMCID: PMC5037827
- DOI: 10.3390/ijms17091555
Omics-Based Strategies in Precision Medicine: Toward a Paradigm Shift in Inborn Errors of Metabolism Investigations
Abstract
The rise of technologies that simultaneously measure thousands of data points represents the heart of systems biology. These technologies have had a huge impact on the discovery of next-generation diagnostics, biomarkers, and drugs in the precision medicine era. Systems biology aims to achieve systemic exploration of complex interactions in biological systems. Driven by high-throughput omics technologies and the computational surge, it enables multi-scale and insightful overviews of cells, organisms, and populations. Precision medicine capitalizes on these conceptual and technological advancements and stands on two main pillars: data generation and data modeling. High-throughput omics technologies allow the retrieval of comprehensive and holistic biological information, whereas computational capabilities enable high-dimensional data modeling and, therefore, accessible and user-friendly visualization. Furthermore, bioinformatics has enabled comprehensive multi-omics and clinical data integration for insightful interpretation. Despite their promise, the translation of these technologies into clinically actionable tools has been slow. In this review, we present state-of-the-art multi-omics data analysis strategies in a clinical context. The challenges of omics-based biomarker translation are discussed. Perspectives regarding the use of multi-omics approaches for inborn errors of metabolism (IEM) are presented by introducing a new paradigm shift in addressing IEM investigations in the post-genomic era.
Keywords: bioinformatics; biomarkers; chemometrics; data integration; inborn errors of metabolism; machine learning; mass spectrometry; next-generation sequencing; omics; precision medicine.
Conflict of interest statement
The authors declare no conflict of interest.
Figures
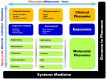
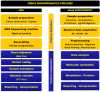
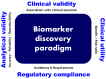
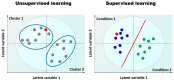
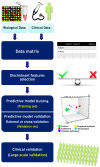
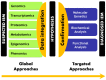
Similar articles
-
[The promise of omics in the precision medicine era].Rev Med Interne. 2022 Nov;43(11):649-660. doi: 10.1016/j.revmed.2022.07.009. Epub 2022 Aug 27. Rev Med Interne. 2022. PMID: 36041909 Review. French.
-
The diagnosis of inborn errors of metabolism by an integrative "multi-omics" approach: A perspective encompassing genomics, transcriptomics, and proteomics.J Inherit Metab Dis. 2020 Jan;43(1):25-35. doi: 10.1002/jimd.12130. Epub 2019 Jun 25. J Inherit Metab Dis. 2020. PMID: 31119744 Review.
-
The Need for Multi-Omics Biomarker Signatures in Precision Medicine.Int J Mol Sci. 2019 Sep 26;20(19):4781. doi: 10.3390/ijms20194781. Int J Mol Sci. 2019. PMID: 31561483 Free PMC article. Review.
-
Clinical Metabolomics: The New Metabolic Window for Inborn Errors of Metabolism Investigations in the Post-Genomic Era.Int J Mol Sci. 2016 Jul 20;17(7):1167. doi: 10.3390/ijms17071167. Int J Mol Sci. 2016. PMID: 27447622 Free PMC article. Review.
-
Integrative methods for analyzing big data in precision medicine.Proteomics. 2016 Mar;16(5):741-58. doi: 10.1002/pmic.201500396. Proteomics. 2016. PMID: 26677817 Review.
Cited by
-
Precision Medicine for Neonatal Sepsis.Front Mol Biosci. 2018 Jul 26;5:70. doi: 10.3389/fmolb.2018.00070. eCollection 2018. Front Mol Biosci. 2018. PMID: 30094238 Free PMC article. Review.
-
The current state of omics technologies in the clinical management of asthma and allergic diseases.Ann Allergy Asthma Immunol. 2019 Dec;123(6):550-557. doi: 10.1016/j.anai.2019.08.460. Epub 2019 Sep 5. Ann Allergy Asthma Immunol. 2019. PMID: 31494234 Free PMC article. Review.
-
Optimized Combination of Multiple Graphs With Application to the Integration of Brain Imaging and (epi)Genomics Data.IEEE Trans Med Imaging. 2020 Jun;39(6):1801-1811. doi: 10.1109/TMI.2019.2958256. Epub 2019 Dec 6. IEEE Trans Med Imaging. 2020. PMID: 31825864 Free PMC article.
-
Integration of Proteomics and Metabolomics in Exploring Genetic and Rare Metabolic Diseases.Kidney Dis (Basel). 2017 Jul;3(2):66-77. doi: 10.1159/000477493. Epub 2017 Jun 30. Kidney Dis (Basel). 2017. PMID: 28868294 Free PMC article. Review.
-
Research, diagnosis and education in inborn errors of metabolism in Colombia: 20 years' experience from a reference center.Orphanet J Rare Dis. 2018 Aug 16;13(1):141. doi: 10.1186/s13023-018-0879-2. Orphanet J Rare Dis. 2018. PMID: 30115094 Free PMC article. Review.
References
-
- Aon M.A. Systems Biology of Metabolic and Signaling Networks. Springer Berlin Heidelberg; Heidelberg, Germany: 2014. Complex systems biology of networks: The riddle and the challenge; pp. 19–35.
Publication types
MeSH terms
LinkOut - more resources
Full Text Sources
Other Literature Sources