Tools for Model Building and Optimization into Near-Atomic Resolution Electron Cryo-Microscopy Density Maps
- PMID: 27572730
- PMCID: PMC5103630
- DOI: 10.1016/bs.mie.2016.06.003
Tools for Model Building and Optimization into Near-Atomic Resolution Electron Cryo-Microscopy Density Maps
Abstract
Electron cryo-microscopy (cryoEM) has advanced dramatically to become a viable tool for high-resolution structural biology research. The ultimate outcome of a cryoEM study is an atomic model of a macromolecule or its complex with interacting partners. This chapter describes a variety of algorithms and software to build a de novo model based on the cryoEM 3D density map, to optimize the model with the best stereochemistry restraints and finally to validate the model with proper protocols. The full process of atomic structure determination from a cryoEM map is described. The tools outlined in this chapter should prove extremely valuable in revealing atomic interactions guided by cryoEM data.
Keywords: CryoEM map-derived model; Model optimization; Model validation.
© 2016 Elsevier Inc. All rights reserved.
Figures
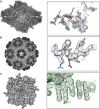
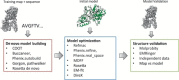
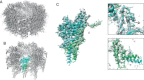
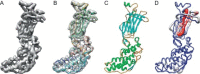
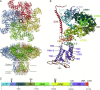
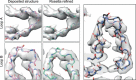
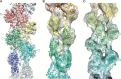
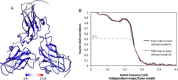
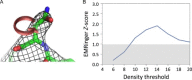
Similar articles
-
Testing the Validity of Single-Particle Maps at Low and High Resolution.Methods Enzymol. 2016;579:227-53. doi: 10.1016/bs.mie.2016.06.004. Epub 2016 Aug 8. Methods Enzymol. 2016. PMID: 27572729 Review.
-
Processing of Structurally Heterogeneous Cryo-EM Data in RELION.Methods Enzymol. 2016;579:125-57. doi: 10.1016/bs.mie.2016.04.012. Epub 2016 May 31. Methods Enzymol. 2016. PMID: 27572726 Review.
-
Refinement of Atomic Structures Against cryo-EM Maps.Methods Enzymol. 2016;579:277-305. doi: 10.1016/bs.mie.2016.05.033. Epub 2016 Jun 24. Methods Enzymol. 2016. PMID: 27572731 Review.
-
An atomic model of brome mosaic virus using direct electron detection and real-space optimization.Nat Commun. 2014 Sep 4;5:4808. doi: 10.1038/ncomms5808. Nat Commun. 2014. PMID: 25185801 Free PMC article.
-
cryoem-cloud-tools: A software platform to deploy and manage cryo-EM jobs in the cloud.J Struct Biol. 2018 Sep;203(3):230-235. doi: 10.1016/j.jsb.2018.05.014. Epub 2018 Jun 1. J Struct Biol. 2018. PMID: 29864529 Free PMC article.
Cited by
-
Accelerated cryo-EM-guided determination of three-dimensional RNA-only structures.Nat Methods. 2020 Jul;17(7):699-707. doi: 10.1038/s41592-020-0878-9. Epub 2020 Jul 2. Nat Methods. 2020. PMID: 32616928 Free PMC article.
-
Novel Artificial Intelligence-Based Approaches for Ab Initio Structure Determination and Atomic Model Building for Cryo-Electron Microscopy.Micromachines (Basel). 2023 Aug 27;14(9):1674. doi: 10.3390/mi14091674. Micromachines (Basel). 2023. PMID: 37763837 Free PMC article. Review.
-
Simulation-Based Methods for Model Building and Refinement in Cryoelectron Microscopy.J Chem Inf Model. 2020 May 26;60(5):2470-2483. doi: 10.1021/acs.jcim.0c00087. Epub 2020 Mar 31. J Chem Inf Model. 2020. PMID: 32202798 Free PMC article. Review.
-
Structural basis for ligand binding modes of CTP synthase.Proc Natl Acad Sci U S A. 2021 Jul 27;118(30):e2026621118. doi: 10.1073/pnas.2026621118. Proc Natl Acad Sci U S A. 2021. PMID: 34301892 Free PMC article.
-
Iterative Molecular Dynamics-Rosetta Membrane Protein Structure Refinement Guided by Cryo-EM Densities.J Chem Theory Comput. 2017 Oct 10;13(10):5131-5145. doi: 10.1021/acs.jctc.7b00464. Epub 2017 Sep 26. J Chem Theory Comput. 2017. PMID: 28949136 Free PMC article.
References
Publication types
MeSH terms
Substances
Grants and funding
LinkOut - more resources
Full Text Sources
Other Literature Sources