Impact of Information based Classification on Network Epidemics
- PMID: 27329348
- PMCID: PMC4916446
- DOI: 10.1038/srep28289
Impact of Information based Classification on Network Epidemics
Abstract
Formulating mathematical models for accurate approximation of malicious propagation in a network is a difficult process because of our inherent lack of understanding of several underlying physical processes that intrinsically characterize the broader picture. The aim of this paper is to understand the impact of available information in the control of malicious network epidemics. A 1-n-n-1 type differential epidemic model is proposed, where the differentiality allows a symptom based classification. This is the first such attempt to add such a classification into the existing epidemic framework. The model is incorporated into a five class system called the DifEpGoss architecture. Analysis reveals an epidemic threshold, based on which the long-term behavior of the system is analyzed. In this work three real network datasets with 22002, 22469 and 22607 undirected edges respectively, are used. The datasets show that classification based prevention given in the model can have a good role in containing network epidemics. Further simulation based experiments are used with a three category classification of attack and defense strengths, which allows us to consider 27 different possibilities. These experiments further corroborate the utility of the proposed model. The paper concludes with several interesting results.
Conflict of interest statement
The authors declare no competing financial interests.
Figures
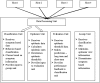
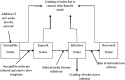
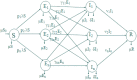
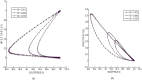
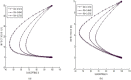
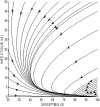
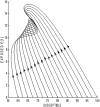
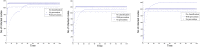
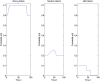
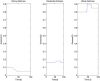
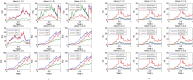
Similar articles
-
Modelling influenza A(H1N1) 2009 epidemics using a random network in a distributed computing environment.Acta Trop. 2015 Mar;143:29-35. doi: 10.1016/j.actatropica.2014.12.008. Epub 2015 Jan 3. Acta Trop. 2015. PMID: 25559047
-
Human mobility and time spent at destination: impact on spatial epidemic spreading.J Theor Biol. 2013 Dec 7;338:41-58. doi: 10.1016/j.jtbi.2013.08.032. Epub 2013 Sep 4. J Theor Biol. 2013. PMID: 24012488
-
A Network Epidemic Model with Preventive Rewiring: Comparative Analysis of the Initial Phase.Bull Math Biol. 2016 Dec;78(12):2427-2454. doi: 10.1007/s11538-016-0227-4. Epub 2016 Oct 31. Bull Math Biol. 2016. PMID: 27800576
-
Performance of a Computational Model of the Mammalian Olfactory System.In: Persaud KC, Marco S, Gutiérrez-Gálvez A, editors. Neuromorphic Olfaction. Boca Raton (FL): CRC Press/Taylor & Francis; 2013. Chapter 6. In: Persaud KC, Marco S, Gutiérrez-Gálvez A, editors. Neuromorphic Olfaction. Boca Raton (FL): CRC Press/Taylor & Francis; 2013. Chapter 6. PMID: 26042330 Free Books & Documents. Review.
-
Engineering Aspects of Olfaction.In: Persaud KC, Marco S, Gutiérrez-Gálvez A, editors. Neuromorphic Olfaction. Boca Raton (FL): CRC Press/Taylor & Francis; 2013. Chapter 1. In: Persaud KC, Marco S, Gutiérrez-Gálvez A, editors. Neuromorphic Olfaction. Boca Raton (FL): CRC Press/Taylor & Francis; 2013. Chapter 1. PMID: 26042329 Free Books & Documents. Review.
Cited by
-
Web malware spread modelling and optimal control strategies.Sci Rep. 2017 Feb 10;7:42308. doi: 10.1038/srep42308. Sci Rep. 2017. PMID: 28186203 Free PMC article.
-
Effective Methods of Restraining Diffusion in Terms of Epidemic Dynamics.Sci Rep. 2017 Jul 20;7(1):6013. doi: 10.1038/s41598-017-06171-6. Sci Rep. 2017. PMID: 28729607 Free PMC article.
References
-
- Chen Z., Gao L. & Kwait K. Modeling the spread of active worms, INFOCOM 2003, Twenty-Second Annual Joint Conference of the IEEE Computer and Communications Societies, California, USA: IEEE. (10.1109/INFCOM.2003.1209211) (2003, March 30-April 3). - DOI
-
- Zou C. C., Gong W. & Towsley D. Code Red Worm Propagation Modeling and Analysis. 9th ACM Conference on Computer and Communications Security, Washington DC, USA: ACM. (10.1145/586110.586130) (2002, November 18–22). - DOI
-
- Machie A., Roculan J., Russell R. & Velzen M. V. Incident Analysis Report on Nimda Worm Analysis. http://www.di.unisa.it/~ads/corso-security/www/CORSO-0102/NIMDA/link_loc.... (2001) (Date of access: 10th June, 2015).
-
- Wood P. et al.. Symantec Corporation Internet Security Threat Report 2011 Trends. http://www.symantec.com/content/en/us/enterprise/other_resources/b-istr_.... (2012) (Date of access: 10th June, 2015).
-
- Symantec Corporation Internet Security Threat Report 2012 Trends. http://www.symantec.com/content/en/us/enterprise/other_resources/b-istr_.... (2013) (Date of access: 10th June, 2015).
LinkOut - more resources
Full Text Sources
Other Literature Sources