Real-Time Predictions of Reservoir Size and Rebound Time during Antiretroviral Therapy Interruption Trials for HIV
- PMID: 27119536
- PMCID: PMC4847932
- DOI: 10.1371/journal.ppat.1005535
Real-Time Predictions of Reservoir Size and Rebound Time during Antiretroviral Therapy Interruption Trials for HIV
Erratum in
-
Correction: Real-Time Predictions of Reservoir Size and Rebound Time during Antiretroviral Therapy Interruption Trials for HIV.PLoS Pathog. 2016 Jul 13;12(7):e1005778. doi: 10.1371/journal.ppat.1005778. eCollection 2016 Jul. PLoS Pathog. 2016. PMID: 27409802 Free PMC article.
-
Correction: Real-Time Predictions of Reservoir Size and Rebound Time during Antiretroviral Therapy Interruption Trials for HIV.PLoS Pathog. 2017 Jul 6;13(7):e1006488. doi: 10.1371/journal.ppat.1006488. eCollection 2017 Jul. PLoS Pathog. 2017. PMID: 28683094 Free PMC article.
Abstract
Monitoring the efficacy of novel reservoir-reducing treatments for HIV is challenging. The limited ability to sample and quantify latent infection means that supervised antiretroviral therapy (ART) interruption studies are generally required. Here we introduce a set of mathematical and statistical modeling tools to aid in the design and interpretation of ART-interruption trials. We show how the likely size of the remaining reservoir can be updated in real-time as patients continue off treatment, by combining the output of laboratory assays with insights from models of reservoir dynamics and rebound. We design an optimal schedule for viral load sampling during interruption, whereby the frequency of follow-up can be decreased as patients continue off ART without rebound. While this scheme can minimize costs when the chance of rebound between visits is low, we find that the reservoir will be almost completely reseeded before rebound is detected unless sampling occurs at least every two weeks and the most sensitive viral load assays are used. We use simulated data to predict the clinical trial size needed to estimate treatment effects in the face of highly variable patient outcomes and imperfect reservoir assays. Our findings suggest that large numbers of patients-between 40 and 150-will be necessary to reliably estimate the reservoir-reducing potential of a new therapy and to compare this across interventions. As an example, we apply these methods to the two "Boston patients", recipients of allogeneic hematopoietic stem cell transplants who experienced large reductions in latent infection and underwent ART-interruption. We argue that the timing of viral rebound was not particularly surprising given the information available before treatment cessation. Additionally, we show how other clinical data can be used to estimate the relative contribution that remaining HIV+ cells in the recipient versus newly infected cells from the donor made to the residual reservoir that eventually caused rebound. Together, these tools will aid HIV researchers in the evaluating new potentially-curative strategies that target the latent reservoir.
Conflict of interest statement
The authors have declared that no competing interests exist.
Figures
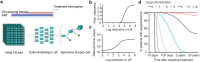
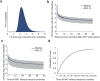
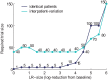
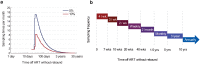
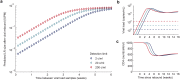
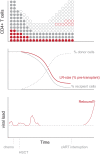
Similar articles
-
Predictions of time to HIV viral rebound following ART suspension that incorporate personal biomarkers.PLoS Comput Biol. 2019 Jul 24;15(7):e1007229. doi: 10.1371/journal.pcbi.1007229. eCollection 2019 Jul. PLoS Comput Biol. 2019. PMID: 31339888 Free PMC article.
-
Modeling of Antilatency Treatment in HIV: What Is the Optimal Duration of Antiretroviral Therapy-Free HIV Remission?J Virol. 2017 Nov 30;91(24):e01395-17. doi: 10.1128/JVI.01395-17. Print 2017 Dec 15. J Virol. 2017. PMID: 29021399 Free PMC article.
-
The size of the expressed HIV reservoir predicts timing of viral rebound after treatment interruption.AIDS. 2016 Jan 28;30(3):343-53. doi: 10.1097/QAD.0000000000000953. AIDS. 2016. PMID: 26588174 Free PMC article.
-
Lessons learned from HIV antiretroviral treatment interruption trials.Curr Opin HIV AIDS. 2018 Sep;13(5):416-421. doi: 10.1097/COH.0000000000000484. Curr Opin HIV AIDS. 2018. PMID: 29878912 Review.
-
Measuring the Size of the Latent Human Immunodeficiency Virus Reservoir: The Present and Future of Evaluating Eradication Strategies.J Infect Dis. 2017 Mar 15;215(suppl_3):S134-S141. doi: 10.1093/infdis/jiw648. J Infect Dis. 2017. PMID: 28520968 Free PMC article. Review.
Cited by
-
Oral cannabinoids in people living with HIV on effective antiretroviral therapy: CTN PT028-study protocol for a pilot randomised trial to assess safety, tolerability and effect on immune activation.BMJ Open. 2019 Jan 17;9(1):e024793. doi: 10.1136/bmjopen-2018-024793. BMJ Open. 2019. PMID: 30659041 Free PMC article.
-
Research priorities for an HIV cure: International AIDS Society Global Scientific Strategy 2021.Nat Med. 2021 Dec;27(12):2085-2098. doi: 10.1038/s41591-021-01590-5. Epub 2021 Dec 1. Nat Med. 2021. PMID: 34848888 Review.
-
Modeling HIV persistence and cure studies.Curr Opin HIV AIDS. 2018 Sep;13(5):428-434. doi: 10.1097/COH.0000000000000490. Curr Opin HIV AIDS. 2018. PMID: 29916846 Free PMC article. Review.
-
Understanding early HIV-1 rebound dynamics following antiretroviral therapy interruption: The importance of effector cell expansion.PLoS Pathog. 2024 Jul 29;20(7):e1012236. doi: 10.1371/journal.ppat.1012236. eCollection 2024 Jul. PLoS Pathog. 2024. PMID: 39074163 Free PMC article.
-
Measuring replication competent HIV-1: advances and challenges in defining the latent reservoir.Retrovirology. 2018 Feb 13;15(1):21. doi: 10.1186/s12977-018-0404-7. Retrovirology. 2018. PMID: 29433524 Free PMC article. Review.
References
-
- Durand CM, Blankson JN, Siliciano RF. Developing strategies for HIV-1 eradication. Trends in Immunology. 2012;33(11):554–562. Available from: http://www.sciencedirect.com/science/article/pii/S1471490612001123. 10.1016/j.it.2012.07.001 - DOI - PMC - PubMed
-
- Archin NM, Liberty AL, Kashuba AD, Choudhary SK, Kuruc JD, Crooks AM, et al. Administration of vorinostat disrupts HIV-1 latency in patients on antiretroviral therapy. Nature. 2012. July;487(7408):482–485. Available from: http://www.nature.com/nature/journal/v487/n7408/full/nature11286.html. 10.1038/nature11286 - DOI - PMC - PubMed
-
- Rasmussen TA, Tolstrup M, Brinkmann CR, Olesen R, Erikstrup C, Solomon A, et al. Panobinostat, a histone deacetylase inhibitor, for latent-virus reactivation in HIV-infected patients on suppressive antiretroviral therapy: a phase 1/2, single group, clinical trial. The Lancet HIV. 2014;1(1):e13–e21. Available from: http://www.sciencedirect.com/science/article/pii/S2352301814700141. 10.1016/S2352-3018(14)70014-1 - DOI - PubMed
-
- Spivak AM, Andrade A, Eisele E, Hoh R, Bacchetti P, Bumpus NN, et al. A Pilot Study Assessing the Safety and Latency-Reversing Activity of Disulfiram in HIV-1 Infected Adults on Antiretroviral Therapy. Clinical Infectious Diseases. 2014. March;58(6):883–890. Available from: http://cid.oxfordjournals.org/content/58/6/883. 10.1093/cid/cit813 - DOI - PMC - PubMed
Publication types
MeSH terms
Substances
Grants and funding
LinkOut - more resources
Full Text Sources
Other Literature Sources
Medical
Research Materials