CryptoSite: Expanding the Druggable Proteome by Characterization and Prediction of Cryptic Binding Sites
- PMID: 26854760
- PMCID: PMC4794384
- DOI: 10.1016/j.jmb.2016.01.029
CryptoSite: Expanding the Druggable Proteome by Characterization and Prediction of Cryptic Binding Sites
Abstract
Many proteins have small-molecule binding pockets that are not easily detectable in the ligand-free structures. These cryptic sites require a conformational change to become apparent; a cryptic site can therefore be defined as a site that forms a pocket in a holo structure, but not in the apo structure. Because many proteins appear to lack druggable pockets, understanding and accurately identifying cryptic sites could expand the set of drug targets. Previously, cryptic sites were identified experimentally by fragment-based ligand discovery and computationally by long molecular dynamics simulations and fragment docking. Here, we begin by constructing a set of structurally defined apo-holo pairs with cryptic sites. Next, we comprehensively characterize the cryptic sites in terms of their sequence, structure, and dynamics attributes. We find that cryptic sites tend to be as conserved in evolution as traditional binding pockets but are less hydrophobic and more flexible. Relying on this characterization, we use machine learning to predict cryptic sites with relatively high accuracy (for our benchmark, the true positive and false positive rates are 73% and 29%, respectively). We then predict cryptic sites in the entire structurally characterized human proteome (11,201 structures, covering 23% of all residues in the proteome). CryptoSite increases the size of the potentially "druggable" human proteome from ~40% to ~78% of disease-associated proteins. Finally, to demonstrate the utility of our approach in practice, we experimentally validate a cryptic site in protein tyrosine phosphatase 1B using a covalent ligand and NMR spectroscopy. The CryptoSite Web server is available at http://salilab.org/cryptosite.
Keywords: cryptic binding sites; machine learning; protein dynamics; undruggable proteins.
Published by Elsevier Ltd.
Figures
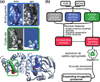
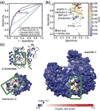
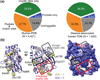
Similar articles
-
Predicting cryptic ligand binding sites based on normal modes guided conformational sampling.Proteins. 2021 Apr;89(4):416-426. doi: 10.1002/prot.26027. Epub 2020 Dec 5. Proteins. 2021. PMID: 33244830
-
Structure-Based Analysis of Cryptic-Site Opening.Structure. 2020 Feb 4;28(2):223-235.e2. doi: 10.1016/j.str.2019.11.007. Epub 2019 Dec 3. Structure. 2020. PMID: 31810712 Free PMC article.
-
Understanding Cryptic Pocket Formation in Protein Targets by Enhanced Sampling Simulations.J Am Chem Soc. 2016 Nov 2;138(43):14257-14263. doi: 10.1021/jacs.6b05425. Epub 2016 Oct 20. J Am Chem Soc. 2016. PMID: 27726386
-
Cryptic binding sites on proteins: definition, detection, and druggability.Curr Opin Chem Biol. 2018 Jun;44:1-8. doi: 10.1016/j.cbpa.2018.05.003. Epub 2018 May 23. Curr Opin Chem Biol. 2018. PMID: 29800865 Free PMC article. Review.
-
Investigating Cryptic Binding Sites by Molecular Dynamics Simulations.Acc Chem Res. 2020 Mar 17;53(3):654-661. doi: 10.1021/acs.accounts.9b00613. Epub 2020 Mar 5. Acc Chem Res. 2020. PMID: 32134250 Free PMC article. Review.
Cited by
-
Structure-based virtual screening of CYP1A1 inhibitors: towards rapid tier-one assessment of potential developmental toxicants.Arch Toxicol. 2021 Sep;95(9):3031-3048. doi: 10.1007/s00204-021-03111-2. Epub 2021 Jun 28. Arch Toxicol. 2021. PMID: 34181028 Free PMC article.
-
Which cryptic sites are feasible drug targets?Drug Discov Today. 2024 Nov;29(11):104197. doi: 10.1016/j.drudis.2024.104197. Epub 2024 Oct 4. Drug Discov Today. 2024. PMID: 39368697 Review.
-
Targeting the cryptic sites: NMR-based strategy to improve protein druggability by controlling the conformational equilibrium.Sci Adv. 2020 Sep 30;6(40):eabd0480. doi: 10.1126/sciadv.abd0480. Print 2020 Sep. Sci Adv. 2020. PMID: 32998885 Free PMC article.
-
The value of protein allostery in rational anticancer drug design: an update.Expert Opin Drug Discov. 2024 Sep;19(9):1071-1085. doi: 10.1080/17460441.2024.2384467. Epub 2024 Jul 28. Expert Opin Drug Discov. 2024. PMID: 39068599 Review.
-
Cryptic Sites in Tau Fibrils Explain the Preferential Binding of the AV-1451 PET Tracer toward Alzheimer's Tauopathy.ACS Chem Neurosci. 2021 Jul 7;12(13):2437-2447. doi: 10.1021/acschemneuro.0c00340. Epub 2021 Jun 21. ACS Chem Neurosci. 2021. PMID: 34152739 Free PMC article.
References
-
- Nisius B, Sha F, Gohlke H. Structure-based computational analysis of protein binding sites for function and druggability prediction. Journal of biotechnology. 2012;159(3):123–134. - PubMed
-
- Campbell SJ, Gold ND, Jackson RM, Westhead DR. Ligand binding: functional site location, similarity and docking. Current opinion in structural biology. 2003;13(3):389–395. - PubMed
-
- Laurie AT, Jackson RM. Q-SiteFinder: an energy-based method for the prediction of protein-ligand binding sites. Bioinformatics. 2005;21(9):1908–1916. - PubMed
Publication types
MeSH terms
Substances
Grants and funding
- R21 GM110580/GM/NIGMS NIH HHS/United States
- P30 DK063720/DK/NIDDK NIH HHS/United States
- R01 GM083960/GM/NIGMS NIH HHS/United States
- T32 GM008692/GM/NIGMS NIH HHS/United States
- U54 RR022220/RR/NCRR NIH HHS/United States
- Howard Hughes Medical Institute/United States
- DP5 OD009180/OD/NIH HHS/United States
- U54 GM094662/GM/NIGMS NIH HHS/United States
- T32 GM064337/GM/NIGMS NIH HHS/United States
- F31 CA180378/CA/NCI NIH HHS/United States
- P41 GM109824/GM/NIGMS NIH HHS/United States
- P01 AI091575/AI/NIAID NIH HHS/United States
- U01 GM098256/GM/NIGMS NIH HHS/United States
LinkOut - more resources
Full Text Sources
Other Literature Sources