DNA methylation outliers in normal breast tissue identify field defects that are enriched in cancer
- PMID: 26823093
- PMCID: PMC4740178
- DOI: 10.1038/ncomms10478
DNA methylation outliers in normal breast tissue identify field defects that are enriched in cancer
Abstract
Identifying molecular alterations in normal tissue adjacent to cancer is important for understanding cancer aetiology and designing preventive measures. Here we analyse the DNA methylome of 569 breast tissue samples, including 50 from cancer-free women and 84 from matched normal cancer pairs. We use statistical algorithms for dissecting intra- and inter-sample cellular heterogeneity and demonstrate that normal tissue adjacent to breast cancer is characterized by tens to thousands of epigenetic alterations. We show that their genomic distribution is non-random, being strongly enriched for binding sites of transcription factors specifying chromatin architecture. We validate the field defects in an independent cohort and demonstrate that over 30% of the alterations exhibit increased enrichment within matched cancer samples. Breast cancers highly enriched for epigenetic field defects, exhibit adverse clinical outcome. Our data support a model where clonal epigenetic reprogramming towards reduced differentiation in normal tissue is an important step in breast carcinogenesis.
Figures
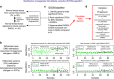
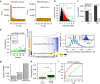
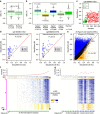
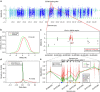
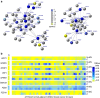
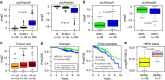
Similar articles
-
Stochastic epigenetic outliers can define field defects in cancer.BMC Bioinformatics. 2016 Apr 22;17:178. doi: 10.1186/s12859-016-1056-z. BMC Bioinformatics. 2016. PMID: 27103033 Free PMC article.
-
Detection of Epigenetic Field Defects Using a Weighted Epigenetic Distance-Based Method.Methods Mol Biol. 2020;2117:109-131. doi: 10.1007/978-1-0716-0301-7_6. Methods Mol Biol. 2020. PMID: 31960375
-
Methylome sequencing in triple-negative breast cancer reveals distinct methylation clusters with prognostic value.Nat Commun. 2015 Feb 2;6:5899. doi: 10.1038/ncomms6899. Nat Commun. 2015. PMID: 25641231
-
Epigenetic events associated with breast cancer and their prevention by dietary components targeting the epigenome.Chem Res Toxicol. 2012 Jan 13;25(1):61-73. doi: 10.1021/tx200378c. Epub 2011 Oct 28. Chem Res Toxicol. 2012. PMID: 21992498 Review.
-
Current and upcoming approaches to exploit the reversibility of epigenetic mutations in breast cancer.Breast Cancer Res. 2014 Jul 29;16(4):412. doi: 10.1186/s13058-014-0412-z. Breast Cancer Res. 2014. PMID: 25410383 Free PMC article. Review.
Cited by
-
Signaling Pathway Alterations Driven by BRCA1 and BRCA2 Germline Mutations are Sufficient to Initiate Breast Tumorigenesis by the PIK3CAH1047R Oncogene.Cancer Res Commun. 2024 Jan 5;4(1):38-54. doi: 10.1158/2767-9764.CRC-23-0330. Cancer Res Commun. 2024. PMID: 38059556 Free PMC article.
-
A novel epigenetic signature for overall survival prediction in patients with breast cancer.J Transl Med. 2019 Nov 20;17(1):380. doi: 10.1186/s12967-019-2126-6. J Transl Med. 2019. PMID: 31747912 Free PMC article.
-
DNA methylation at quantitative trait loci (mQTLs) varies with cell type and nonheritable factors and may improve breast cancer risk assessment.NPJ Precis Oncol. 2023 Sep 27;7(1):99. doi: 10.1038/s41698-023-00452-2. NPJ Precis Oncol. 2023. PMID: 37758816 Free PMC article.
-
DNA methylation signatures of monozygotic twins clinically discordant for multiple sclerosis.Nat Commun. 2019 May 7;10(1):2094. doi: 10.1038/s41467-019-09984-3. Nat Commun. 2019. PMID: 31064978 Free PMC article.
-
Notch, SUMOylation, and ESR-Mediated Signalling Are the Main Molecular Pathways Showing Significantly Different Epimutation Scores between Expressing or Not Oestrogen Receptor Breast Cancer in Three Public EWAS Datasets.Cancers (Basel). 2023 Aug 15;15(16):4109. doi: 10.3390/cancers15164109. Cancers (Basel). 2023. PMID: 37627137 Free PMC article.
References
-
- Maley C. C. et al.. Genetic clonal diversity predicts progression to esophageal adenocarcinoma. Nature Genet. 38, 468–473 (2006). - PubMed
-
- Shah S. P. et al.. Mutational evolution in a lobular breast tumour profiled at single nucleotide resolution. Nature 461, 809–813 (2009). - PubMed
-
- Merlo L. M., Pepper J. W., Reid B. J. & Maley C. C. Cancer as an evolutionary and ecological process. Nat. Rev. Cancer 6, 924–935 (2006). - PubMed
Publication types
MeSH terms
Grants and funding
LinkOut - more resources
Full Text Sources
Other Literature Sources
Medical
Molecular Biology Databases