Confounding Factors in the Transcriptome Analysis of an In-Vivo Exposure Experiment
- PMID: 26789003
- PMCID: PMC4720430
- DOI: 10.1371/journal.pone.0145252
Confounding Factors in the Transcriptome Analysis of an In-Vivo Exposure Experiment
Abstract
Confounding factors: In transcriptomics experimentation, confounding factors frequently exist alongside the intended experimental factors and can severely influence the outcome of a transcriptome analysis. Confounding factors are regularly discussed in methodological literature, but their actual, practical impact on the outcome and interpretation of transcriptomics experiments is, to our knowledge, not documented. For instance, in-vivo experimental factors; like Individual, Sample-Composition and Time-of-Day are potentially formidable confounding factors. To study these confounding factors, we designed an extensive in-vivo transcriptome experiment (n = 264) with UVR exposure of murine skin containing six consecutive samples from each individual mouse (n = 64).
Analysis approach: Evaluation of the confounding factors: Sample-Composition, Time-of-Day, Handling-Stress, and Individual-Mouse resulted in the identification of many genes that were affected by them. These genes sometimes showed over 30-fold expression differences. The most prominent confounding factor was Sample-Composition caused by mouse-dependent skin composition differences, sampling variation and/or influx/efflux of mobile cells. Although we can only evaluate these effects for known cell type specifically expressed genes in our complex heterogeneous samples, it is clear that the observed variations also affect the cumulative expression levels of many other non-cell-type-specific genes.
Anova: ANOVA analysis can only attempt to neutralize the effects of the well-defined confounding factors, such as Individual-Mouse, on the experimental factors UV-Dose and Recovery-Time. Also, by definition, ANOVA only yields reproducible gene-expression differences, but we found that these differences were very small compared to the fold changes induced by the confounding factors, questioning the biological relevance of these ANOVA-detected differences. Furthermore, it turned out that many of the differentially expressed genes found by ANOVA were also present in the gene clusters associated with the confounding factors.
Conclusion: Hence our overall conclusion is that confounding factors have a major impact on the outcome of in-vivo transcriptomics experiments. Thus the set-up, analysis, and interpretation of such experiments should be approached with the utmost prudence.
Conflict of interest statement
Figures
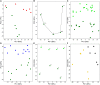
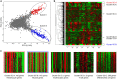
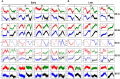
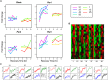
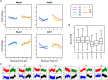
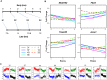
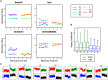
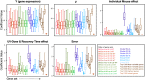
Similar articles
-
A range finding protocol to support design for transcriptomics experimentation: examples of in-vitro and in-vivo murine UV exposure.PLoS One. 2014 May 13;9(5):e97089. doi: 10.1371/journal.pone.0097089. eCollection 2014. PLoS One. 2014. PMID: 24823911 Free PMC article.
-
Identification of distinct and common gene expression changes after oxidative stress and gamma and ultraviolet radiation.Mol Carcinog. 2003 Jun;37(2):65-82. doi: 10.1002/mc.10122. Mol Carcinog. 2003. PMID: 12766906
-
Identification of Skin injury-related genes induced by ionizing radiation in human keratinocytes using cDNA microarray.J Radiat Res. 2005 Jun;46(2):173-84. doi: 10.1269/jrr.46.173. J Radiat Res. 2005. PMID: 15988135
-
Identification of differentially expressed genes in mouse kidney after irradiation using microarray analysis.Radiat Res. 2004 Jan;161(1):28-38. doi: 10.1667/rr3097. Radiat Res. 2004. PMID: 14680399
-
Differential expression of oxidative stress and extracellular matrix remodeling genes in low- or high-dose-rate photon-irradiated skin.Radiat Res. 2011 Aug;176(2):187-97. doi: 10.1667/rr2493.1. Epub 2011 May 16. Radiat Res. 2011. PMID: 21574862
Cited by
-
Comparative Genomics and Evolution of Avian Specialized Traits.Curr Genomics. 2021 Dec 31;22(7):496-511. doi: 10.2174/1389202923666211227143952. Curr Genomics. 2021. PMID: 35386431 Free PMC article. Review.
-
Genome-wide identification of directed gene networks using large-scale population genomics data.Nat Commun. 2018 Aug 6;9(1):3097. doi: 10.1038/s41467-018-05452-6. Nat Commun. 2018. PMID: 30082726 Free PMC article.
-
Human pancreatic islet microRNAs implicated in diabetes and related traits by large-scale genetic analysis.Proc Natl Acad Sci U S A. 2023 Feb 14;120(7):e2206797120. doi: 10.1073/pnas.2206797120. Epub 2023 Feb 9. Proc Natl Acad Sci U S A. 2023. PMID: 36757889 Free PMC article.
-
Accurate estimation of cell composition in bulk expression through robust integration of single-cell information.Nat Commun. 2020 Apr 24;11(1):1971. doi: 10.1038/s41467-020-15816-6. Nat Commun. 2020. PMID: 32332754 Free PMC article.
-
The Role of Systems Biology in Deciphering Asthma Heterogeneity.Life (Basel). 2022 Oct 8;12(10):1562. doi: 10.3390/life12101562. Life (Basel). 2022. PMID: 36294997 Free PMC article. Review.
References
-
- Flaherty P, Arkin A, Jordan M. Robust design of biological experiments. Adv Neural Inf Process Syst 18. 2005; Available: http://machinelearning.wustl.edu/mlpapers/paper_files/NIPS2005_647.pdf
Publication types
MeSH terms
Grants and funding
LinkOut - more resources
Full Text Sources
Other Literature Sources