Mapping residual transmission for malaria elimination
- PMID: 26714110
- PMCID: PMC4744184
- DOI: 10.7554/eLife.09520
Mapping residual transmission for malaria elimination
Abstract
Eliminating malaria from a defined region involves draining the endemic parasite reservoir and minimizing local malaria transmission around imported malaria infections . In the last phases of malaria elimination, as universal interventions reap diminishing marginal returns, national resources must become increasingly devoted to identifying where residual transmission is occurring. The needs for accurate measures of progress and practical advice about how to allocate scarce resources require new analytical methods to quantify fine-grained heterogeneity in malaria risk. Using routine national surveillance data from Swaziland (a sub-Saharan country on the verge of elimination), we estimated individual reproductive numbers. Fine-grained maps of reproductive numbers and local malaria importation rates were combined to show 'malariogenic potential', a first for malaria elimination. As countries approach elimination, these individual-based measures of transmission risk provide meaningful metrics for planning programmatic responses and prioritizing areas where interventions will contribute most to malaria elimination.
Keywords: ecology; epidemiology; global health; human; malaria elimination; plasmodium falciparum; spatio-temporal transmission dynamics.
Conflict of interest statement
The authors declare that no competing interests exist.
Figures
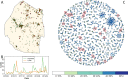
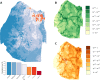
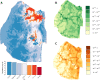
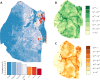
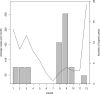
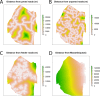
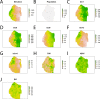
Similar articles
-
Changing epidemiology and challenges of malaria in China towards elimination.Malar J. 2019 Mar 29;18(1):107. doi: 10.1186/s12936-019-2736-8. Malar J. 2019. PMID: 30922301 Free PMC article.
-
Towards malaria elimination in the MOSASWA (Mozambique, South Africa and Swaziland) region.Malar J. 2016 Aug 18;15(1):419. doi: 10.1186/s12936-016-1470-8. Malar J. 2016. PMID: 27538990 Free PMC article.
-
Sustaining control: lessons from the Lubombo spatial development initiative in southern Africa.Malar J. 2016 Aug 12;15(1):409. doi: 10.1186/s12936-016-1453-9. Malar J. 2016. PMID: 27520364 Free PMC article.
-
malERA: An updated research agenda for characterising the reservoir and measuring transmission in malaria elimination and eradication.PLoS Med. 2017 Nov 30;14(11):e1002452. doi: 10.1371/journal.pmed.1002452. eCollection 2017 Nov. PLoS Med. 2017. PMID: 29190279 Free PMC article. Review.
-
Use of epidemiological and entomological tools in the control and elimination of malaria in Ethiopia.Malar J. 2018 Jan 12;17(1):26. doi: 10.1186/s12936-018-2172-1. Malar J. 2018. PMID: 29329545 Free PMC article. Review.
Cited by
-
Assessing receptivity to malaria using case surveillance and forest data in a near-elimination setting in northeast Thailand.Malar J. 2024 Jul 30;23(1):224. doi: 10.1186/s12936-024-05044-4. Malar J. 2024. PMID: 39080748 Free PMC article.
-
Factors related to human-vector contact that modify the likelihood of malaria transmission during a contained Plasmodium falciparum outbreak in Praia, Cabo Verde.Front Epidemiol. 2022 Nov 25;2:1031230. doi: 10.3389/fepid.2022.1031230. eCollection 2022. Front Epidemiol. 2022. PMID: 38455281 Free PMC article.
-
Characterizing the spatial distribution of multiple malaria diagnostic endpoints in a low-transmission setting in Lao PDR.Front Med (Lausanne). 2022 Aug 18;9:929366. doi: 10.3389/fmed.2022.929366. eCollection 2022. Front Med (Lausanne). 2022. PMID: 36059850 Free PMC article.
-
Spatio-temporal monitoring of health facility-level malaria trends in Zambia and adaptive scaling for operational intervention.Commun Med (Lond). 2022 Jul 1;2:79. doi: 10.1038/s43856-022-00144-1. eCollection 2022. Commun Med (Lond). 2022. PMID: 35789566 Free PMC article.
-
Receptivity to malaria: meaning and measurement.Malar J. 2022 May 8;21(1):145. doi: 10.1186/s12936-022-04155-0. Malar J. 2022. PMID: 35527264 Free PMC article. Review.
References
-
- Anderson RM, May RM. Infectious Diseases of Humans. Oxford: Oxford University Press; 1991.
-
- Anderson R P. Novel methods improve prediction of species’ distributions from occurrence data. Ecography. 2006;29:129–151.
-
- Bejon P, Williams TN, Nyundo C, Hay SI, Benz D, Gething PW, Otiende M, Peshu J, Bashraheil M, Greenhouse B, Bousema T, Bauni E, Marsh K, Smith DL, Borrmann S. A micro-epidemiological analysis of febrile malaria in coastal kenya showing hotspots within hotspots. eLife. 2014;3:e02130. doi: 10.7554/eLife.02130. - DOI - PMC - PubMed
-
- Bousema T, Drakeley C, Gesase S, Hashim R, Magesa S, Mosha F, Otieno S, Carneiro I, Cox J, Msuya E, Kleinschmidt I, Maxwell C, Greenwood B, Riley E, Sauerwein R, Chandramohan D, Gosling R. Identification of hot spots of malaria transmission for targeted malaria control. The Journal of Infectious Diseases. 2010;201:1764–1774. doi: 10.1086/652456. - DOI - PubMed
MeSH terms
Grants and funding
LinkOut - more resources
Full Text Sources
Medical
Research Materials