Interactive Big Data Resource to Elucidate Human Immune Pathways and Diseases
- PMID: 26362267
- PMCID: PMC4753773
- DOI: 10.1016/j.immuni.2015.08.014
Interactive Big Data Resource to Elucidate Human Immune Pathways and Diseases
Abstract
Many functionally important interactions between genes and proteins involved in immunological diseases and processes are unknown. The exponential growth in public high-throughput data offers an opportunity to expand this knowledge. To unlock human-immunology-relevant insight contained in the global biomedical research effort, including all public high-throughput datasets, we performed immunological-pathway-focused Bayesian integration of a comprehensive, heterogeneous compendium comprising 38,088 genome-scale experiments. The distillation of this knowledge into immunological networks of functional relationships between molecular entities (ImmuNet), and tools to mine this resource, are accessible to the public at http://immunet.princeton.edu. The predictive capacity of ImmuNet, established by rigorous statistical validation, is easily accessed by experimentalists to generate data-driven hypotheses. We demonstrate the power of this approach through the identification of unique host-virus interaction responses, and we show how ImmuNet complements genetic studies by predicting disease-associated genes. ImmuNet should be widely beneficial for investigating the mechanisms of the human immune system and immunological diseases.
Copyright © 2015 Elsevier Inc. All rights reserved.
Figures
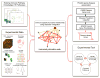
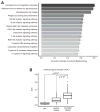
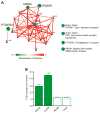
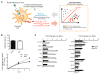
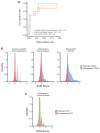
Similar articles
-
Immunological Genome Project and systems immunology.Trends Immunol. 2013 Dec;34(12):602-9. doi: 10.1016/j.it.2013.03.004. Epub 2013 Apr 27. Trends Immunol. 2013. PMID: 23631936 Free PMC article. Review.
-
Systems Biology Approaches to Understanding the Human Immune System.Front Immunol. 2020 Jul 30;11:1683. doi: 10.3389/fimmu.2020.01683. eCollection 2020. Front Immunol. 2020. PMID: 32849587 Free PMC article.
-
Computational prediction of virus-human protein-protein interactions using embedding kernelized heterogeneous data.Mol Biosyst. 2016 May 24;12(6):1976-86. doi: 10.1039/c6mb00065g. Mol Biosyst. 2016. PMID: 27072625
-
Integrated functional networks of process, tissue, and developmental stage specific interactions in Arabidopsis thaliana.BMC Syst Biol. 2010 Dec 31;4:180. doi: 10.1186/1752-0509-4-180. BMC Syst Biol. 2010. PMID: 21194434 Free PMC article.
-
Unlocking immune-mediated disease mechanisms with transcriptomics.Biochem Soc Trans. 2021 Apr 30;49(2):705-714. doi: 10.1042/BST20200652. Biochem Soc Trans. 2021. PMID: 33843974 Free PMC article. Review.
Cited by
-
A platinum@polymer-catechol nanobraker enables radio-immunotherapy for crippling melanoma tumorigenesis, angiogenesis, and radioresistance.Bioact Mater. 2022 Sep 24;22:34-46. doi: 10.1016/j.bioactmat.2022.09.006. eCollection 2023 Apr. Bioact Mater. 2022. PMID: 36203954 Free PMC article.
-
Proteomic Applications in Antimicrobial Resistance and Clinical Microbiology Studies.Infect Drug Resist. 2020 Jun 16;13:1785-1806. doi: 10.2147/IDR.S238446. eCollection 2020. Infect Drug Resist. 2020. PMID: 32606829 Free PMC article. Review.
-
Interferon-γ Represses M2 Gene Expression in Human Macrophages by Disassembling Enhancers Bound by the Transcription Factor MAF.Immunity. 2017 Aug 15;47(2):235-250.e4. doi: 10.1016/j.immuni.2017.07.017. Immunity. 2017. PMID: 28813657 Free PMC article.
-
Proteomics and integrative omic approaches for understanding host-pathogen interactions and infectious diseases.Mol Syst Biol. 2017 Mar 27;13(3):922. doi: 10.15252/msb.20167062. Mol Syst Biol. 2017. PMID: 28348067 Free PMC article. Review.
-
Solving Immunology?Trends Immunol. 2017 Feb;38(2):116-127. doi: 10.1016/j.it.2016.11.006. Epub 2016 Dec 13. Trends Immunol. 2017. PMID: 27986392 Free PMC article. Review.
References
-
- Albert ML, Sauter B, Bhardwaj N. Dendritic cells acquire antigen from apoptotic cells and induce class I-restricted CTLs. Nature. 1998;392:86–89. - PubMed
-
- Bhattacharya S, Andorf S, Gomes L, Dunn P, Schaefer H, Pontius J, Berger P, Desborough V, Smith T, Campbell J, et al. ImmPort: disseminating data to the public for the future of immunology. Immunol Res. 2014;58:234–239. - PubMed
-
- Briggs RC, Shults KE, Flye LA, McClintock-Treep SA, Jagasia MH, Goodman SA, Boulos FI, Jacobberger JW, Stelzer GT, Head DR. Dysregulated human myeloid nuclear differentiation antigen expression in myelodysplastic syndromes: evidence for a role in apoptosis. Cancer Res. 2006;66:4645–4651. - PubMed
Publication types
MeSH terms
Grants and funding
LinkOut - more resources
Full Text Sources
Other Literature Sources
Medical