Gene set analysis approaches for RNA-seq data: performance evaluation and application guideline
- PMID: 26342128
- PMCID: PMC4870397
- DOI: 10.1093/bib/bbv069
Gene set analysis approaches for RNA-seq data: performance evaluation and application guideline
Abstract
Transcriptome sequencing (RNA-seq) is gradually replacing microarrays for high-throughput studies of gene expression. The main challenge of analyzing microarray data is not in finding differentially expressed genes, but in gaining insights into the biological processes underlying phenotypic differences. To interpret experimental results from microarrays, gene set analysis (GSA) has become the method of choice, in particular because it incorporates pre-existing biological knowledge (in a form of functionally related gene sets) into the analysis. Here we provide a brief review of several statistically different GSA approaches (competitive and self-contained) that can be adapted from microarrays practice as well as those specifically designed for RNA-seq. We evaluate their performance (in terms of Type I error rate, power, robustness to the sample size and heterogeneity, as well as the sensitivity to different types of selection biases) on simulated and real RNA-seq data. Not surprisingly, the performance of various GSA approaches depends only on the statistical hypothesis they test and does not depend on whether the test was developed for microarrays or RNA-seq data. Interestingly, we found that competitive methods have lower power as well as robustness to the samples heterogeneity than self-contained methods, leading to poor results reproducibility. We also found that the power of unsupervised competitive methods depends on the balance between up- and down-regulated genes in tested gene sets. These properties of competitive methods have been overlooked before. Our evaluation provides a concise guideline for selecting GSA approaches, best performing under particular experimental settings in the context of RNA-seq.
Keywords: RNA-seq; competitive; gene set analysis; robustness; self-contained.
© The Author 2015. Published by Oxford University Press.
Figures
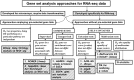
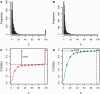
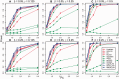
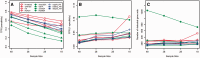
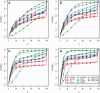
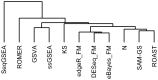
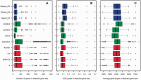
Similar articles
-
Comparative evaluation of gene set analysis approaches for RNA-Seq data.BMC Bioinformatics. 2014 Dec 5;15(1):397. doi: 10.1186/s12859-014-0397-8. BMC Bioinformatics. 2014. PMID: 25475910 Free PMC article.
-
Extracting the Strongest Signals from Omics Data: Differentially Expressed Pathways and Beyond.Methods Mol Biol. 2017;1613:125-159. doi: 10.1007/978-1-4939-7027-8_7. Methods Mol Biol. 2017. PMID: 28849561 Free PMC article.
-
Robust identification of differentially expressed genes from RNA-seq data.Genomics. 2020 Mar;112(2):2000-2010. doi: 10.1016/j.ygeno.2019.11.012. Epub 2019 Nov 20. Genomics. 2020. PMID: 31756426
-
Statistical detection of differentially expressed genes based on RNA-seq: from biological to phylogenetic replicates.Brief Bioinform. 2016 Mar;17(2):243-8. doi: 10.1093/bib/bbv035. Epub 2015 Jun 24. Brief Bioinform. 2016. PMID: 26108230 Review.
-
RNA-Seq methods for transcriptome analysis.Wiley Interdiscip Rev RNA. 2017 Jan;8(1):10.1002/wrna.1364. doi: 10.1002/wrna.1364. Epub 2016 May 19. Wiley Interdiscip Rev RNA. 2017. PMID: 27198714 Free PMC article. Review.
Cited by
-
Discovery of Selenocysteine as a Potential Nanomedicine Promotes Cartilage Regeneration With Enhanced Immune Response by Text Mining and Biomedical Databases.Front Pharmacol. 2020 Jul 24;11:1138. doi: 10.3389/fphar.2020.01138. eCollection 2020. Front Pharmacol. 2020. PMID: 32792959 Free PMC article.
-
Longitudinal linear combination test for gene set analysis.BMC Bioinformatics. 2019 Dec 10;20(1):650. doi: 10.1186/s12859-019-3221-7. BMC Bioinformatics. 2019. PMID: 31822265 Free PMC article.
-
GSAR: Bioconductor package for Gene Set analysis in R.BMC Bioinformatics. 2017 Jan 24;18(1):61. doi: 10.1186/s12859-017-1482-6. BMC Bioinformatics. 2017. PMID: 28118818 Free PMC article.
-
Roastgsa: a comparison of rotation-based scores for gene set enrichment analysis.BMC Bioinformatics. 2023 Oct 30;24(1):408. doi: 10.1186/s12859-023-05510-x. BMC Bioinformatics. 2023. PMID: 37904108 Free PMC article.
-
Resolving host-pathogen interactions by dual RNA-seq.PLoS Pathog. 2017 Feb 16;13(2):e1006033. doi: 10.1371/journal.ppat.1006033. eCollection 2017 Feb. PLoS Pathog. 2017. PMID: 28207848 Free PMC article. Review.
References
MeSH terms
Substances
Grants and funding
LinkOut - more resources
Full Text Sources
Other Literature Sources