Drugs that reverse disease transcriptomic signatures are more effective in a mouse model of dyslipidemia
- PMID: 26148350
- PMCID: PMC4380926
- DOI: 10.15252/msb.20145486
Drugs that reverse disease transcriptomic signatures are more effective in a mouse model of dyslipidemia
Abstract
High-throughput omics have proven invaluable in studying human disease, and yet day-to-day clinical practice still relies on physiological, non-omic markers. The metabolic syndrome, for example, is diagnosed and monitored by blood and urine indices such as blood cholesterol levels. Nevertheless, the association between the molecular and the physiological manifestations of the disease, especially in response to treatment, has not been investigated in a systematic manner. To this end, we studied a mouse model of diet-induced dyslipidemia and atherosclerosis that was subject to various drug treatments relevant to the disease in question. Both physiological data and gene expression data (from the liver and white adipose) were analyzed and compared. We find that treatments that restore gene expression patterns to their norm are associated with the successful restoration of physiological markers to their baselines. This holds in a tissue-specific manner—treatments that reverse the transcriptomic signatures of the disease in a particular tissue are associated with positive physiological effects in that tissue. Further, treatments that introduce large non-restorative gene expression alterations are associated with unfavorable physiological outcomes. These results provide a sound basis to in silico methods that rely on omic metrics for drug repurposing and drug discovery by searching for compounds that reverse a disease’s omic signatures. Moreover, they highlight the need to develop drugs that restore the global cellular state to its healthy norm rather than rectify particular disease phenotypes.
Figures
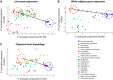
A-C The figure shows the first two principal components of (A) the gene expression space of the liver, (B) the gene expression space of white adipose tissue, and (C) the physiological space. In all panels, each dot represents one animal; color codes denote the different experimental groups. Red squares represent the HFD animals, and blue triangles represent the LFD animals. The dashed arrow connects the HFD centroid (yellow square, circled in red) to the LFD centroid (yellow triangle, circled in blue) and denotes the direction of a reversal of the gene expression or physiological state back to the norm.
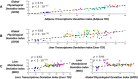
A, B The correlation between the global physiological deviation index (GPDI) and the transcriptomic deviation index (TDI) in (A) white adipose tissue and (B) liver tissue.
C Left panel: the correlation between the liver metabolome deviation index (MDI) and the liver TDI. Right panel: the correlation between the liver MDI and the GPDI.
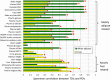
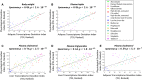
A-D White adipose (A, B) and liver (C, D) TDIs (deviations from the baseline gene expression) are correlated with PDIs (deviations from the baseline physiology) across different experimental groups and treatment regimens. PDIs of four physiological markers are shown, two that are highly correlated with adipose transcriptome (A, B) and two that are highly correlated with hepatic transcriptome (C, D).
E Adipose TDIs and plasma cholesterol PDIs are generally correlated, but this does not hold for animals treated with T090137 (purple dots at the top left corner of the figure), probably due to this drug's particular mechanism of action (see main text). The correlation displayed in (E) increases from 0.59 to 0.8 (P < 1.3e-13) when excluding the T090137 animals.
Similar articles
-
An experimental study on amelioration of dyslipidemia-induced atherosclesis by Clematichinenoside through regulating Peroxisome proliferator-activated receptor-α mediated apolipoprotein A-I, A-II and C-III.Eur J Pharmacol. 2015 Aug 15;761:362-74. doi: 10.1016/j.ejphar.2015.04.015. Epub 2015 May 12. Eur J Pharmacol. 2015. PMID: 25979856
-
Dioscoreophyllum cumminsii (Stapf) Diels leaves halt high-fructose induced metabolic syndrome: Hyperglycemia, insulin resistance, inflammation and oxidative stress.J Ethnopharmacol. 2016 Nov 4;192:471-479. doi: 10.1016/j.jep.2016.08.024. Epub 2016 Aug 26. J Ethnopharmacol. 2016. PMID: 27568876
-
I4, a synthetic anti-diabetes agent, attenuates atherosclerosis through its lipid-lowering, anti-inflammatory and anti-apoptosis properties.Mol Cell Endocrinol. 2017 Jan 15;440:80-92. doi: 10.1016/j.mce.2016.10.007. Epub 2016 Oct 8. Mol Cell Endocrinol. 2017. PMID: 27725191
-
Lipid pharmacotherapy for treatment of atherosclerosis.Expert Opin Pharmacother. 2014 Jun;15(8):1119-25. doi: 10.1517/14656566.2014.904287. Epub 2014 Apr 5. Expert Opin Pharmacother. 2014. PMID: 24702590 Review.
-
Optimal management of combined dyslipidemia: what have we behind statins monotherapy?Adv Cardiol. 2008;45:127-153. doi: 10.1159/000115192. Adv Cardiol. 2008. PMID: 18230960 Review.
Cited by
-
A survey of optimal strategy for signature-based drug repositioning and an application to liver cancer.Elife. 2022 Feb 22;11:e71880. doi: 10.7554/eLife.71880. Elife. 2022. PMID: 35191375 Free PMC article.
-
Prediction and identification of synergistic compound combinations against pancreatic cancer cells.iScience. 2021 Sep 3;24(9):103080. doi: 10.1016/j.isci.2021.103080. eCollection 2021 Sep 24. iScience. 2021. PMID: 34585118 Free PMC article.
-
On the correspondence between the transcriptomic response of a compound and its effects on its targets.BMC Bioinformatics. 2023 May 19;24(1):207. doi: 10.1186/s12859-023-05337-6. BMC Bioinformatics. 2023. PMID: 37208587 Free PMC article.
-
Transcription Factor Activation Profiles (TFAP) identify compounds promoting differentiation of Acute Myeloid Leukemia cell lines.Cell Death Discov. 2022 Jan 10;8(1):16. doi: 10.1038/s41420-021-00811-7. Cell Death Discov. 2022. PMID: 35013135 Free PMC article.
-
Use of big data in drug development for precision medicine: an update.Expert Rev Precis Med Drug Dev. 2019;4(3):189-200. doi: 10.1080/23808993.2019.1617632. Epub 2019 May 20. Expert Rev Precis Med Drug Dev. 2019. PMID: 31286058 Free PMC article.
References
-
- Aggarwal CC, Hinneburg A, Keim DA. Proceedings of the 8th. London, UK: Springer-Verlag; 2001. On the Surprising Behavior of Distance Metrics in High Dimensional Spaces; pp. 420–434. International Conference on Database Theory.
-
- Awtry EH, Loscalzo J. Aspirin. Circulation. 2000;101:1206–1218. - PubMed
-
- Backes JM, Gibson CA, Ruisinger JF, Moriarty PM. Fibrates: what have we learned in the past 40 years? Pharmacother J Hum Pharmacol Drug Ther. 2007;27:412–424. - PubMed
-
- Bahrami H, Bluemke DA, Kronmal R, Bertoni AG, Lloyd-Jones DM, Shahar E, Szklo M, Lima JAC. Novel metabolic risk factors for incident heart failure and their relationship with obesity: the MESA (Multi-Ethnic Study of Atherosclerosis) study. J Am Coll Cardiol. 2008;51:1775–1783. - PubMed
Publication types
MeSH terms
Substances
LinkOut - more resources
Full Text Sources
Other Literature Sources
Medical