The Discovery of Novel Biomarkers Improves Breast Cancer Intrinsic Subtype Prediction and Reconciles the Labels in the METABRIC Data Set
- PMID: 26132585
- PMCID: PMC4488510
- DOI: 10.1371/journal.pone.0129711
The Discovery of Novel Biomarkers Improves Breast Cancer Intrinsic Subtype Prediction and Reconciles the Labels in the METABRIC Data Set
Abstract
Background: The prediction of breast cancer intrinsic subtypes has been introduced as a valuable strategy to determine patient diagnosis and prognosis, and therapy response. The PAM50 method, based on the expression levels of 50 genes, uses a single sample predictor model to assign subtype labels to samples. Intrinsic errors reported within this assay demonstrate the challenge of identifying and understanding the breast cancer groups. In this study, we aim to: a) identify novel biomarkers for subtype individuation by exploring the competence of a newly proposed method named CM1 score, and b) apply an ensemble learning, as opposed to the use of a single classifier, for sample subtype assignment. The overarching objective is to improve class prediction.
Methods and findings: The microarray transcriptome data sets used in this study are: the METABRIC breast cancer data recorded for over 2000 patients, and the public integrated source from ROCK database with 1570 samples. We first computed the CM1 score to identify the probes with highly discriminative patterns of expression across samples of each intrinsic subtype. We further assessed the ability of 42 selected probes on assigning correct subtype labels using 24 different classifiers from the Weka software suite. For comparison, the same method was applied on the list of 50 genes from the PAM50 method.
Conclusions: The CM1 score portrayed 30 novel biomarkers for predicting breast cancer subtypes, with the confirmation of the role of 12 well-established genes. Intrinsic subtypes assigned using the CM1 list and the ensemble of classifiers are more consistent and homogeneous than the original PAM50 labels. The new subtypes show accurate distributions of current clinical markers ER, PR and HER2, and survival curves in the METABRIC and ROCK data sets. Remarkably, the paradoxical attribution of the original labels reinforces the limitations of employing a single sample classifiers to predict breast cancer intrinsic subtypes.
Conflict of interest statement
Figures
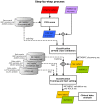
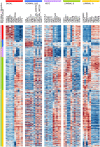
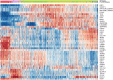
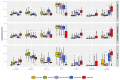
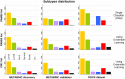
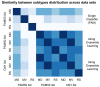
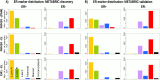
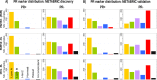
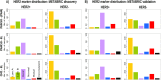
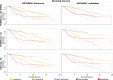
Similar articles
-
Iteratively refining breast cancer intrinsic subtypes in the METABRIC dataset.BioData Min. 2016 Jan 13;9:2. doi: 10.1186/s13040-015-0078-9. eCollection 2016. BioData Min. 2016. PMID: 26770261 Free PMC article.
-
Quantification of intrinsic subtype ambiguity in Luminal A breast cancer and its relationship to clinical outcomes.BMC Cancer. 2019 Mar 8;19(1):215. doi: 10.1186/s12885-019-5392-z. BMC Cancer. 2019. PMID: 30849944 Free PMC article.
-
PAM50 breast cancer subtyping by RT-qPCR and concordance with standard clinical molecular markers.BMC Med Genomics. 2012 Oct 4;5:44. doi: 10.1186/1755-8794-5-44. BMC Med Genomics. 2012. PMID: 23035882 Free PMC article.
-
Predictive and prognostic biomarkers in breast tumours.Pathology. 2024 Mar;56(2):186-191. doi: 10.1016/j.pathol.2023.10.014. Epub 2023 Dec 12. Pathology. 2024. PMID: 38212230 Review.
-
Gene Expression Profiles in Cancers and Their Therapeutic Implications.Cancer J. 2023 Jan-Feb 01;29(1):9-14. doi: 10.1097/PPO.0000000000000638. Cancer J. 2023. PMID: 36693152 Free PMC article. Review.
Cited by
-
CLCA2 is a positive regulator of store-operated calcium entry and TMEM16A.PLoS One. 2018 May 14;13(5):e0196512. doi: 10.1371/journal.pone.0196512. eCollection 2018. PLoS One. 2018. PMID: 29758025 Free PMC article.
-
Iteratively refining breast cancer intrinsic subtypes in the METABRIC dataset.BioData Min. 2016 Jan 13;9:2. doi: 10.1186/s13040-015-0078-9. eCollection 2016. BioData Min. 2016. PMID: 26770261 Free PMC article.
-
Pathway-Based Drug-Repurposing Schemes in Cancer: The Role of Translational Bioinformatics.Front Oncol. 2021 Jan 14;10:605680. doi: 10.3389/fonc.2020.605680. eCollection 2020. Front Oncol. 2021. PMID: 33520715 Free PMC article. Review.
-
Basal-like breast cancer: molecular profiles, clinical features and survival outcomes.BMC Med Genomics. 2017 Mar 28;10(1):19. doi: 10.1186/s12920-017-0250-9. BMC Med Genomics. 2017. PMID: 28351365 Free PMC article.
-
Extensive Transcriptomic and Genomic Analysis Provides New Insights about Luminal Breast Cancers.PLoS One. 2016 Jun 24;11(6):e0158259. doi: 10.1371/journal.pone.0158259. eCollection 2016. PLoS One. 2016. PMID: 27341628 Free PMC article.
References
-
- Kelly CM, Bernard PS, Krishnamurthy S, Wang B, Ebbert MT, Bastien RR, et al. Agreement in risk prediction between the 21-gene recurrence score assay (Oncotype DX(R)) and the PAM50 breast cancer intrinsic Classifier in early-stage estrogen receptor-positive breast cancer. Oncologist. 2012;17(4):492–498. 10.1634/theoncologist.2012-0007 - DOI - PMC - PubMed
Publication types
MeSH terms
Substances
Grants and funding
LinkOut - more resources
Full Text Sources
Other Literature Sources
Medical
Research Materials
Miscellaneous