Spatial and temporal epidemiological analysis in the Big Data era
- PMID: 26092722
- PMCID: PMC7114113
- DOI: 10.1016/j.prevetmed.2015.05.012
Spatial and temporal epidemiological analysis in the Big Data era
Abstract
Concurrent with global economic development in the last 50 years, the opportunities for the spread of existing diseases and emergence of new infectious pathogens, have increased substantially. The activities associated with the enormously intensified global connectivity have resulted in large amounts of data being generated, which in turn provides opportunities for generating knowledge that will allow more effective management of animal and human health risks. This so-called Big Data has, more recently, been accompanied by the Internet of Things which highlights the increasing presence of a wide range of sensors, interconnected via the Internet. Analysis of this data needs to exploit its complexity, accommodate variation in data quality and should take advantage of its spatial and temporal dimensions, where available. Apart from the development of hardware technologies and networking/communication infrastructure, it is necessary to develop appropriate data management tools that make this data accessible for analysis. This includes relational databases, geographical information systems and most recently, cloud-based data storage such as Hadoop distributed file systems. While the development in analytical methodologies has not quite caught up with the data deluge, important advances have been made in a number of areas, including spatial and temporal data analysis where the spectrum of analytical methods ranges from visualisation and exploratory analysis, to modelling. While there used to be a primary focus on statistical science in terms of methodological development for data analysis, the newly emerged discipline of data science is a reflection of the challenges presented by the need to integrate diverse data sources and exploit them using novel data- and knowledge-driven modelling methods while simultaneously recognising the value of quantitative as well as qualitative analytical approaches. Machine learning regression methods, which are more robust and can handle large datasets faster than classical regression approaches, are now also used to analyse spatial and spatio-temporal data. Multi-criteria decision analysis methods have gained greater acceptance, due in part, to the need to increasingly combine data from diverse sources including published scientific information and expert opinion in an attempt to fill important knowledge gaps. The opportunities for more effective prevention, detection and control of animal health threats arising from these developments are immense, but not without risks given the different types, and much higher frequency, of biases associated with these data.
Keywords: Data science; Exploratory analysis; Internet of Things; Modelling; Multi-criteria decision analysis; Spatial analysis; Visualisation.
Copyright © 2015 Elsevier B.V. All rights reserved.
Figures
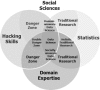
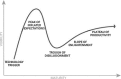
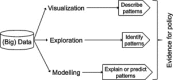
Similar articles
-
Assessment and statistical modeling of the relationship between remotely sensed aerosol optical depth and PM2.5 in the eastern United States.Res Rep Health Eff Inst. 2012 May;(167):5-83; discussion 85-91. Res Rep Health Eff Inst. 2012. PMID: 22838153
-
Adapting a geographical information system-based water resource management to the needs of the Romanian water authorities.Environ Sci Pollut Res Int. 2009 Aug;16 Suppl 1:S33-41. doi: 10.1007/s11356-008-0065-5. Epub 2008 Nov 8. Environ Sci Pollut Res Int. 2009. PMID: 18998185
-
Microbial Ecology and Genomics: A Crossroads of Opportunity: This report is based on a colloquium sponsored by the American Academy of Microbiology held February 23-25, 2001, in Singer Island, Florida.Washington (DC): American Society for Microbiology; 2002. Washington (DC): American Society for Microbiology; 2002. PMID: 33119230 Free Books & Documents. Review.
-
Tuberculosis.In: Holmes KK, Bertozzi S, Bloom BR, Jha P, editors. Major Infectious Diseases. 3rd edition. Washington (DC): The International Bank for Reconstruction and Development / The World Bank; 2017 Nov 3. Chapter 11. In: Holmes KK, Bertozzi S, Bloom BR, Jha P, editors. Major Infectious Diseases. 3rd edition. Washington (DC): The International Bank for Reconstruction and Development / The World Bank; 2017 Nov 3. Chapter 11. PMID: 30212088 Free Books & Documents. Review.
-
The value of information: Current challenges in surveillance implementation.Prev Vet Med. 2015 Nov 1;122(1-2):229-34. doi: 10.1016/j.prevetmed.2015.05.002. Epub 2015 May 14. Prev Vet Med. 2015. PMID: 26021437 Review.
Cited by
-
Temporal and spatial distribution characteristics in the natural plague foci of Chinese Mongolian gerbils based on spatial autocorrelation.Infect Dis Poverty. 2017 Aug 7;6(1):124. doi: 10.1186/s40249-017-0338-7. Infect Dis Poverty. 2017. PMID: 28780908 Free PMC article.
-
Temporal and Spatial Characteristics of Cataract Surgery Rates in China.Risk Manag Healthc Policy. 2021 Aug 25;14:3551-3561. doi: 10.2147/RMHP.S317547. eCollection 2021. Risk Manag Healthc Policy. 2021. PMID: 34475788 Free PMC article.
-
EpiVECS: exploring spatiotemporal epidemiological data using cluster embedding and interactive visualization.Sci Rep. 2023 Dec 1;13(1):21193. doi: 10.1038/s41598-023-48484-9. Sci Rep. 2023. PMID: 38040776 Free PMC article.
-
Translating Big Data into Smart Data for Veterinary Epidemiology.Front Vet Sci. 2017 Jul 17;4:110. doi: 10.3389/fvets.2017.00110. eCollection 2017. Front Vet Sci. 2017. PMID: 28770216 Free PMC article.
-
A Practitioner-Driven Research Agenda for Syndromic Surveillance.Public Health Rep. 2017 Jul/Aug;132(1_suppl):116S-126S. doi: 10.1177/0033354917709784. Public Health Rep. 2017. PMID: 28692395 Free PMC article.
References
-
- Alvarado-Serrano D.F., Knowles L.L. Ecological niche models in phylogeographic studies: applications, advances and precautions. Mol. Ecol. Resources. 2013;14:233–248. - PubMed
-
- Anderson C. The end of theory: the data deluge makes the scientific method obsolete. Wired Mag. 2008;16:07.
-
- Andrienko N., Andrienko G. Visual analytics of movement: an overview of methods, tools and procedures. Inf. Visual. 2012;12:3–24.
-
- Anon . The National Academies Press; Washington DC, USA: 2011. Toward Precision Medicine: Building a Knowledge Network for Biomedical Research and a New Taxonomy of Disease. - PubMed
-
- Anon . National Research Council of the National Academies; Washington DC, USA: 2013. Frontiers in Massive Data Analysis. 176pp.
Publication types
MeSH terms
LinkOut - more resources
Full Text Sources
Other Literature Sources