Identifying module biomarker in type 2 diabetes mellitus by discriminative area of functional activity
- PMID: 25888350
- PMCID: PMC4374500
- DOI: 10.1186/s12859-015-0519-y
Identifying module biomarker in type 2 diabetes mellitus by discriminative area of functional activity
Abstract
Background: Identifying diagnosis and prognosis biomarkers from expression profiling data is of great significance for achieving personalized medicine and designing therapeutic strategy in complex diseases. However, the reproducibility of identified biomarkers across tissues and experiments is still a challenge for this issue.
Results: We propose a strategy based on discriminative area of module activities to identify gene biomarkers which interconnect as a subnetwork or module by integrating gene expression data and protein-protein interactions. Then, we implement the procedure in T2DM as a case study and identify a module biomarker with 32 genes from mRNA expression data in skeletal muscle for T2DM. This module biomarker is enriched with known causal genes and related functions of T2DM. Further analysis shows that the module biomarker is of superior performance in classification, and has consistently high accuracies across tissues and experiments.
Conclusion: The proposed approach can efficiently identify robust and functionally meaningful module biomarkers in T2DM, and could be employed in biomarker discovery of other complex diseases characterized by expression profiles.
Figures
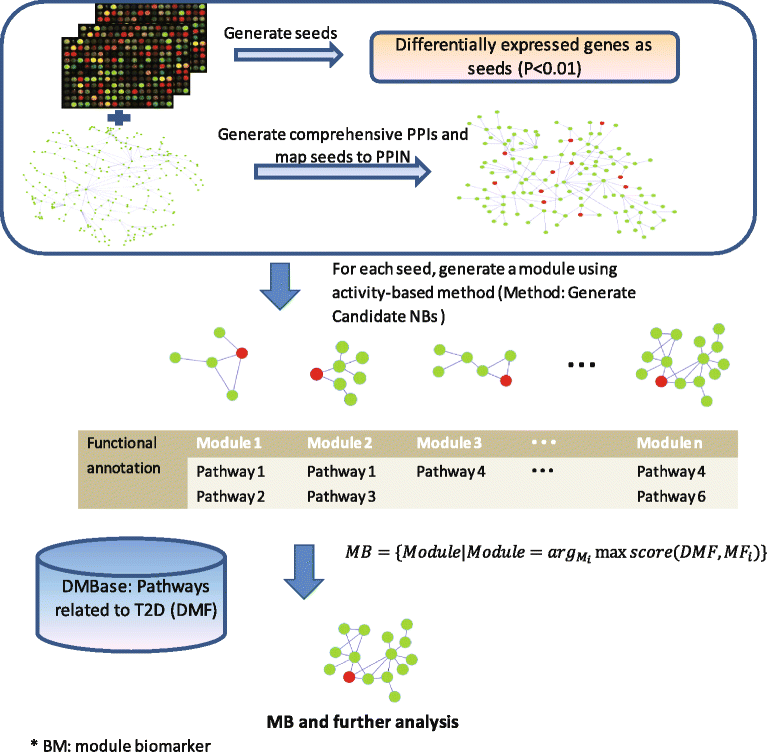
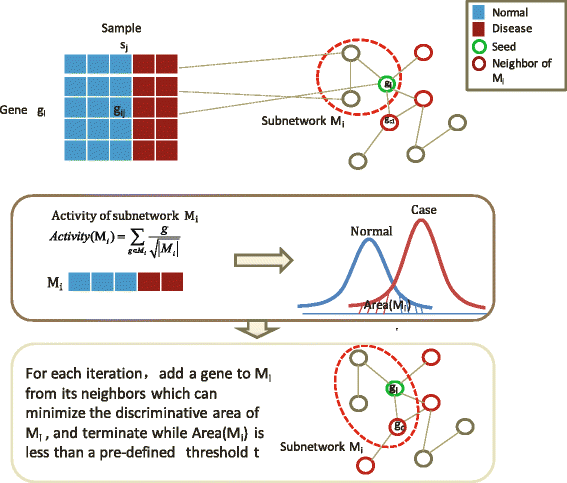
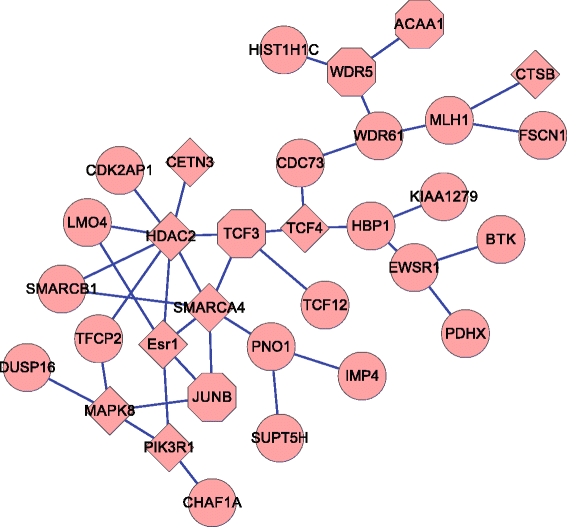
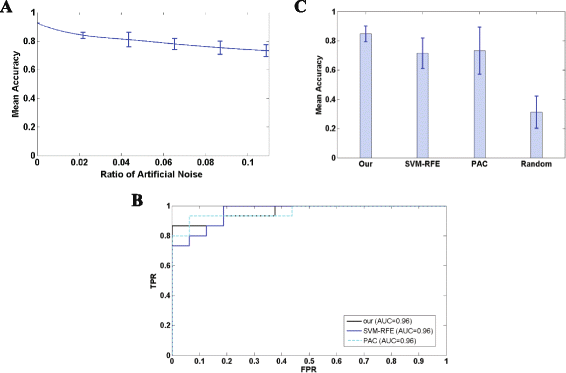
Similar articles
-
Detecting tissue-specific early warning signals for complex diseases based on dynamical network biomarkers: study of type 2 diabetes by cross-tissue analysis.Brief Bioinform. 2014 Mar;15(2):229-43. doi: 10.1093/bib/bbt027. Epub 2013 Apr 25. Brief Bioinform. 2014. PMID: 23620135
-
Tissue-Specific Molecular Biomarker Signatures of Type 2 Diabetes: An Integrative Analysis of Transcriptomics and Protein-Protein Interaction Data.OMICS. 2015 Sep;19(9):563-73. doi: 10.1089/omi.2015.0088. OMICS. 2015. PMID: 26348713
-
The "Metabolic biomarkers of frailty in older people with type 2 diabetes mellitus" (MetaboFrail) study: Rationale, design and methods.Exp Gerontol. 2020 Jan;129:110782. doi: 10.1016/j.exger.2019.110782. Epub 2019 Nov 18. Exp Gerontol. 2020. PMID: 31751663
-
Network-Assisted Disease Classification and Biomarker Discovery.Methods Mol Biol. 2016;1386:353-74. doi: 10.1007/978-1-4939-3283-2_16. Methods Mol Biol. 2016. PMID: 26677191 Review.
-
Functional genomics and proteomics in the clinical neurosciences: data mining and bioinformatics.Prog Brain Res. 2006;158:83-108. doi: 10.1016/S0079-6123(06)58004-5. Prog Brain Res. 2006. PMID: 17027692 Review.
Cited by
-
ModuleDiscoverer: Identification of regulatory modules in protein-protein interaction networks.Sci Rep. 2018 Jan 11;8(1):433. doi: 10.1038/s41598-017-18370-2. Sci Rep. 2018. PMID: 29323246 Free PMC article.
-
Data analysis methods for defining biomarkers from omics data.Anal Bioanal Chem. 2022 Jan;414(1):235-250. doi: 10.1007/s00216-021-03813-7. Epub 2021 Dec 24. Anal Bioanal Chem. 2022. PMID: 34951658 Review.
-
Regularized logistic regression with network-based pairwise interaction for biomarker identification in breast cancer.BMC Bioinformatics. 2016 Feb 27;17:108. doi: 10.1186/s12859-016-0951-7. BMC Bioinformatics. 2016. PMID: 26921029 Free PMC article.
-
Capsaicin and Zinc Promote Glucose Uptake in C2C12 Skeletal Muscle Cells through a Common Calcium Signalling Pathway.Int J Mol Sci. 2022 Feb 17;23(4):2207. doi: 10.3390/ijms23042207. Int J Mol Sci. 2022. PMID: 35216322 Free PMC article.
References
-
- Ripsin CM, Kang H, URban RJ. Management of blood glucose in type 2 diabetes mellitus. Am Fam Physician. 2009;79:29–36. - PubMed
Publication types
MeSH terms
Substances
LinkOut - more resources
Full Text Sources
Other Literature Sources
Medical