HCsnip: An R Package for Semi-supervised Snipping of the Hierarchical Clustering Tree
- PMID: 25861213
- PMCID: PMC4372030
- DOI: 10.4137/CIN.S22080
HCsnip: An R Package for Semi-supervised Snipping of the Hierarchical Clustering Tree
Abstract
Hierarchical clustering (HC) is one of the most frequently used methods in computational biology in the analysis of high-dimensional genomics data. Given a data set, HC outputs a binary tree leaves of which are the data points and internal nodes represent clusters of various sizes. Normally, a fixed-height cut on the HC tree is chosen, and each contiguous branch of data points below that height is considered as a separate cluster. However, the fixed-height branch cut may not be ideal in situations where one expects a complicated tree structure with nested clusters. Furthermore, due to lack of utilization of related background information in selecting the cutoff, induced clusters are often difficult to interpret. This paper describes a novel procedure that aims to automatically extract meaningful clusters from the HC tree in a semi-supervised way. The procedure is implemented in the R package HCsnip available from Bioconductor. Rather than cutting the HC tree at a fixed-height, HCsnip probes the various way of snipping, possibly at variable heights, to tease out hidden clusters ensconced deep down in the tree. The cluster extraction process utilizes, along with the data set from which the HC tree is derived, commonly available background information. Consequently, the extracted clusters are highly reproducible and robust against various sources of variations that "haunted" high-dimensional genomics data. Since the clustering process is guided by the background information, clusters are easy to interpret. Unlike existing packages, no constraint is placed on the data type on which clustering is desired. Particularly, the package accepts patient follow-up data for guiding the cluster extraction process. To our knowledge, HCsnip is the first package that is able to decomposes the HC tree into clusters with piecewise snipping under the guidance of patient time-to-event information. Our implementation of the semi-supervised HC tree snipping framework is generic, and can be combined with other algorithms that operate on detected clusters.
Keywords: R package; data integration; hierarchical clustering; high-dimensional data; semi-supervised clustering.
Figures
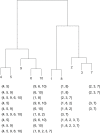
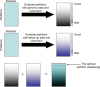
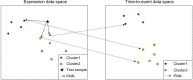
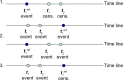
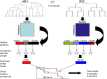
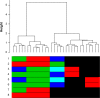
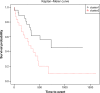
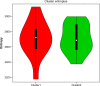
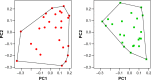
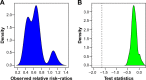
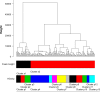
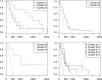
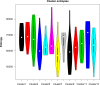
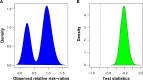
Similar articles
-
Semi-supervised adaptive-height snipping of the hierarchical clustering tree.BMC Bioinformatics. 2015 Jan 16;16(1):15. doi: 10.1186/s12859-014-0448-1. BMC Bioinformatics. 2015. PMID: 25592847 Free PMC article.
-
Defining clusters from a hierarchical cluster tree: the Dynamic Tree Cut package for R.Bioinformatics. 2008 Mar 1;24(5):719-20. doi: 10.1093/bioinformatics/btm563. Epub 2007 Nov 16. Bioinformatics. 2008. PMID: 18024473
-
Fast tree aggregation for consensus hierarchical clustering.BMC Bioinformatics. 2020 Mar 20;21(1):120. doi: 10.1186/s12859-020-3453-6. BMC Bioinformatics. 2020. PMID: 32197576 Free PMC article.
-
Hierarchical tree snipping: clustering guided by prior knowledge.Bioinformatics. 2007 Dec 15;23(24):3335-42. doi: 10.1093/bioinformatics/btm526. Epub 2007 Nov 7. Bioinformatics. 2007. PMID: 17989094
-
multiClust: An R-package for Identifying Biologically Relevant Clusters in Cancer Transcriptome Profiles.Cancer Inform. 2016 Jun 12;15:103-14. doi: 10.4137/CIN.S38000. eCollection 2016. Cancer Inform. 2016. PMID: 27330269 Free PMC article. Review.
References
Publication types
LinkOut - more resources
Full Text Sources