Stability, structure and scale: improvements in multi-modal vessel extraction for SEEG trajectory planning
- PMID: 25847663
- PMCID: PMC4523698
- DOI: 10.1007/s11548-015-1174-5
Stability, structure and scale: improvements in multi-modal vessel extraction for SEEG trajectory planning
Abstract
Purpose: Brain vessels are among the most critical landmarks that need to be assessed for mitigating surgical risks in stereo-electroencephalography (SEEG) implantation. Intracranial haemorrhage is the most common complication associated with implantation, carrying significantly associated morbidity. SEEG planning is done pre-operatively to identify avascular trajectories for the electrodes. In current practice, neurosurgeons have no assistance in the planning of electrode trajectories. There is great interest in developing computer-assisted planning systems that can optimise the safety profile of electrode trajectories, maximising the distance to critical structures. This paper presents a method that integrates the concepts of scale, neighbourhood structure and feature stability with the aim of improving robustness and accuracy of vessel extraction within a SEEG planning system.
Methods: The developed method accounts for scale and vicinity of a voxel by formulating the problem within a multi-scale tensor voting framework. Feature stability is achieved through a similarity measure that evaluates the multi-modal consistency in vesselness responses. The proposed measurement allows the combination of multiple images modalities into a single image that is used within the planning system to visualise critical vessels.
Results: Twelve paired data sets from two image modalities available within the planning system were used for evaluation. The mean Dice similarity coefficient was 0.89 ± 0.04, representing a statistically significantly improvement when compared to a semi-automated single human rater, single-modality segmentation protocol used in clinical practice (0.80 ± 0.03).
Conclusions: Multi-modal vessel extraction is superior to semi-automated single-modality segmentation, indicating the possibility of safer SEEG planning, with reduced patient morbidity.
Figures
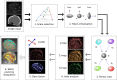
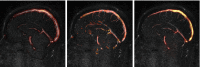
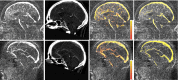
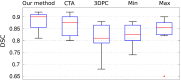
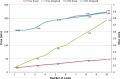
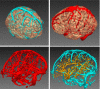
Similar articles
-
SEEG trajectory planning: combining stability, structure and scale in vessel extraction.Med Image Comput Comput Assist Interv. 2014;17(Pt 2):651-8. doi: 10.1007/978-3-319-10470-6_81. Med Image Comput Comput Assist Interv. 2014. PMID: 25485435
-
Automated multiple trajectory planning algorithm for the placement of stereo-electroencephalography (SEEG) electrodes in epilepsy treatment.Int J Comput Assist Radiol Surg. 2017 Jan;12(1):123-136. doi: 10.1007/s11548-016-1452-x. Epub 2016 Jul 1. Int J Comput Assist Radiol Surg. 2017. PMID: 27368184 Free PMC article.
-
Multi-trajectories automatic planner for StereoElectroEncephaloGraphy (SEEG).Int J Comput Assist Radiol Surg. 2014 Nov;9(6):1087-97. doi: 10.1007/s11548-014-1004-1. Epub 2014 Apr 20. Int J Comput Assist Radiol Surg. 2014. PMID: 24748210
-
Automation Advances in Stereoelectroencephalography Planning.Neurosurg Clin N Am. 2020 Jul;31(3):407-419. doi: 10.1016/j.nec.2020.03.005. Epub 2020 Apr 23. Neurosurg Clin N Am. 2020. PMID: 32475489 Free PMC article. Review.
-
Stereoelectroencephalography: Indication and Efficacy.Neurol Med Chir (Tokyo). 2017 Aug 15;57(8):375-385. doi: 10.2176/nmc.ra.2017-0008. Epub 2017 Jun 20. Neurol Med Chir (Tokyo). 2017. PMID: 28637943 Free PMC article. Review.
Cited by
-
Anatomy-driven multiple trajectory planning (ADMTP) of intracranial electrodes for epilepsy surgery.Int J Comput Assist Radiol Surg. 2017 Aug;12(8):1245-1255. doi: 10.1007/s11548-017-1628-z. Epub 2017 Jun 15. Int J Comput Assist Radiol Surg. 2017. PMID: 28620830 Free PMC article.
-
Inference of Cerebrovascular Topology With Geodesic Minimum Spanning Trees.IEEE Trans Med Imaging. 2019 Jan;38(1):225-239. doi: 10.1109/TMI.2018.2860239. Epub 2018 Jul 26. IEEE Trans Med Imaging. 2019. PMID: 30059296 Free PMC article.
-
Accuracy of intracranial electrode placement for stereoencephalography: A systematic review and meta-analysis.Epilepsia. 2017 Jun;58(6):921-932. doi: 10.1111/epi.13713. Epub 2017 Mar 6. Epilepsia. 2017. PMID: 28261785 Free PMC article. Review.
-
Interactive virtual 3D models of renal cancer patient anatomies alter partial nephrectomy surgical planning decisions and increase surgeon confidence compared to volume-rendered images.Int J Comput Assist Radiol Surg. 2019 Apr;14(4):723-732. doi: 10.1007/s11548-019-01913-5. Epub 2019 Jan 24. Int J Comput Assist Radiol Surg. 2019. PMID: 30680601 Free PMC article.
-
Multicenter validation of automated trajectories for selective laser amygdalohippocampectomy.Epilepsia. 2019 Sep;60(9):1949-1959. doi: 10.1111/epi.16307. Epub 2019 Aug 7. Epilepsia. 2019. PMID: 31392717 Free PMC article.
References
-
- Cossu M, Cardinale F, Castana L, Citterio A, Francione S, Tassi L, Benabid ALM, Lo Russo G. Stereoelectroencephalography in the presurgical evaluation of focal epilepsy: a retrospective analysis of 215 procedures. Neurosurgery. 2005;57(4):706–718. doi: 10.1227/01.NEU.0000176656.33523.1e. - DOI - PubMed
Publication types
MeSH terms
Grants and funding
LinkOut - more resources
Full Text Sources
Other Literature Sources