A novel mathematical model describing adaptive cellular drug metabolism and toxicity in the chemoimmune system
- PMID: 25699998
- PMCID: PMC4338831
- DOI: 10.1371/journal.pone.0115533
A novel mathematical model describing adaptive cellular drug metabolism and toxicity in the chemoimmune system
Abstract
Cells cope with the threat of xenobiotic stress by activating a complex molecular network that recognizes and eliminates chemically diverse toxic compounds. This "chemoimmune system" consists of cellular Phase I and Phase II metabolic enzymes, Phase 0 and Phase III ATP Binding Cassette (ABC) membrane transporters, and nuclear receptors regulating these components. In order to provide a systems biology characterization of the chemoimmune network, we designed a reaction kinetic model based on differential equations describing Phase 0-III participants and regulatory elements, and characterized cellular fitness to evaluate toxicity. In spite of the simplifications, the model recapitulates changes associated with acquired drug resistance and allows toxicity predictions under variable protein expression and xenobiotic exposure conditions. Our simulations suggest that multidrug ABC transporters at Phase 0 significantly facilitate the defense function of successive network members by lowering intracellular drug concentrations. The model was extended with a novel toxicity framework which opened the possibility of performing in silico cytotoxicity assays. The alterations of the in silico cytotoxicity curves show good agreement with in vitro cell killing experiments. The behavior of the simplified kinetic model suggests that it can serve as a basis for more complex models to efficiently predict xenobiotic and drug metabolism for human medical applications.
Conflict of interest statement
Figures
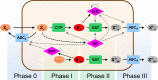
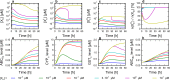
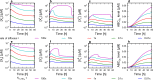
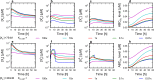
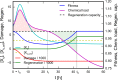
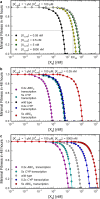
Similar articles
-
The role of ABC transporters in drug resistance, metabolism and toxicity.Curr Drug Deliv. 2004 Jan;1(1):27-42. doi: 10.2174/1567201043480036. Curr Drug Deliv. 2004. PMID: 16305368 Review.
-
Activity of the dietary flavonoid, apigenin, against multidrug-resistant tumor cells as determined by pharmacogenomics and molecular docking.J Nutr Biochem. 2015 Jan;26(1):44-56. doi: 10.1016/j.jnutbio.2014.09.008. Epub 2014 Oct 13. J Nutr Biochem. 2015. PMID: 25459885
-
Differential expression and functionality of ATP-binding cassette transporters in the human hair follicle.Br J Dermatol. 2015 Jun;172(6):1562-1572. doi: 10.1111/bjd.13549. Epub 2015 Mar 19. Br J Dermatol. 2015. PMID: 25418064
-
Multidrug resistance proteins: role of P-glycoprotein, MRP1, MRP2, and BCRP (ABCG2) in tissue defense.Toxicol Appl Pharmacol. 2005 May 1;204(3):216-37. doi: 10.1016/j.taap.2004.10.012. Toxicol Appl Pharmacol. 2005. PMID: 15845415 Review.
-
The role of multidrug resistance efflux transporters in antifolate resistance and folate homeostasis.Drug Resist Updat. 2006 Aug-Oct;9(4-5):227-46. doi: 10.1016/j.drup.2006.09.001. Epub 2006 Nov 7. Drug Resist Updat. 2006. PMID: 17092765 Review.
Cited by
-
Glutathione S-Transferase M3 Is Associated with Glycolysis in Intrinsic Temozolomide-Resistant Glioblastoma Multiforme Cells.Int J Mol Sci. 2021 Jun 30;22(13):7080. doi: 10.3390/ijms22137080. Int J Mol Sci. 2021. PMID: 34209254 Free PMC article.
-
GLUL gene knockdown and restricted glucose level show synergistic inhibitory effect on the luminal subtype breast cancer MCF7 cells' proliferation and metastasis.EXCLI J. 2023 Aug 16;22:847-861. doi: 10.17179/excli2023-6287. eCollection 2023. EXCLI J. 2023. PMID: 37780942 Free PMC article.
-
Mathematical Models for Immunology: Current State of the Art and Future Research Directions.Bull Math Biol. 2016 Oct;78(10):2091-2134. doi: 10.1007/s11538-016-0214-9. Epub 2016 Oct 6. Bull Math Biol. 2016. PMID: 27714570 Free PMC article. Review.
References
-
- Sarkadi B, Homolya L, Szakacs G, Varadi A (2006) Human multidrug resistance ABCB and ABCG transporters: participation in a chemoimmunity defense system. Physiological reviews 86: 1179–1236. - PubMed
Publication types
MeSH terms
Substances
Grants and funding
LinkOut - more resources
Full Text Sources
Other Literature Sources