Evolutionary triage governs fitness in driver and passenger mutations and suggests targeting never mutations
- PMID: 25407411
- PMCID: PMC4260773
- DOI: 10.1038/ncomms6499
Evolutionary triage governs fitness in driver and passenger mutations and suggests targeting never mutations
Abstract
Genetic and epigenetic changes in cancer cells are typically divided into 'drivers' and 'passengers'. Drug development strategies target driver mutations, but inter- and intratumoral heterogeneity usually results in emergence of resistance. Here we model intratumoral evolution in the context of a fecundity/survivorship trade-off. Simulations demonstrate that the fitness value of any genetic change is not fixed but dependent on evolutionary triage governed by initial cell properties, current selection forces and prior genotypic/phenotypic trajectories. We demonstrate that spatial variations in molecular properties of tumour cells are the result of changes in environmental selection forces such as blood flow. Simulated therapies targeting fitness-increasing (driver) mutations usually decrease the tumour burden but almost inevitably fail due to population heterogeneity. An alternative strategy targets gene mutations that are never observed. Because up or downregulation of these genes unconditionally reduces cellular fitness, they are eliminated by evolutionary triage but can be exploited for targeted therapy.
Conflict of interest statement
The authors declare no competing financial interests
Figures
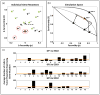
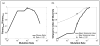
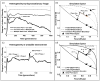
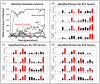
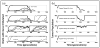
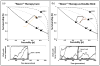
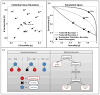
Similar articles
-
Mutations, evolution and the central role of a self-defined fitness function in the initiation and progression of cancer.Biochim Biophys Acta Rev Cancer. 2017 Apr;1867(2):162-166. doi: 10.1016/j.bbcan.2017.03.005. Epub 2017 Mar 21. Biochim Biophys Acta Rev Cancer. 2017. PMID: 28341421 Free PMC article. Review.
-
Passenger mutations can accelerate tumour suppressor gene inactivation in cancer evolution.J R Soc Interface. 2018 Jun;15(143):20170967. doi: 10.1098/rsif.2017.0967. J R Soc Interface. 2018. PMID: 29875280 Free PMC article.
-
Baldwin effect under multipeaked fitness landscapes: phenotypic fluctuation accelerates evolutionary rate.Phys Rev E Stat Nonlin Soft Matter Phys. 2013 May;87(5):052701. doi: 10.1103/PhysRevE.87.052701. Epub 2013 May 2. Phys Rev E Stat Nonlin Soft Matter Phys. 2013. PMID: 23767560
-
Somatic clonal evolution: A selection-centric perspective.Biochim Biophys Acta Rev Cancer. 2017 Apr;1867(2):139-150. doi: 10.1016/j.bbcan.2017.01.006. Epub 2017 Feb 2. Biochim Biophys Acta Rev Cancer. 2017. PMID: 28161395 Review.
-
Evolutionary dynamics of tumor progression with random fitness values.Theor Popul Biol. 2010 Aug;78(1):54-66. doi: 10.1016/j.tpb.2010.05.001. Epub 2010 May 19. Theor Popul Biol. 2010. PMID: 20488197 Free PMC article.
Cited by
-
Genotyping of circulating tumor DNA in cholangiocarcinoma reveals diagnostic and prognostic information.Sci Rep. 2019 Sep 13;9(1):13261. doi: 10.1038/s41598-019-49860-0. Sci Rep. 2019. PMID: 31519967 Free PMC article.
-
Exploiting evolutionary principles to prolong tumor control in preclinical models of breast cancer.Sci Transl Med. 2016 Feb 24;8(327):327ra24. doi: 10.1126/scitranslmed.aad7842. Sci Transl Med. 2016. PMID: 26912903 Free PMC article.
-
Darwinian Dynamics of Intratumoral Heterogeneity: Not Solely Random Mutations but Also Variable Environmental Selection Forces.Cancer Res. 2016 Jun 1;76(11):3136-44. doi: 10.1158/0008-5472.CAN-15-2962. Epub 2016 Mar 23. Cancer Res. 2016. PMID: 27009166 Free PMC article.
-
Toward an evolutionary model of cancer: Considering the mechanisms that govern the fate of somatic mutations.Proc Natl Acad Sci U S A. 2015 Jul 21;112(29):8914-21. doi: 10.1073/pnas.1501713112. Proc Natl Acad Sci U S A. 2015. PMID: 26195756 Free PMC article.
-
Evolutionary selection identifies critical immune-relevant genes in lung cancer subtypes.Front Genet. 2022 Aug 24;13:921447. doi: 10.3389/fgene.2022.921447. eCollection 2022. Front Genet. 2022. PMID: 36092893 Free PMC article.
References
-
- Nowell PC. The clonal evolution of tumor cell populations. Science. 1976;194:23–8. - PubMed
-
- Merlo LM, Pepper JW, Reid BJ, Maley CC. Cancer as an evolutionary and ecological process. Nature Rev Cancer. 2006;812:924–35. - PubMed
-
- Komarova NL, Sengupta A, Nowak MA. Mutation-selection networks in cancer initiation: tumor suppressor genes and chromosomal instability. J Theor Biol. 2003;223:433–50. - PubMed
-
- Hanahan D, Weinberg RA. Hallmarks of cancer: the next generation. Cell. 2011;144:646–674. - PubMed
Publication types
MeSH terms
Grants and funding
LinkOut - more resources
Full Text Sources
Other Literature Sources