Power analysis and sample size estimation for RNA-Seq differential expression
- PMID: 25246651
- PMCID: PMC4201821
- DOI: 10.1261/rna.046011.114
Power analysis and sample size estimation for RNA-Seq differential expression
Abstract
It is crucial for researchers to optimize RNA-seq experimental designs for differential expression detection. Currently, the field lacks general methods to estimate power and sample size for RNA-Seq in complex experimental designs, under the assumption of the negative binomial distribution. We simulate RNA-Seq count data based on parameters estimated from six widely different public data sets (including cell line comparison, tissue comparison, and cancer data sets) and calculate the statistical power in paired and unpaired sample experiments. We comprehensively compare five differential expression analysis packages (DESeq, edgeR, DESeq2, sSeq, and EBSeq) and evaluate their performance by power, receiver operator characteristic (ROC) curves, and other metrics including areas under the curve (AUC), Matthews correlation coefficient (MCC), and F-measures. DESeq2 and edgeR tend to give the best performance in general. Increasing sample size or sequencing depth increases power; however, increasing sample size is more potent than sequencing depth to increase power, especially when the sequencing depth reaches 20 million reads. Long intergenic noncoding RNAs (lincRNA) yields lower power relative to the protein coding mRNAs, given their lower expression level in the same RNA-Seq experiment. On the other hand, paired-sample RNA-Seq significantly enhances the statistical power, confirming the importance of considering the multifactor experimental design. Finally, a local optimal power is achievable for a given budget constraint, and the dominant contributing factor is sample size rather than the sequencing depth. In conclusion, we provide a power analysis tool (http://www2.hawaii.edu/~lgarmire/RNASeqPowerCalculator.htm) that captures the dispersion in the data and can serve as a practical reference under the budget constraint of RNA-Seq experiments.
Keywords: RNA-Seq; bioinformatics; power analysis; sample size; simulation.
© 2014 Ching et al.; Published by Cold Spring Harbor Laboratory Press for the RNA Society.
Figures
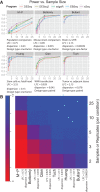
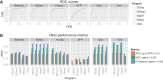
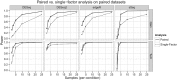
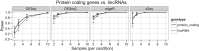
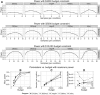
Similar articles
-
An evaluation of RNA-seq differential analysis methods.PLoS One. 2022 Sep 16;17(9):e0264246. doi: 10.1371/journal.pone.0264246. eCollection 2022. PLoS One. 2022. PMID: 36112652 Free PMC article.
-
A comparison of per sample global scaling and per gene normalization methods for differential expression analysis of RNA-seq data.PLoS One. 2017 May 1;12(5):e0176185. doi: 10.1371/journal.pone.0176185. eCollection 2017. PLoS One. 2017. PMID: 28459823 Free PMC article.
-
Benchmarking differential expression analysis tools for RNA-Seq: normalization-based vs. log-ratio transformation-based methods.BMC Bioinformatics. 2018 Jul 18;19(1):274. doi: 10.1186/s12859-018-2261-8. BMC Bioinformatics. 2018. PMID: 30021534 Free PMC article.
-
Differential Expression Analysis of RNA-seq Reads: Overview, Taxonomy, and Tools.IEEE/ACM Trans Comput Biol Bioinform. 2020 Mar-Apr;17(2):566-586. doi: 10.1109/TCBB.2018.2873010. Epub 2018 Oct 1. IEEE/ACM Trans Comput Biol Bioinform. 2020. PMID: 30281477 Review.
-
The power and promise of RNA-seq in ecology and evolution.Mol Ecol. 2016 Mar;25(6):1224-41. doi: 10.1111/mec.13526. Epub 2016 Mar 1. Mol Ecol. 2016. PMID: 26756714 Review.
Cited by
-
RNA-Seq reveals 10 novel promising candidate genes affecting milk protein concentration in the Chinese Holstein population.Sci Rep. 2016 Jun 2;6:26813. doi: 10.1038/srep26813. Sci Rep. 2016. PMID: 27254118 Free PMC article.
-
Meta-dimensional data integration identifies critical pathways for susceptibility, tumorigenesis and progression of endometrial cancer.Oncotarget. 2016 Aug 23;7(34):55249-55263. doi: 10.18632/oncotarget.10509. Oncotarget. 2016. PMID: 27409342 Free PMC article.
-
Pan-Cancer Analyses Reveal Long Intergenic Non-Coding RNAs Relevant to Tumor Diagnosis, Subtyping and Prognosis.EBioMedicine. 2016 May;7:62-72. doi: 10.1016/j.ebiom.2016.03.023. Epub 2016 Mar 19. EBioMedicine. 2016. PMID: 27322459 Free PMC article.
-
A comprehensive method protocol for annotation and integrated functional understanding of lncRNAs.Brief Bioinform. 2020 Jul 15;21(4):1391-1396. doi: 10.1093/bib/bbz066. Brief Bioinform. 2020. PMID: 31578571 Free PMC article.
-
Intrinsically dysregulated cellular stress signaling genes and gene networks in postpartum depression.Mol Psychiatry. 2023 Jul;28(7):3023-3032. doi: 10.1038/s41380-023-01985-5. Epub 2023 Feb 13. Mol Psychiatry. 2023. PMID: 36782063 Free PMC article.
References
Publication types
MeSH terms
Substances
Grants and funding
LinkOut - more resources
Full Text Sources
Other Literature Sources