Computational prediction and validation of an expert's evaluation of chemical probes
- PMID: 25244007
- PMCID: PMC4955571
- DOI: 10.1021/ci500445u
Computational prediction and validation of an expert's evaluation of chemical probes
Abstract
In a decade with over half a billion dollars of investment, more than 300 chemical probes have been identified to have biological activity through NIH funded screening efforts. We have collected the evaluations of an experienced medicinal chemist on the likely chemistry quality of these probes based on a number of criteria including literature related to the probe and potential chemical reactivity. Over 20% of these probes were found to be undesirable. Analysis of the molecular properties of these compounds scored as desirable suggested higher pKa, molecular weight, heavy atom count, and rotatable bond number. We were particularly interested whether the human evaluation aspect of medicinal chemistry due diligence could be computationally predicted. We used a process of sequential Bayesian model building and iterative testing as we included additional probes. Following external validation of these methods and comparing different machine learning methods, we identified Bayesian models with accuracy comparable to other measures of drug-likeness and filtering rules created to date.
Figures
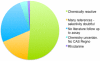
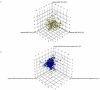
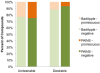
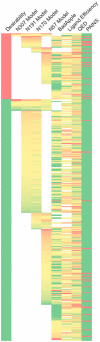
Similar articles
-
Drug-likeness analysis of traditional Chinese medicines: prediction of drug-likeness using machine learning approaches.Mol Pharm. 2012 Oct 1;9(10):2875-86. doi: 10.1021/mp300198d. Epub 2012 Sep 20. Mol Pharm. 2012. PMID: 22738405
-
Evaluating machine learning approaches for aiding probe selection for gene-expression arrays.Bioinformatics. 2002;18 Suppl 1:S164-71. doi: 10.1093/bioinformatics/18.suppl_1.s164. Bioinformatics. 2002. PMID: 12169544
-
Objective, Quantitative, Data-Driven Assessment of Chemical Probes.Cell Chem Biol. 2018 Feb 15;25(2):194-205.e5. doi: 10.1016/j.chembiol.2017.11.004. Epub 2017 Dec 14. Cell Chem Biol. 2018. PMID: 29249694 Free PMC article.
-
Advances in machine learning prediction of toxicological properties and adverse drug reactions of pharmaceutical agents.Curr Drug Saf. 2008 May;3(2):100-14. doi: 10.2174/157488608784529224. Curr Drug Saf. 2008. PMID: 18690988 Review.
-
Computational chemical biology on the rise.Future Med Chem. 2019 Jan;11(1):1-3. doi: 10.4155/fmc-2018-0282. Epub 2018 Dec 10. Future Med Chem. 2019. PMID: 30526065 Review. No abstract available.
Cited by
-
Open Source Bayesian Models. 3. Composite Models for Prediction of Binned Responses.J Chem Inf Model. 2016 Feb 22;56(2):275-85. doi: 10.1021/acs.jcim.5b00555. Epub 2016 Jan 19. J Chem Inf Model. 2016. PMID: 26750305 Free PMC article.
-
Comparison of Deep Learning With Multiple Machine Learning Methods and Metrics Using Diverse Drug Discovery Data Sets.Mol Pharm. 2017 Dec 4;14(12):4462-4475. doi: 10.1021/acs.molpharmaceut.7b00578. Epub 2017 Nov 13. Mol Pharm. 2017. PMID: 29096442 Free PMC article.
-
Small molecules with antiviral activity against the Ebola virus.F1000Res. 2015 Feb 9;4:38. doi: 10.12688/f1000research.6120.1. eCollection 2015. F1000Res. 2015. PMID: 25713700 Free PMC article. Review.
-
Parallel worlds of public and commercial bioactive chemistry data.J Med Chem. 2015 Mar 12;58(5):2068-76. doi: 10.1021/jm5011308. Epub 2014 Dec 4. J Med Chem. 2015. PMID: 25415348 Free PMC article.
-
Will the chemical probes please stand up?RSC Med Chem. 2021 Jul 16;12(8):1428-1441. doi: 10.1039/d1md00138h. eCollection 2021 Aug 18. RSC Med Chem. 2021. PMID: 34447939 Free PMC article.
References
-
- Kaiser J. National Institutes of Health. Drug-screening program looking for a home. Science. 2011;334:299. - PubMed
-
- Jarvis L. Anatomy of an academic drug discovery program. Chemistry & Engineering News. 2014 Jan 20;:28–29.
MeSH terms
Substances
Grants and funding
LinkOut - more resources
Full Text Sources
Other Literature Sources