Big data in chemical toxicity research: the use of high-throughput screening assays to identify potential toxicants
- PMID: 25195622
- PMCID: PMC4203392
- DOI: 10.1021/tx500145h
Big data in chemical toxicity research: the use of high-throughput screening assays to identify potential toxicants
Abstract
High-throughput screening (HTS) assays that measure the in vitro toxicity of environmental compounds have been widely applied as an alternative to in vivo animal tests of chemical toxicity. Current HTS studies provide the community with rich toxicology information that has the potential to be integrated into toxicity research. The available in vitro toxicity data is updated daily in structured formats (e.g., deposited into PubChem and other data-sharing web portals) or in an unstructured way (papers, laboratory reports, toxicity Web site updates, etc.). The information derived from the current toxicity data is so large and complex that it becomes difficult to process using available database management tools or traditional data processing applications. For this reason, it is necessary to develop a big data approach when conducting modern chemical toxicity research. In vitro data for a compound, obtained from meaningful bioassays, can be viewed as a response profile that gives detailed information about the compound's ability to affect relevant biological proteins/receptors. This information is critical for the evaluation of complex bioactivities (e.g., animal toxicities) and grows rapidly as big data in toxicology communities. This review focuses mainly on the existing structured in vitro data (e.g., PubChem data sets) as response profiles for compounds of environmental interest (e.g., potential human/animal toxicants). Potential modeling and mining tools to use the current big data pool in chemical toxicity research are also described.
Figures
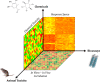
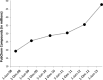
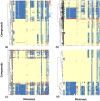
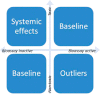
Similar articles
-
Mechanism Profiling of Hepatotoxicity Caused by Oxidative Stress Using Antioxidant Response Element Reporter Gene Assay Models and Big Data.Environ Health Perspect. 2016 May;124(5):634-41. doi: 10.1289/ehp.1509763. Epub 2015 Sep 18. Environ Health Perspect. 2016. PMID: 26383846 Free PMC article.
-
Profiling animal toxicants by automatically mining public bioassay data: a big data approach for computational toxicology.PLoS One. 2014 Jun 20;9(6):e99863. doi: 10.1371/journal.pone.0099863. eCollection 2014. PLoS One. 2014. PMID: 24950175 Free PMC article.
-
Mechanism-Driven Read-Across of Chemical Hepatotoxicants Based on Chemical Structures and Biological Data.Toxicol Sci. 2020 Apr 1;174(2):178-188. doi: 10.1093/toxsci/kfaa005. Toxicol Sci. 2020. PMID: 32073637 Free PMC article.
-
Safety and nutritional assessment of GM plants and derived food and feed: the role of animal feeding trials.Food Chem Toxicol. 2008 Mar;46 Suppl 1:S2-70. doi: 10.1016/j.fct.2008.02.008. Epub 2008 Feb 13. Food Chem Toxicol. 2008. PMID: 18328408 Review.
-
Quantitative in vitro-to-in vivo extrapolation in a high-throughput environment.Toxicology. 2015 Jun 5;332:94-101. doi: 10.1016/j.tox.2014.05.012. Epub 2014 Jun 5. Toxicology. 2015. PMID: 24907440 Review.
Cited by
-
Identification of nonmonotonic concentration-responses in Tox21 high-throughput screening estrogen receptor assays.Toxicol Appl Pharmacol. 2022 Oct 1;452:116206. doi: 10.1016/j.taap.2022.116206. Epub 2022 Aug 19. Toxicol Appl Pharmacol. 2022. PMID: 35988584 Free PMC article.
-
Deep Learning-driven research for drug discovery: Tackling Malaria.PLoS Comput Biol. 2020 Feb 18;16(2):e1007025. doi: 10.1371/journal.pcbi.1007025. eCollection 2020 Feb. PLoS Comput Biol. 2020. PMID: 32069285 Free PMC article.
-
Data-Driven Quantitative Structure-Activity Relationship Modeling for Human Carcinogenicity by Chronic Oral Exposure.Environ Sci Technol. 2023 Apr 25;57(16):6573-6588. doi: 10.1021/acs.est.3c00648. Epub 2023 Apr 11. Environ Sci Technol. 2023. PMID: 37040559 Free PMC article.
-
Experimental Errors in QSAR Modeling Sets: What We Can Do and What We Cannot Do.ACS Omega. 2017 Jun 30;2(6):2805-2812. doi: 10.1021/acsomega.7b00274. Epub 2017 Jun 19. ACS Omega. 2017. PMID: 28691113 Free PMC article.
-
Bioactivity Comparison across Multiple Machine Learning Algorithms Using over 5000 Datasets for Drug Discovery.Mol Pharm. 2021 Jan 4;18(1):403-415. doi: 10.1021/acs.molpharmaceut.0c01013. Epub 2020 Dec 16. Mol Pharm. 2021. PMID: 33325717 Free PMC article.
References
-
- Patel D. V.; Gordon E. M. (1996) Applications of small-molecule combinatorial chemistry to drug discovery. Drug Discovery Today 1, 134–144.
-
- Schreiber S. L. (2000) Target-oriented and diversity-oriented organic synthesis in drug discovery. Science 287, 1964–1969. - PubMed
-
- Malo N.; Hanley J. A.; Cerquozzi S.; Pelletier J.; Nadon R. (2006) Statistical practice in high-throughput screening data analysis. Nat. Biotechnol. 24, 167–175. - PubMed
-
- Inglese J.; Auld D. S.; Jadhav A.; Johnson R. L.; Simeonov A.; Yasgar A.; Zheng W.; Austin C. P. (2006) Quantitative high-throughput screening: a titration-based approach that efficiently identifies biological activities in large chemical libraries. Proc. Natl. Acad. Sci. U.S.A. 103, 11473–11478. - PMC - PubMed
-
- Committee on Toxicity Testing and Assessment of Environmental Agents, N.R.C. (2007) Toxicity testing in the 21st century: a vision and a strategy, The National Academies Press, Washington, DC.
Publication types
MeSH terms
Substances
Grants and funding
LinkOut - more resources
Full Text Sources
Other Literature Sources