Identifying the gene signatures from gene-pathway bipartite network guarantees the robust model performance on predicting the cancer prognosis
- PMID: 25126556
- PMCID: PMC4122106
- DOI: 10.1155/2014/424509
Identifying the gene signatures from gene-pathway bipartite network guarantees the robust model performance on predicting the cancer prognosis
Abstract
For the purpose of improving the prediction of cancer prognosis in the clinical researches, various algorithms have been developed to construct the predictive models with the gene signatures detected by DNA microarrays. Due to the heterogeneity of the clinical samples, the list of differentially expressed genes (DEGs) generated by the statistical methods or the machine learning algorithms often involves a number of false positive genes, which are not associated with the phenotypic differences between the compared clinical conditions, and subsequently impacts the reliability of the predictive models. In this study, we proposed a strategy, which combined the statistical algorithm with the gene-pathway bipartite networks, to generate the reliable lists of cancer-related DEGs and constructed the models by using support vector machine for predicting the prognosis of three types of cancers, namely, breast cancer, acute myeloma leukemia, and glioblastoma. Our results demonstrated that, combined with the gene-pathway bipartite networks, our proposed strategy can efficiently generate the reliable cancer-related DEG lists for constructing the predictive models. In addition, the model performance in the swap analysis was similar to that in the original analysis, indicating the robustness of the models in predicting the cancer outcomes.
Figures
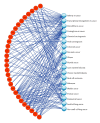
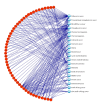
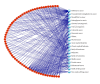
Similar articles
-
Improving the prediction of chemotherapeutic sensitivity of tumors in breast cancer via optimizing the selection of candidate genes.Comput Biol Chem. 2014 Apr;49:71-8. doi: 10.1016/j.compbiolchem.2013.12.002. Epub 2014 Jan 1. Comput Biol Chem. 2014. PMID: 24440656
-
Thousands of samples are needed to generate a robust gene list for predicting outcome in cancer.Proc Natl Acad Sci U S A. 2006 Apr 11;103(15):5923-8. doi: 10.1073/pnas.0601231103. Epub 2006 Apr 3. Proc Natl Acad Sci U S A. 2006. PMID: 16585533 Free PMC article.
-
A 21‑gene Support Vector Machine classifier and a 10‑gene risk score system constructed for patients with gastric cancer.Mol Med Rep. 2020 Jan;21(1):347-359. doi: 10.3892/mmr.2019.10841. Epub 2019 Nov 21. Mol Med Rep. 2020. PMID: 31939629 Free PMC article.
-
Expression genomics in breast cancer research: microarrays at the crossroads of biology and medicine.Breast Cancer Res. 2007;9(2):206. doi: 10.1186/bcr1662. Breast Cancer Res. 2007. PMID: 17397520 Free PMC article. Review.
-
Diagnostic classification of cancer using DNA microarrays and artificial intelligence.Ann N Y Acad Sci. 2004 May;1020:49-66. doi: 10.1196/annals.1310.007. Ann N Y Acad Sci. 2004. PMID: 15208183 Review.
Cited by
-
Synstable Fusion: A Network-Based Algorithm for Estimating Driver Genes in Fusion Structures.Molecules. 2018 Aug 16;23(8):2055. doi: 10.3390/molecules23082055. Molecules. 2018. PMID: 30115851 Free PMC article.
-
Ensemble Methods with Voting Protocols Exhibit Superior Performance for Predicting Cancer Clinical Endpoints and Providing More Complete Coverage of Disease-Related Genes.Int J Genomics. 2018 Jan 10;2018:8124950. doi: 10.1155/2018/8124950. eCollection 2018. Int J Genomics. 2018. PMID: 29546047 Free PMC article.
-
Community Detection in Large-Scale Bipartite Biological Networks.Front Genet. 2021 Apr 21;12:649440. doi: 10.3389/fgene.2021.649440. eCollection 2021. Front Genet. 2021. PMID: 33968132 Free PMC article. Review.
-
Bipartite graphs in systems biology and medicine: a survey of methods and applications.Gigascience. 2018 Apr 1;7(4):1-31. doi: 10.1093/gigascience/giy014. Gigascience. 2018. PMID: 29648623 Free PMC article. Review.
References
-
- van't Veer LJ, Dai H, van de Vijver MJ, et al. Gene expression profiling predicts clinical outcome of breast cancer. Nature. 2002;415(6871):530–536. - PubMed
-
- Zhan F, Hardin J, Kordsmeier B, et al. Global gene expression profiling of multiple myeloma, monoclonal gammopathy of undetermined significance, and normal bone marrow plasma cells. Blood. 2002;99(5):1745–1757. - PubMed
-
- Hess KR, Anderson K, Symmans WF, et al. Pharmacogenomic predictor of sensitivity to preoperative chemotherapy with paclitaxel and fluorouracil, doxorubicin, and cyclophosphamide in breast cancer. Journal of Clinical Oncology. 2006;24(26):4236–4244. - PubMed
-
- Shaughnessy JD, Jr., Zhan F, Burington BE, et al. Avalidated gene expression model of high-risk multiple myeloma is defined by deregulated expression of genes mapping to chromosome 1. Blood. 2007;109(6):2276–2284. - PubMed
Publication types
MeSH terms
Substances
LinkOut - more resources
Full Text Sources
Other Literature Sources