MAVTgsa: an R package for gene set (enrichment) analysis
- PMID: 25101274
- PMCID: PMC4101957
- DOI: 10.1155/2014/346074
MAVTgsa: an R package for gene set (enrichment) analysis
Abstract
Gene set analysis methods aim to determine whether an a priori defined set of genes shows statistically significant difference in expression on either categorical or continuous outcomes. Although many methods for gene set analysis have been proposed, a systematic analysis tool for identification of different types of gene set significance modules has not been developed previously. This work presents an R package, called MAVTgsa, which includes three different methods for integrated gene set enrichment analysis. (1) The one-sided OLS (ordinary least squares) test detects coordinated changes of genes in gene set in one direction, either up- or downregulation. (2) The two-sided MANOVA (multivariate analysis variance) detects changes both up- and downregulation for studying two or more experimental conditions. (3) A random forests-based procedure is to identify gene sets that can accurately predict samples from different experimental conditions or are associated with the continuous phenotypes. MAVTgsa computes the P values and FDR (false discovery rate) q-value for all gene sets in the study. Furthermore, MAVTgsa provides several visualization outputs to support and interpret the enrichment results. This package is available online.
Figures
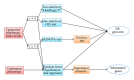
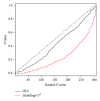
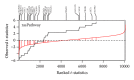
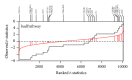
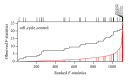
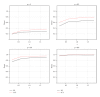
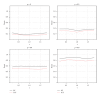
Similar articles
-
Concordant integrative gene set enrichment analysis of multiple large-scale two-sample expression data sets.BMC Genomics. 2014;15 Suppl 1(Suppl 1):S6. doi: 10.1186/1471-2164-15-S1-S6. Epub 2014 Jan 24. BMC Genomics. 2014. PMID: 24564564 Free PMC article.
-
Comparative study of gene set enrichment methods.BMC Bioinformatics. 2009 Sep 2;10:275. doi: 10.1186/1471-2105-10-275. BMC Bioinformatics. 2009. PMID: 19725948 Free PMC article.
-
Extensions to gene set enrichment.Bioinformatics. 2007 Feb 1;23(3):306-13. doi: 10.1093/bioinformatics/btl599. Epub 2006 Nov 24. Bioinformatics. 2007. PMID: 17127676
-
Gene-set analysis and reduction.Brief Bioinform. 2009 Jan;10(1):24-34. doi: 10.1093/bib/bbn042. Epub 2008 Oct 4. Brief Bioinform. 2009. PMID: 18836208 Free PMC article. Review.
-
Stability and aggregation of ranked gene lists.Brief Bioinform. 2009 Sep;10(5):556-68. doi: 10.1093/bib/bbp034. Brief Bioinform. 2009. PMID: 19679825 Review.
Cited by
-
Gene set analysis using sufficient dimension reduction.BMC Bioinformatics. 2016 Feb 6;17:74. doi: 10.1186/s12859-016-0928-6. BMC Bioinformatics. 2016. PMID: 26852017 Free PMC article.
-
mitch: multi-contrast pathway enrichment for multi-omics and single-cell profiling data.BMC Genomics. 2020 Jun 29;21(1):447. doi: 10.1186/s12864-020-06856-9. BMC Genomics. 2020. PMID: 32600408 Free PMC article.
-
Geographically weighted linear combination test for gene-set analysis of a continuous spatial phenotype as applied to intratumor heterogeneity.Front Cell Dev Biol. 2023 Mar 9;11:1065586. doi: 10.3389/fcell.2023.1065586. eCollection 2023. Front Cell Dev Biol. 2023. PMID: 36998245 Free PMC article.
References
-
- Drǎghici S, Khatri P, Martins RP, Ostermeier GC, Krawetz SA. Global functional profiling of gene expression. Genomics. 2003;81(2):98–104. - PubMed
-
- Rivals I, Personnaz L, Taing L, Potier MC. Enrichment or depletion of a GO category within a class of genes: which test? Bioinformatics. 2007;23(4):401–407. - PubMed
-
- Mootha VK, Lindgren CM, Eriksson K, et al. PGC-1α-responsive genes involved in oxidative phosphorylation are coordinately downregulated in human diabetes. Nature Genetics. 2003;34(3):267–273. - PubMed
Publication types
MeSH terms
LinkOut - more resources
Full Text Sources
Other Literature Sources