Homology-based prediction of interactions between proteins using Averaged One-Dependence Estimators
- PMID: 24953126
- PMCID: PMC4229973
- DOI: 10.1186/1471-2105-15-213
Homology-based prediction of interactions between proteins using Averaged One-Dependence Estimators
Abstract
Background: Identification of protein-protein interactions (PPIs) is essential for a better understanding of biological processes, pathways and functions. However, experimental identification of the complete set of PPIs in a cell/organism ("an interactome") is still a difficult task. To circumvent limitations of current high-throughput experimental techniques, it is necessary to develop high-performance computational methods for predicting PPIs.
Results: In this article, we propose a new computational method to predict interaction between a given pair of protein sequences using features derived from known homologous PPIs. The proposed method is capable of predicting interaction between two proteins (of unknown structure) using Averaged One-Dependence Estimators (AODE) and three features calculated for the protein pair: (a) sequence similarities to a known interacting protein pair (FSeq), (b) statistical propensities of domain pairs observed in interacting proteins (FDom) and (c) a sum of edge weights along the shortest path between homologous proteins in a PPI network (FNet). Feature vectors were defined to lie in a half-space of the symmetrical high-dimensional feature space to make them independent of the protein order. The predictability of the method was assessed by a 10-fold cross validation on a recently created human PPI dataset with randomly sampled negative data, and the best model achieved an Area Under the Curve of 0.79 (pAUC0.5% = 0.16). In addition, the AODE trained on all three features (named PSOPIA) showed better prediction performance on a separate independent data set than a recently reported homology-based method.
Conclusions: Our results suggest that FNet, a feature representing proximity in a known PPI network between two proteins that are homologous to a target protein pair, contributes to the prediction of whether the target proteins interact or not. PSOPIA will help identify novel PPIs and estimate complete PPI networks. The method proposed in this article is freely available on the web at http://mizuguchilab.org/PSOPIA.
Figures
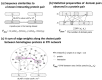
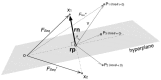
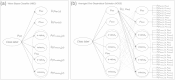
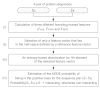
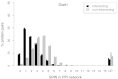
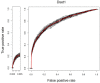
Similar articles
-
Predicting domain-domain interaction based on domain profiles with feature selection and support vector machines.BMC Bioinformatics. 2010 Oct 29;11:537. doi: 10.1186/1471-2105-11-537. BMC Bioinformatics. 2010. PMID: 21034480 Free PMC article.
-
A computational model for predicting protein interactions based on multidomain collaboration.IEEE/ACM Trans Comput Biol Bioinform. 2012 Jul-Aug;9(4):1081-90. doi: 10.1109/TCBB.2012.55. IEEE/ACM Trans Comput Biol Bioinform. 2012. PMID: 22508910
-
RVMAB: Using the Relevance Vector Machine Model Combined with Average Blocks to Predict the Interactions of Proteins from Protein Sequences.Int J Mol Sci. 2016 May 18;17(5):757. doi: 10.3390/ijms17050757. Int J Mol Sci. 2016. PMID: 27213337 Free PMC article.
-
Recent advances in predicting and modeling protein-protein interactions.Trends Biochem Sci. 2023 Jun;48(6):527-538. doi: 10.1016/j.tibs.2023.03.003. Epub 2023 Apr 14. Trends Biochem Sci. 2023. PMID: 37061423 Review.
-
Domain-mediated protein interaction prediction: From genome to network.FEBS Lett. 2012 Aug 14;586(17):2751-63. doi: 10.1016/j.febslet.2012.04.027. Epub 2012 May 3. FEBS Lett. 2012. PMID: 22561014 Review.
Cited by
-
A source of resistance against yellow mosaic disease in soybeans correlates with a novel mutation in a resistance gene.Front Plant Sci. 2023 Nov 24;14:1230559. doi: 10.3389/fpls.2023.1230559. eCollection 2023. Front Plant Sci. 2023. PMID: 38078080 Free PMC article.
-
SpatialPPI: Three-dimensional space protein-protein interaction prediction with AlphaFold Multimer.Comput Struct Biotechnol J. 2024 Mar 15;23:1214-1225. doi: 10.1016/j.csbj.2024.03.009. eCollection 2024 Dec. Comput Struct Biotechnol J. 2024. PMID: 38545599 Free PMC article.
-
The Dual Functions of Andrographolide in the Epstein-Barr Virus-Positive Head-and-Neck Cancer Cells: The Inhibition of Lytic Reactivation of the Epstein-Barr Virus and the Induction of Cell Death.Int J Mol Sci. 2023 Nov 1;24(21):15867. doi: 10.3390/ijms242115867. Int J Mol Sci. 2023. PMID: 37958849 Free PMC article.
-
Machine Learning and Its Applications for Protozoal Pathogens and Protozoal Infectious Diseases.Front Cell Infect Microbiol. 2022 Apr 28;12:882995. doi: 10.3389/fcimb.2022.882995. eCollection 2022. Front Cell Infect Microbiol. 2022. PMID: 35573796 Free PMC article. Review.
-
Analysis of Predicted Host-Parasite Interactomes Reveals Commonalities and Specificities Related to Parasitic Lifestyle and Tissues Tropism.Front Immunol. 2019 Feb 13;10:212. doi: 10.3389/fimmu.2019.00212. eCollection 2019. Front Immunol. 2019. PMID: 30815000 Free PMC article.
References
-
- von Mering C, Krause R, Snel B, Cornell M, Oliver SG, Fields S, Bork P. Comparative assessment of large-scale data sets of protein-protein interactions. Nature. 2002;417(6887):399–403. - PubMed
-
- Han JD, Dupuy D, Bertin N, Cusick ME, Vidal M. Effect of sampling on topology predictions of protein-protein interaction networks. Nat Biotechnol. 2005;23(7):839–844. - PubMed
-
- Bock JR, Gough DA. Predicting protein–protein interactions from primary structure. Bioinformatics. 2001;17(5):455–460. - PubMed
-
- Sprinzak E, Margalit H. Correlated sequence-signatures as markers of protein-protein interaction. J Mol Biol. 2001;311(4):681–692. - PubMed
-
- Gomez SM, Noble WS, Rzhetsky A. Learning to predict protein-protein interactions from protein sequences. Bioinformatics. 2003;19(15):1875–1881. - PubMed
Publication types
MeSH terms
Substances
LinkOut - more resources
Full Text Sources
Other Literature Sources
Molecular Biology Databases